Quantifying Causes of Arctic Amplification via Deep Learning based Time-series Causal Inference
2023 International Conference on Machine Learning and Applications (ICMLA)(2023)
摘要
The warming of the Arctic, also known as Arctic amplification, is led by several atmospheric and oceanic drivers, however, the details of its underlying thermodynamic causes are still unknown. Inferring the causal effects of atmospheric processes on sea ice melt using fixed treatment effect strategies leads to unrealistic counterfactual estimations. Such models are also prone to bias due to time-varying confoundedness. In order to tackle these challenges, we propose TCINet - time-series causal inference model to infer causation under continuous treatment using recurrent neural networks. Through experiments on synthetic and observational data, we show how our research can substantially improve the ability to quantify the leading causes of Arctic sea ice melt.
更多查看译文
关键词
Causal Inference,Deep Learning,LSTM,Arctic Amplification
AI 理解论文
溯源树
样例
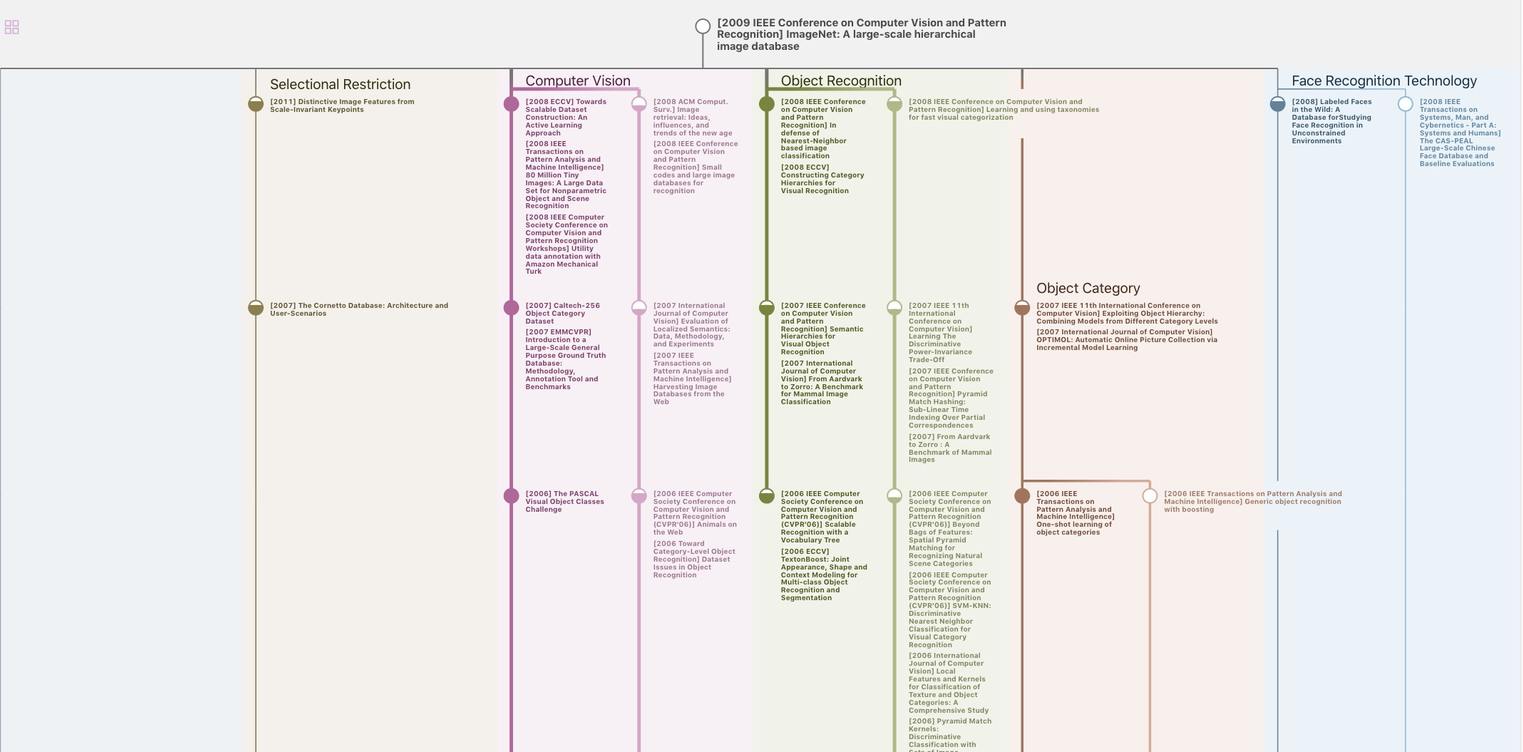
生成溯源树,研究论文发展脉络
Chat Paper
正在生成论文摘要