Calibration method for sensor drifting bias in data center cooling system using Bayesian Inference coupling with Autoencoder
Journal of Building Engineering(2023)
摘要
The optimal control strategy is considered a promising solution to reduce the energy consumption of cooling supply systems in data centers. The strategy is mainly based on sensor measurement data to adjust and control, thereby achieving energy-saving effects. However, the high thermal density characteristics of data centers can cause drifting bias of the sensor, resulting in failure to achieve the desired optimal control strategy. This study proposed a novel method based on Bayesian inference (BI) coupled with autoencoder to model the drifting bias correlation functions and calibrate the sensor drifting bias. Therein, Markov Chain Monte Carlo (MCMC) algorithm was used to generate the equivalent samples without complex integral solution process. Case studies of eight individual/multiple sensors drifting bias scenarios in the computer room air handler (CRAH), were conducted to comprehensively evaluate the calibration performance of the pro-posed method. Moreover, the effect of prior distribution parameters setting on the calibration performance was also discussed. The simulation results show that the proposed method performs well in terms of correction accuracy exceeding 92.60% and 96.34% for individual sensor and multiple sensors drifting bias scenarios, respectively. In addition, the updated strategy of the prior distribution parameter settings plays a crucial role in improving the correction accuracy, espe-cially for multiple sensors drifting bias scenarios. This study filled the knowledge gap of sensor drifting bias correction for data center cooling supply systems and facilitates the application of advanced technologies in data centers.
更多查看译文
关键词
Data center,Computer room air handler,Drifting bias correction,Bayesian Inference,Autoencoder
AI 理解论文
溯源树
样例
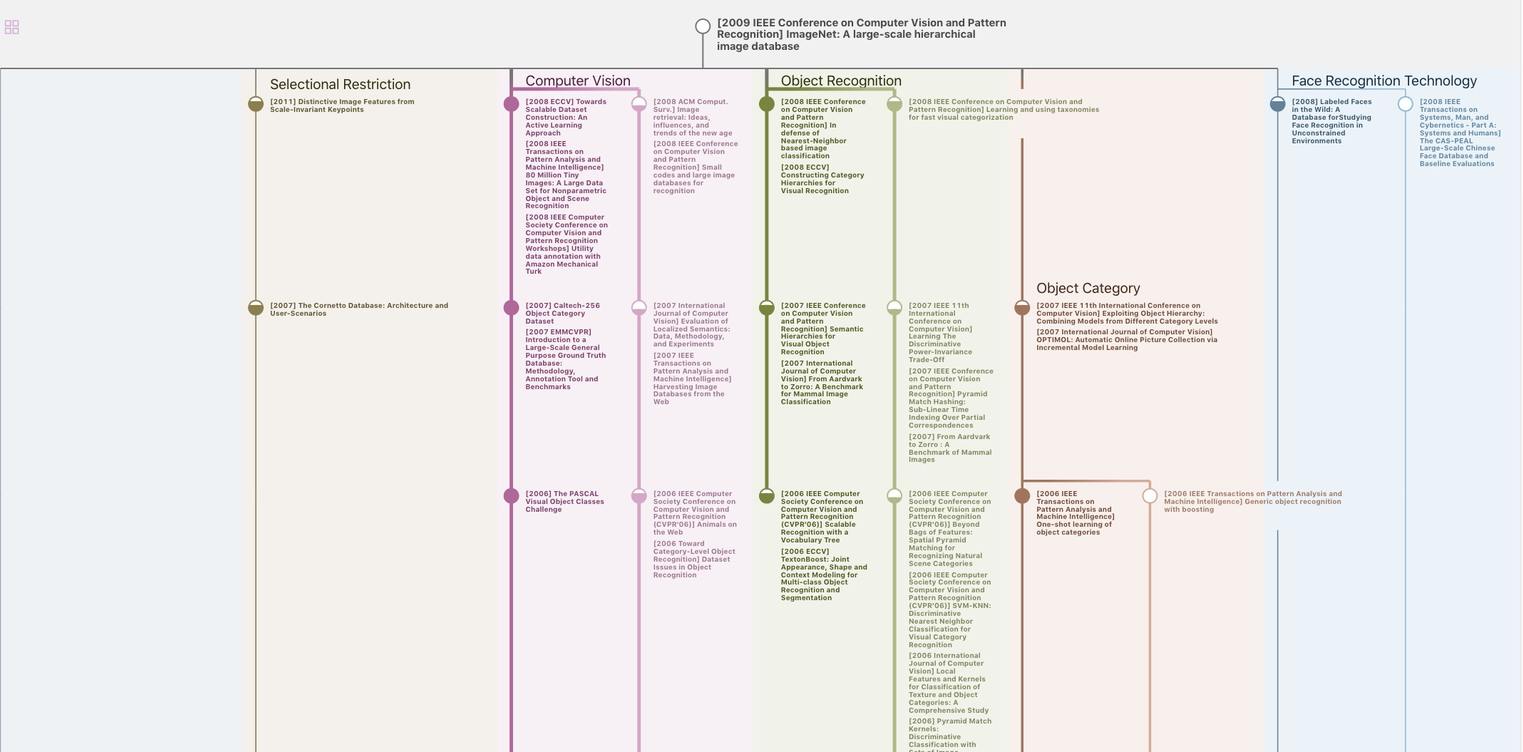
生成溯源树,研究论文发展脉络
Chat Paper
正在生成论文摘要