AUTODIAL: Efficient Asynchronous Task-Oriented Dialogue Model
arxiv(2023)
摘要
As large dialogue models become commonplace in practice, the problems surrounding high compute requirements for training, inference and larger memory footprint still persists. In this work, we present AUTODIAL, a multi-task dialogue model that addresses the challenges of deploying dialogue model. AUTODIAL utilizes parallel decoders to perform tasks such as dialogue act prediction, domain prediction, intent prediction, and dialogue state tracking. Using classification decoders over generative decoders allows AUTODIAL to significantly reduce memory footprint and achieve faster inference times compared to existing generative approach namely SimpleTOD. We demonstrate that AUTODIAL provides 3-6x speedups during inference while having 11x fewer parameters on three dialogue tasks compared to SimpleTOD. Our results show that extending current dialogue models to have parallel decoders can be a viable alternative for deploying them in resource-constrained environments.
更多查看译文
关键词
dialogue model,task-oriented
AI 理解论文
溯源树
样例
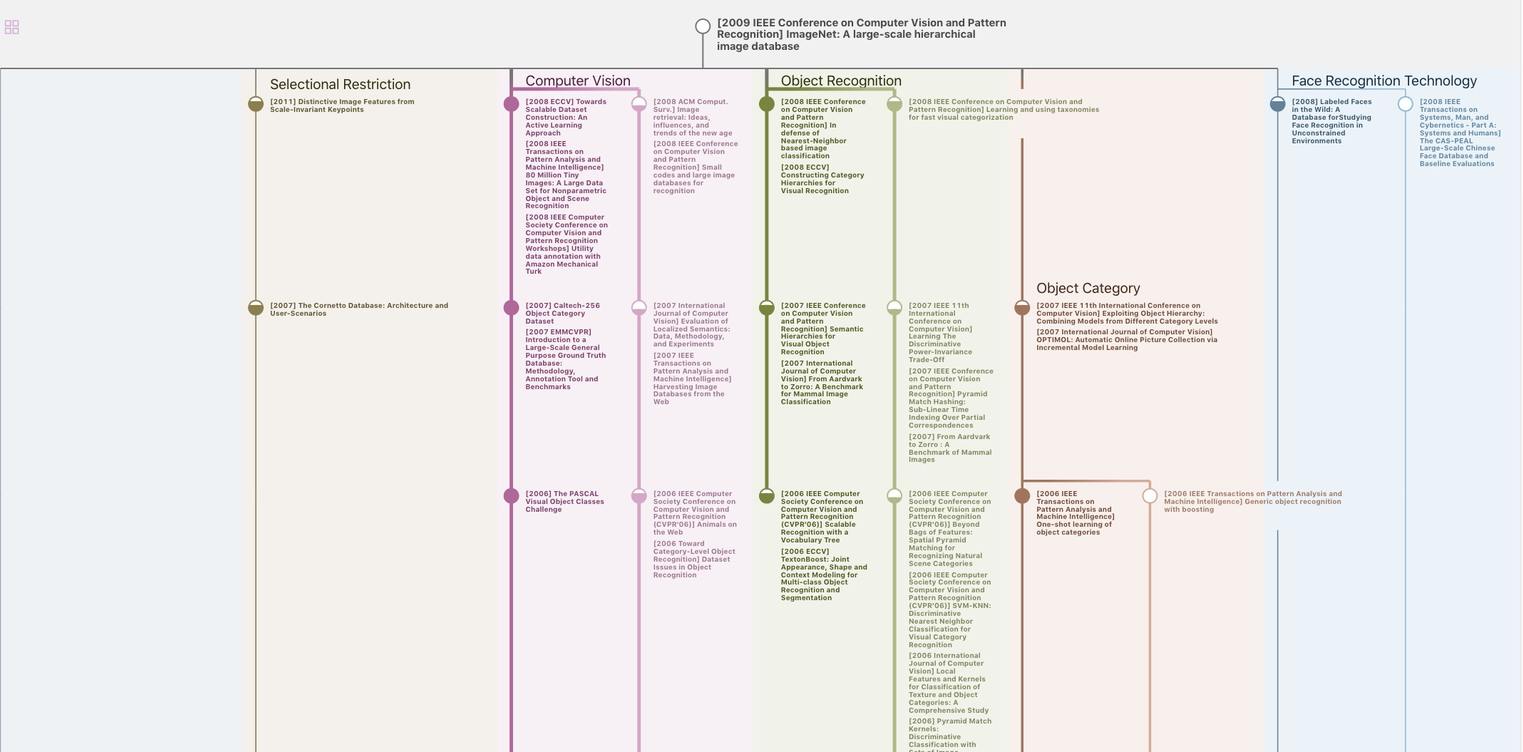
生成溯源树,研究论文发展脉络
Chat Paper
正在生成论文摘要