Development of a machine learning model for prediction of continuous cooling transformation diagrams in welding heat-affected zone
Journal of Materials Science(2023)
摘要
The traditional thermal simulation technique for the measurement of the weld continuous cooling transformation diagrams in synthetic weld heat-affected zone (SH-CCT diagrams) is a complex and time-consuming process. Therefore, it is necessary to develop a model that can rapidly and accurately predict SH-CCT diagrams. In this article, an optimized machine learning (ML) model is developed to predict the SH-CCT diagrams of various types of steel, which consist of the Back Propagation (BP) neural network for the prediction of ferrite transformation starting temperature curves (Fs), and Radial Basis Function (RBF) neural network for the prediction of bainite, pearlite, and martensite transformation starting temperature curves (Bs, Ps, Ms). The accuracy of the present ML model was firstly verified by the excellent agreement between the predicted transformation starting temperature curves and the measured values on the internal dataset (Validation Set I). It was also observed that the predictions obtained through the present model are better than those of the previous models. Moreover, the excellent generalization ability was further verified by the prediction performance on the external dataset (Validation Set II), in which the R 2 values were all above 0.93, and the MAE values were less than 7 K. This successful demonstration indicates that the present model can accurately predict the SH-CCT diagrams of various types of steel, which provides a certain reference for the optimization of steel welding process parameters and the prediction of heat-affected zone (HAZ) microstructure. Graphical Abstract
更多查看译文
关键词
transformation diagrams,machine learning model,machine learning,cooling,heat-affected
AI 理解论文
溯源树
样例
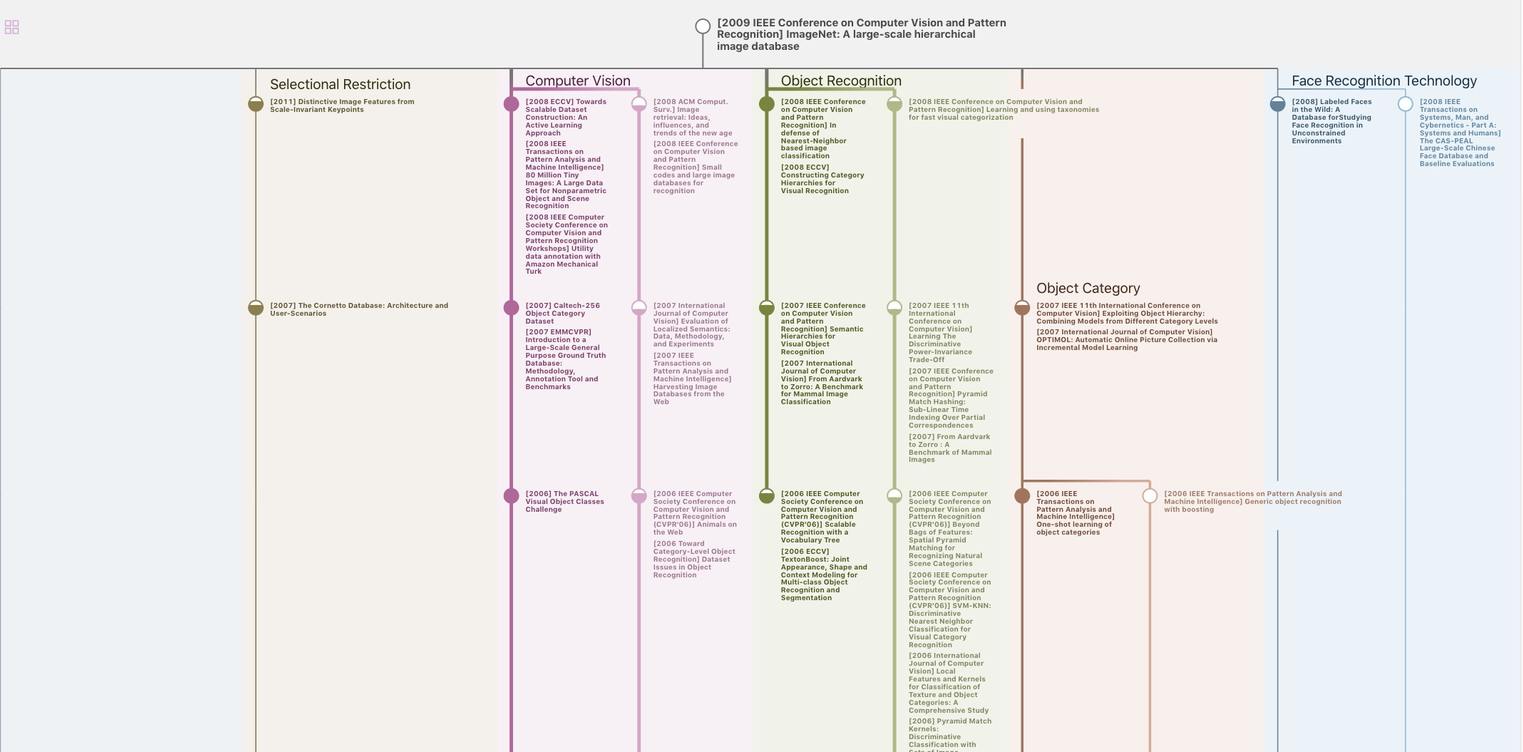
生成溯源树,研究论文发展脉络
Chat Paper
正在生成论文摘要