A new state of charge estimation technique of lithium-ion battery using adaptive extended Kalman filter and artificial neural network.
Trans. Inst. Meas. Control(2023)
摘要
Due to rapid rise in climate change, world is seeing a major technology shift from conventional vehicles to electric vehicles (EVs). Battery's state of charge (SOC) is a critical parameter in EVs whose accuracy affects not only the battery management system but can also influence the driving range estimation for an EV. In this paper, a simulation-based hybrid technique is presented, that combines both adaptive extended Kalman filter (AEKF) and artificial neural network (ANN) for SOC estimation of lithium-ion battery. The proposed hybrid technique is validated using five different EV driving cycles, that is, LA92, US06, urban dynamometer driving schedule (UDDS), highway fuel economy test (HWFET) and a mixed driving cycle at four different temperature values, that is, 0 degrees C, 10 degrees C, 25 degrees C and 40 degrees C. To facilitate the potential EV users, a real-time SOC estimator is also implemented. The real-time SOC estimated through open circuit voltage method and Coulomb counting method is further used for reservation of a charging slot in a nearby charging station. Moreover, to monitor the charging status of a potential EV from a remote location, a cloud-based Internet of things (IoT) platform, that is, ThingSpeak, is used. This results into the reduction of waiting time for an EV user at a charging station. In addition to this, a comparative analysis is carried out between proposed hybrid method and existing methods. It is demonstrated that our hybrid technique achieves lower root mean square error and higher accuracy as compared to existing methods at different operating conditions.
更多查看译文
关键词
State of charge,artificial neural network,AEKF,Coulomb counting,charging stations,Internet of things,EV drive cycles
AI 理解论文
溯源树
样例
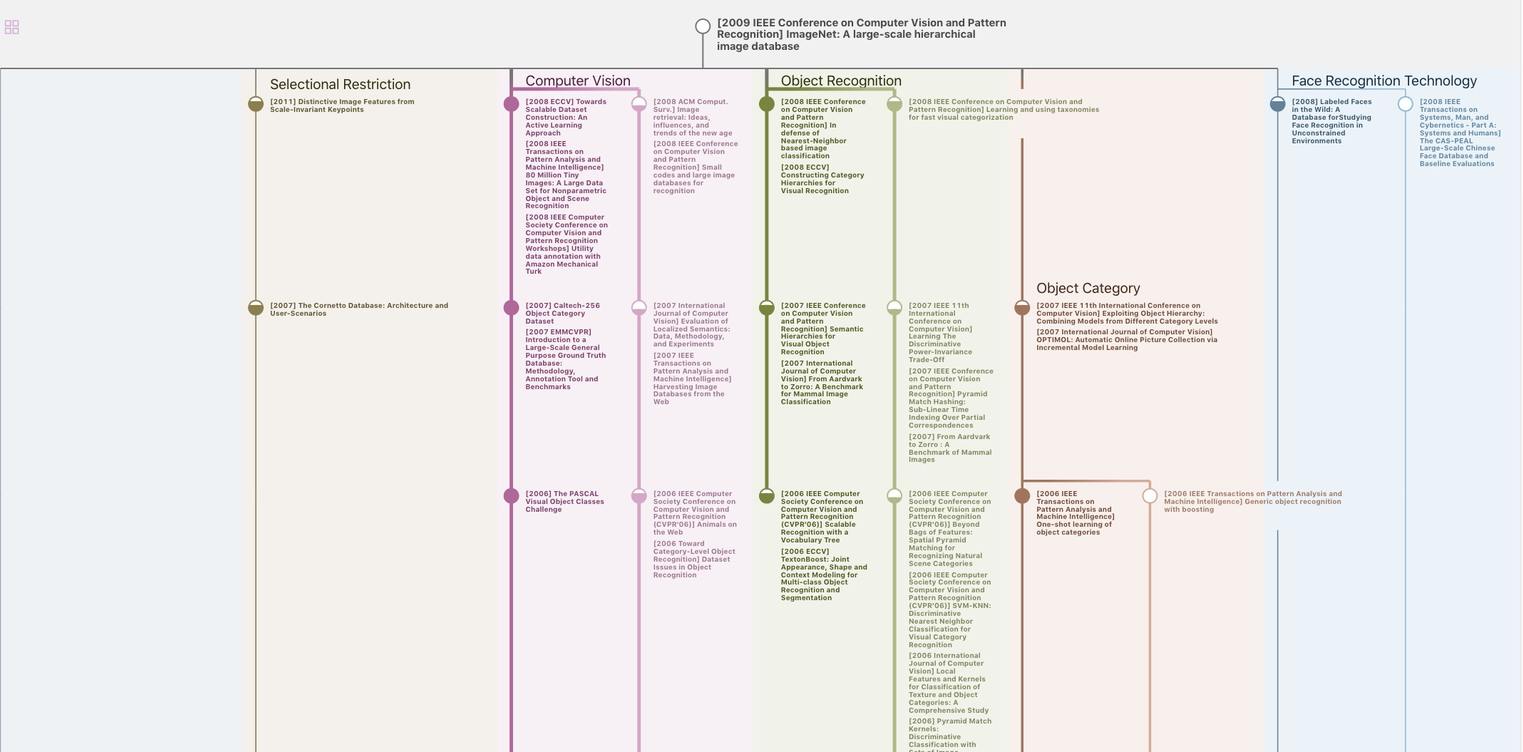
生成溯源树,研究论文发展脉络
Chat Paper
正在生成论文摘要