Unsupervised Domain Transfer with Conditional Invertible Neural Networks
MEDICAL IMAGE COMPUTING AND COMPUTER ASSISTED INTERVENTION, MICCAI 2023, PT I(2023)
摘要
Synthetic medical image generation has evolved as a key technique for neural network training and validation. A core challenge, however, remains in the domain gap between simulations and real data. While deep learning-based domain transfer using Cycle Generative Adversarial Networks and similar architectures has led to substantial progress in the field, there are use cases in which state-of-the-art approaches still fail to generate training images that produce convincing results on relevant downstream tasks. Here, we address this issue with a domain transfer approach based on conditional invertible neural networks (cINNs). As a particular advantage, our method inherently guarantees cycle consistency through its invertible architecture, and network training can efficiently be conducted with maximum likelihood training. To showcase our method's generic applicability, we apply it to two spectral imaging modalities at different scales, namely hyperspectral imaging (pixel-level) and photoacoustic tomography (image-level). According to comprehensive experiments, our method enables the generation of realistic spectral data and outperforms the state of the art on two downstream classification tasks (binary and multi-class). cINN-based domain transfer could thus evolve as an important method for realistic synthetic data generation in the field of spectral imaging and beyond. The code is available at https://github.com/IMSY- DKFZ/UDT- cINN.
更多查看译文
关键词
Domain transfer,invertible neural networks,medical imaging,photoacoustic tomography,hyperspectral imaging,deep learning
AI 理解论文
溯源树
样例
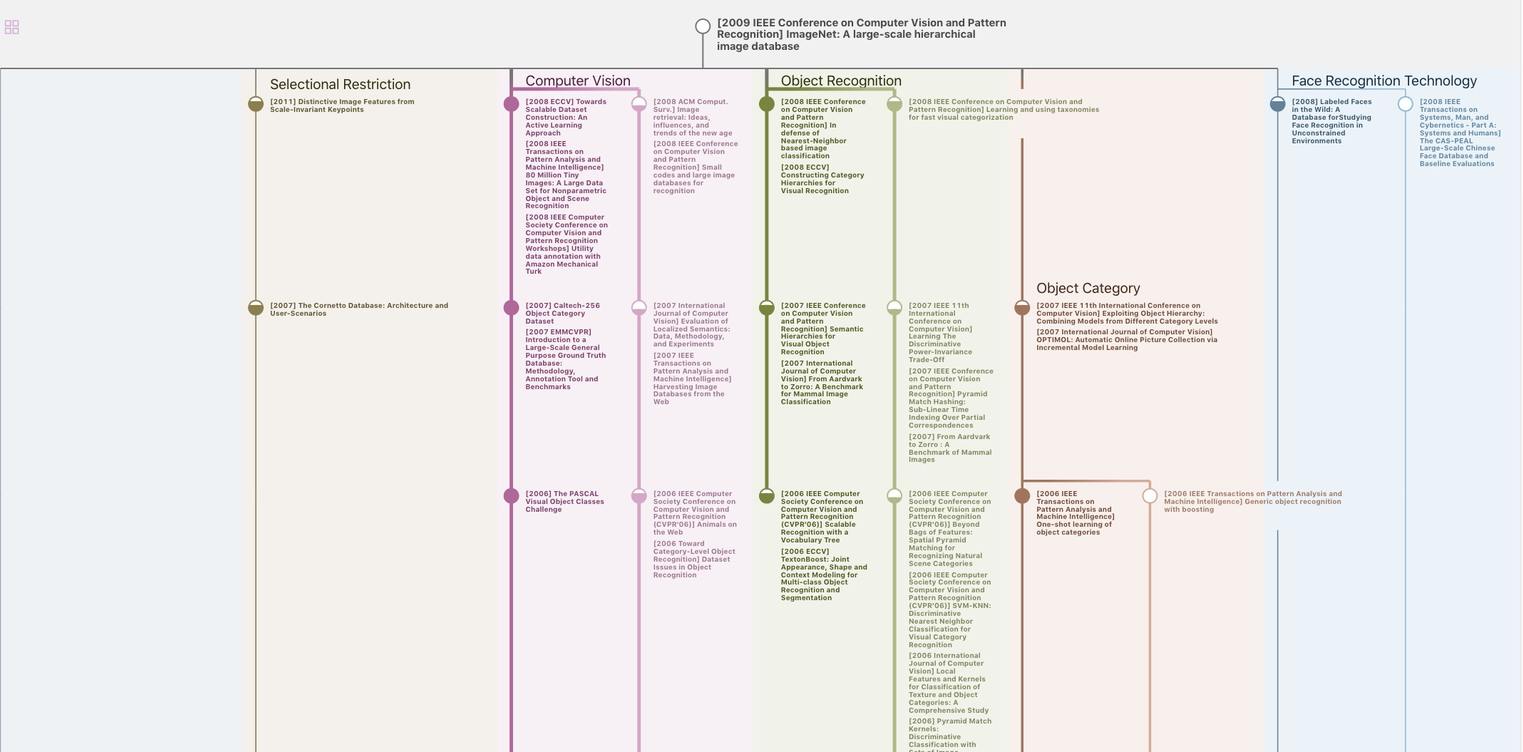
生成溯源树,研究论文发展脉络
Chat Paper
正在生成论文摘要