Physics-Informed Optical Kernel Regression Using Complex-valued Neural Fields
arxiv(2023)
摘要
Lithography is fundamental to integrated circuit fabrication, necessitating large computation overhead. The advancement of machine learning (ML)-based lithography models alleviates the trade-offs between manufacturing process expense and capability. However, all previous methods regard the lithography system as an image-to-image black box mapping, utilizing network parameters to learn by rote mappings from massive mask-to-aerial or mask-to-resist image pairs, resulting in poor generalization capability. In this paper, we propose a new ML-based paradigm disassembling the rigorous lithographic model into non-parametric mask operations and learned optical kernels containing determinant source, pupil, and lithography information. By optimizing complex-valued neural fields to perform optical kernel regression from coordinates, our method can accurately restore lithography system using a small-scale training dataset with fewer parameters, demonstrating superior generalization capability as well. Experiments show that our framework can use 31% of parameters while achieving 69$\times$ smaller mean squared error with 1.3$\times$ higher throughput than the state-of-the-art.
更多查看译文
关键词
complex-valued neural fields,image-to-image black box mapping,integrated circuit fabrication,machine learning-based lithography models,mask-to-resist image pairs,massive mask-to-aerial image pairs,ML-based paradigm,nonparametric mask operations,physics-informed optical kernel regression,rote mappings
AI 理解论文
溯源树
样例
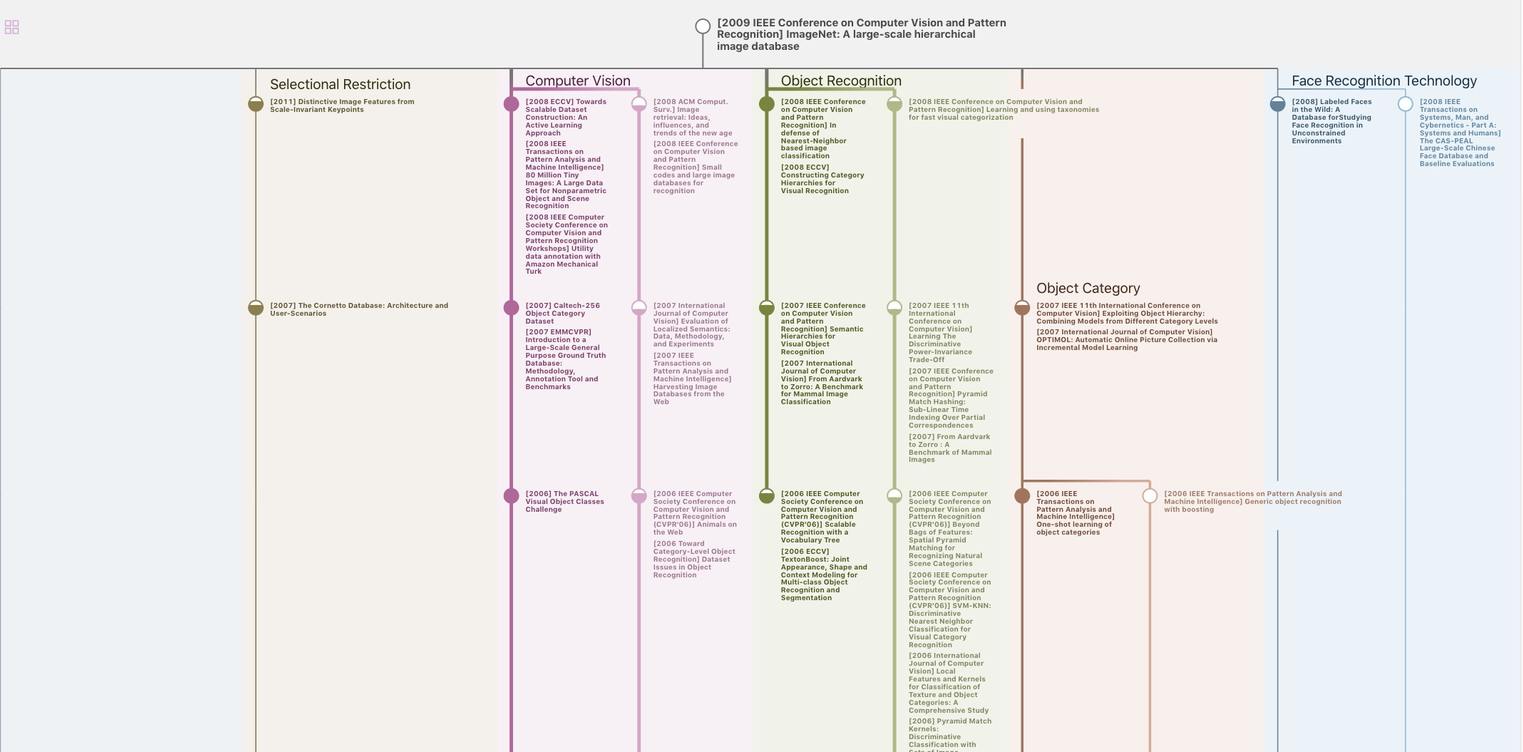
生成溯源树,研究论文发展脉络
Chat Paper
正在生成论文摘要