Addressing bias in online selection with limited budget of comparisons
arxiv(2023)
摘要
Consider a hiring process with candidates coming from different universities. It is easy to order candidates who have the exact same background, yet it can be challenging to compare candidates otherwise. The latter case requires additional assessments, leading to a potentially high total cost for the hiring organization. Given an assigned budget, what is the optimal strategy to select the most qualified candidate? In the absence of additional information, we model the above problem by introducing a new variant of the secretary problem. Completely ordered candidates, belonging to distinct groups, are arriving in a sequential manner. The decision maker has access to the partial order of the candidates within their own group and can request access to the total order of observed candidates by paying some price. Given a bounded budget of comparisons, the goal of the decision-maker is to maximize the probability of selecting the best candidate. We consider a special case of two groups with stochastic i.i.d.\ group membership. We introduce and analyze a particular family of algorithms that we called Dynamic Double Threshold (DDT) family, deriving its asymptotic success probability which, given an optimal choice of parameter converges rapidly to the theoretical upper bound of $1/e$ as the comparison budget growth. We provide an optimal non-asymptotic memory-less algorithm for the above problem and give numerical evidence that it belongs to the DDT family when the number of candidates is high. We compare theoretically and numerically the optimal algorithm with a more naive approach that is directly inspired by the standard single-threshold secretary algorithm. Our analysis reveals several alluring properties of the optimal algorithm. It provides a step towards a fairer online selection process in the presence of unidentifiable biases.
更多查看译文
关键词
online selection,comparisons,bias
AI 理解论文
溯源树
样例
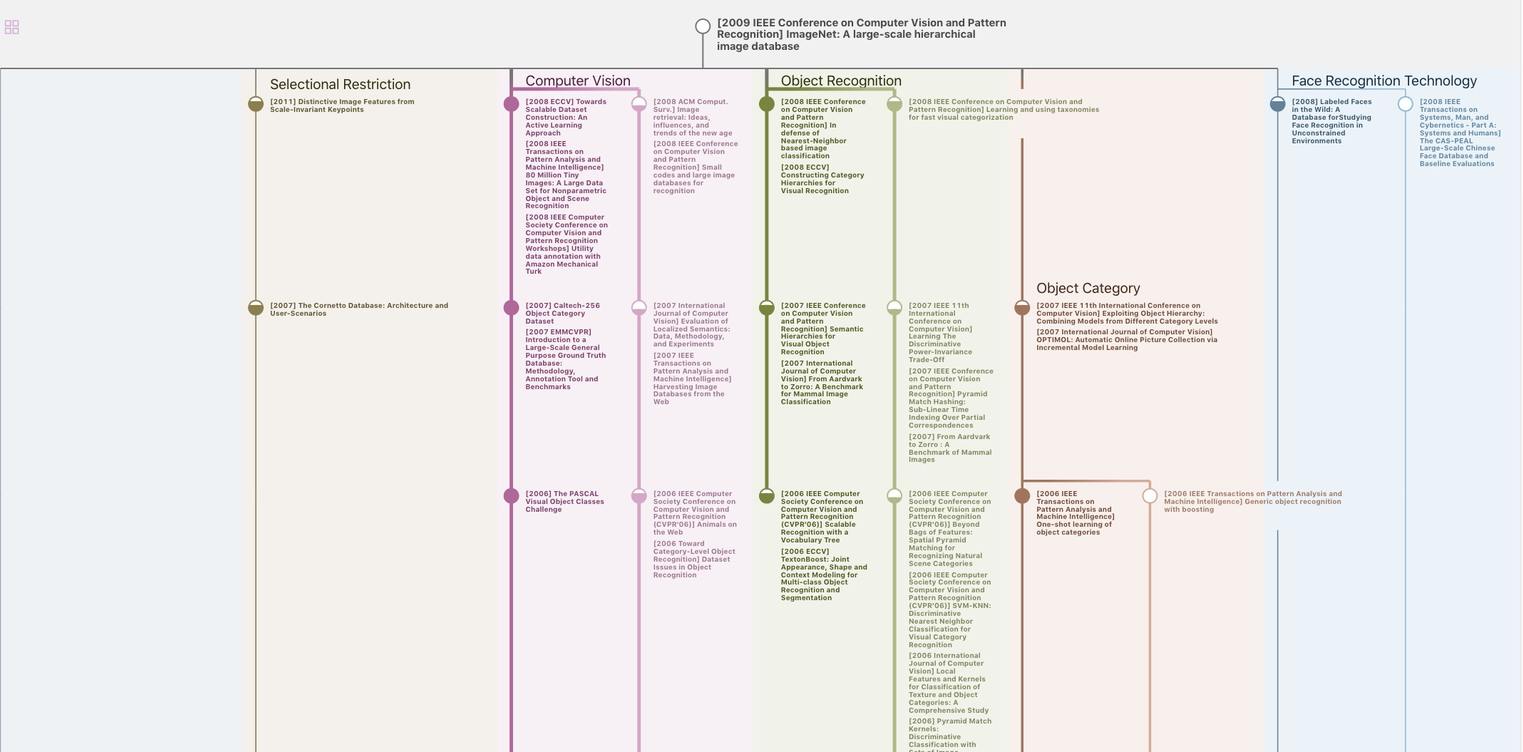
生成溯源树,研究论文发展脉络
Chat Paper
正在生成论文摘要