Semi-supervised domain adaptation for segmentation models on different monitoring settings
Automation in Construction(2023)
摘要
The performance of deep learning models could easily degrade even with slight changes in monitoring settings and environments. Although previous studies have addressed such problems with domain adaptation (DA) methods, this study found that even the state-of-the-art DA methods could not achieve decent adaptation performance in the construction domain. To address the problem, this study presents a novel semi-supervised DA method for semantic segmentation that is built on data augmentation, an unsupervised DA method, and knowledge distillation. Experiments were conducted on the dataset from one construction site (the source domain) and three different construction sites (the target domains). The experimental results demonstrated the effectiveness of the proposed method: the performance is comparable to a model trained with the data in the target domain. The major finding is that DA can be significantly improved when unsupervised DA methods are used with the target domain-specific data augmentation for construction site scenes.
更多查看译文
关键词
Domain adaptation,Semantic segmentation,Target-specific data augmentation,Construction site monitoring
AI 理解论文
溯源树
样例
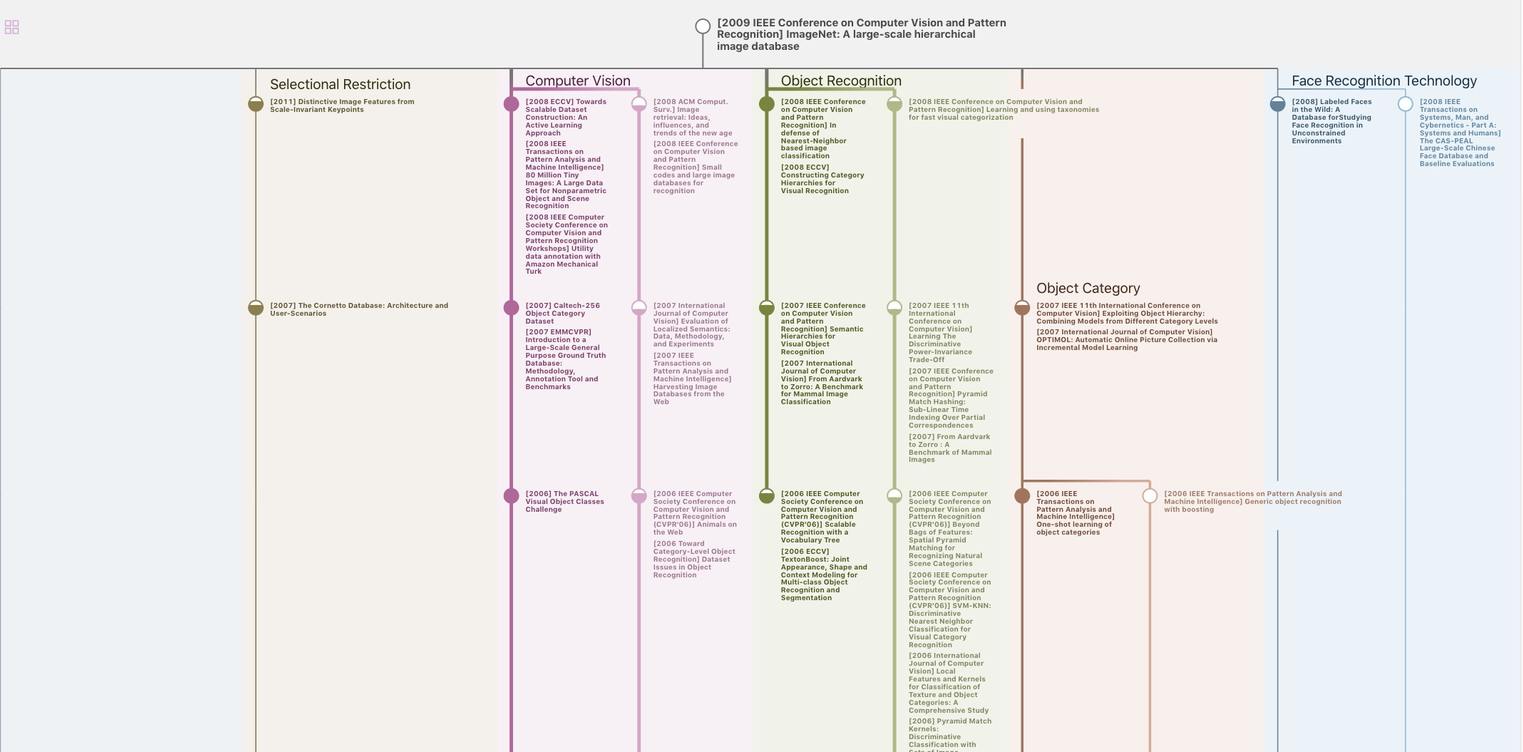
生成溯源树,研究论文发展脉络
Chat Paper
正在生成论文摘要