Viewpoint planning with transition management for active object recognition.
Frontiers in neurorobotics(2023)
摘要
Active object recognition (AOR) provides a paradigm where an agent can capture additional evidence by purposefully changing its viewpoint to improve the quality of recognition. One of the most concerned problems in AOR is viewpoint planning (VP) which refers to developing a policy to determine the next viewpoints of the agent. A research trend is to solve the VP problem with reinforcement learning, namely to use the viewpoint transitions explored by the agent to train the VP policy. However, most research discards the trained transitions, which may lead to an inefficient use of the explored transitions. To solve this challenge, we present a novel VP method with transition management based on reinforcement learning, which can reuse the explored viewpoint transitions. To be specific, a learning framework of the VP policy is first established the deterministic policy gradient theory, which provides an opportunity to reuse the explored transitions. Then, we design a scheme of viewpoint transition management that can store the explored transitions and decide which transitions are used for the policy learning. Finally, within the framework, we develop an algorithm based on twin delayed deep deterministic policy gradient and the designed scheme to train the VP policy. Experiments on the public and challenging dataset GERMS show the effectiveness of our method in comparison with several competing approaches.
更多查看译文
关键词
active object recognition,deterministic policy gradient,reinforcement learning,twin delayed deep deterministic policy gradient,viewpoint planning,viewpoint transition management
AI 理解论文
溯源树
样例
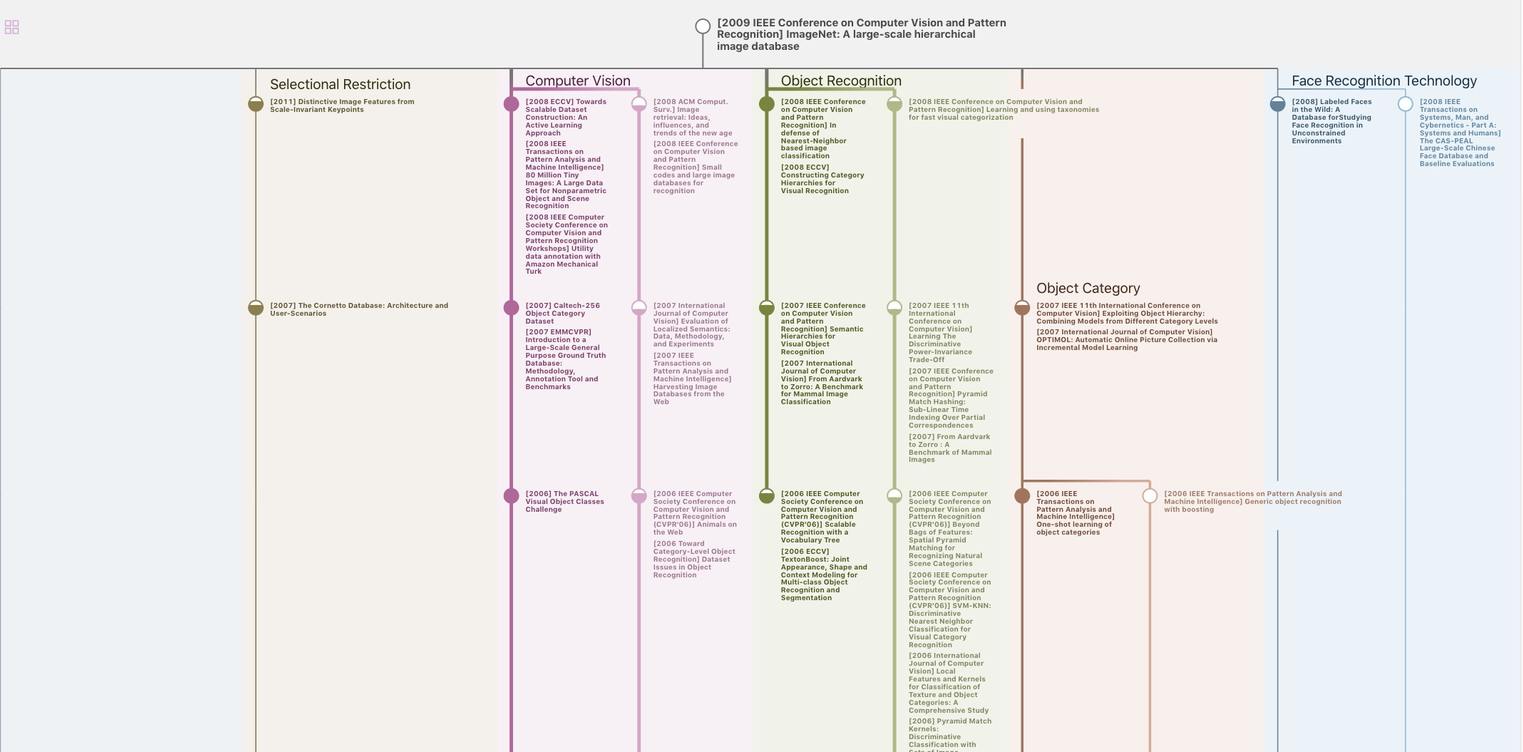
生成溯源树,研究论文发展脉络
Chat Paper
正在生成论文摘要