Deep Masked Graph Matching for Correspondence Identification in Collaborative Perception
2023 IEEE INTERNATIONAL CONFERENCE ON ROBOTICS AND AUTOMATION, ICRA(2023)
摘要
Correspondence identification (CoID) is an essential component for collaborative perception in multi-robot systems, such as connected autonomous vehicles. The goal of CoID is to identify the correspondence of objects observed by multiple robots in their own field of view in order for robots to consistently refer to the same objects. CoID is challenging due to perceptual aliasing, object non-covisibility, and noisy sensing. In this paper, we introduce a novel deep masked graph matching approach to enable CoID and address the challenges. Our approach formulates CoID as a graph matching problem and we design a masked neural network to integrate the multimodal visual, spatial, and GPS information to perform CoID. In addition, we design a new technique to explicitly address object non-covisibility caused by occlusion and the vehicle's limited field of view. We evaluate our approach in a variety of street environments using a high-fidelity simulation that integrates the CARLA and SUMO simulators. The experimental results show that our approach outperforms the previous approaches and achieves state-of-the-art CoID performance in connected autonomous driving applications. Our work is available at: https://github.com/gaopeng5/DMGM.git.
更多查看译文
AI 理解论文
溯源树
样例
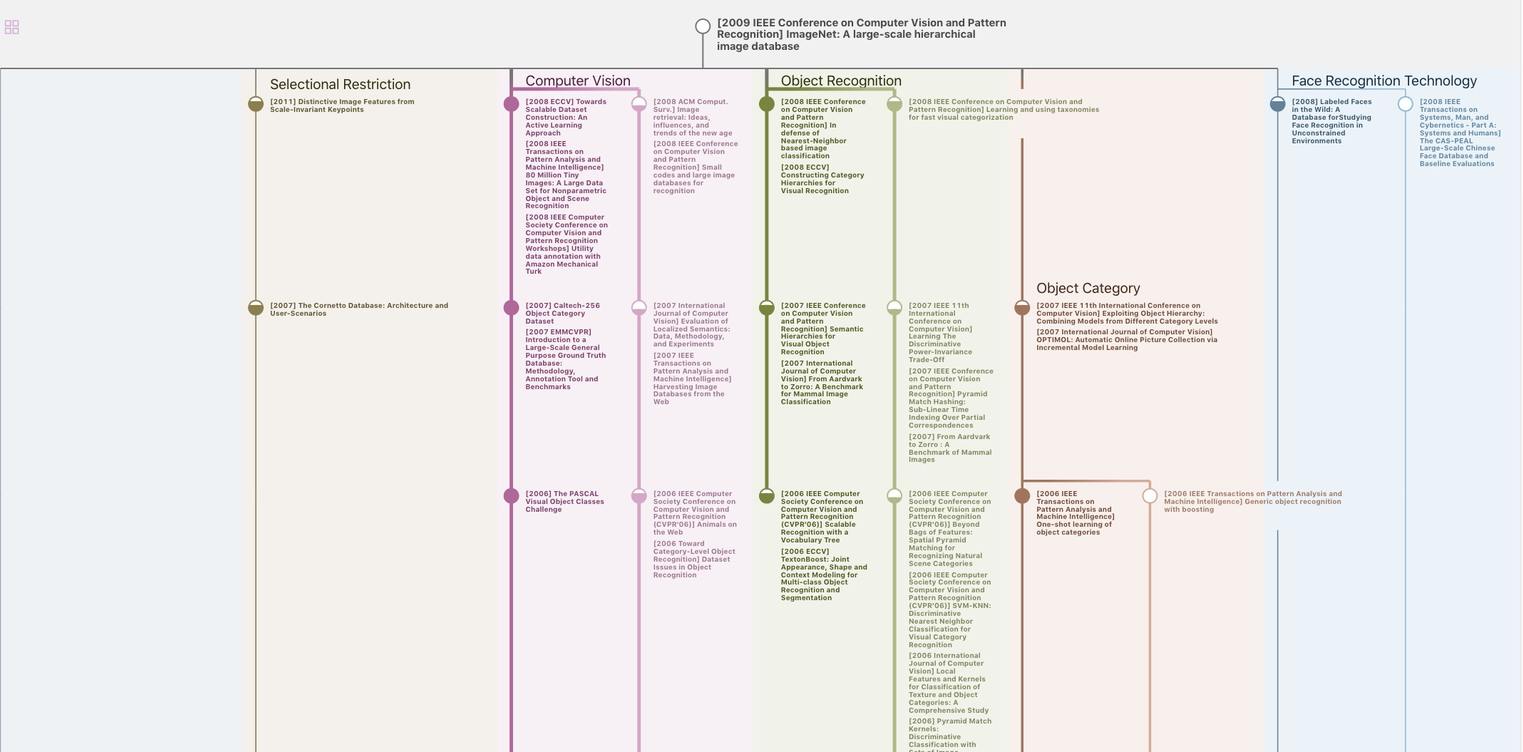
生成溯源树,研究论文发展脉络
Chat Paper
正在生成论文摘要