Video joint denoising and demosaicing with recurrent CNNs
WACV(2023)
摘要
Denoising and demosaicing are two critical components of the image/video processing pipeline. While historically these two tasks have mainly been considered separately, current neural network approaches allow to obtain state-of-the-art results by treating them jointly. However, most existing research focuses in single image or burst joint denoising and demosaicing (JDD). Although related to burst JDD, video JDD deserves its own treatment. In this work we present an empirical exploration of different design aspects of video joint denoising and demosaicing using neural networks. We compare recurrent and non-recurrent approaches and explore aspects such as type of propagated information in recurrent networks, motion compensation, video stabilization, and network architecture. We found that recurrent networks with motion compensation achieve best results. Our work should serve as a strong baseline for future research in video JDD.
更多查看译文
关键词
Applications: Smartphones/end user devices,Visualization
AI 理解论文
溯源树
样例
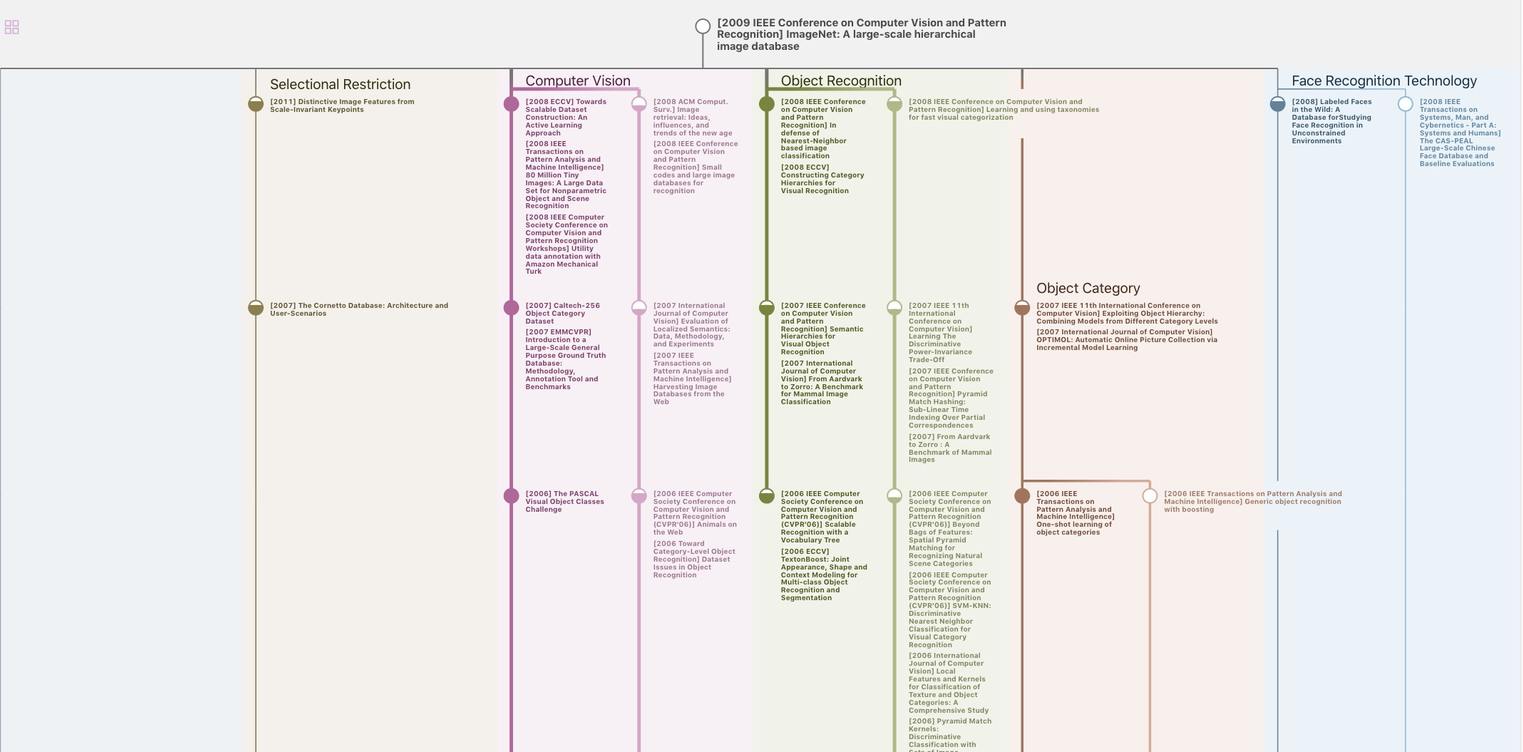
生成溯源树,研究论文发展脉络
Chat Paper
正在生成论文摘要