Adaptive Supervised PatchNCE Loss for Learning H&E-to-IHC Stain Translation with Inconsistent Groundtruth Image Pairs
MEDICAL IMAGE COMPUTING AND COMPUTER ASSISTED INTERVENTION, MICCAI 2023, PT VI(2023)
摘要
Immunohistochemical (IHC) staining highlights the molecular information critical to diagnostics in tissue samples. However, compared to H&E staining, IHC staining can be much more expensive in terms of both labor and the laboratory equipment required. This motivates recent research that demonstrates that the correlations between the morphological information present in the H&E-stained slides and the molecular information in the IHC-stained slides can be used for H&E-to-IHC stain translation. However, due to a lack of pixel-perfect H&E-IHC groundtruth pairs, most existing methods have resorted to relying on expert annotations. To remedy this situation, we present a new loss function, Adaptive Supervised PatchNCE (ASP), to directly deal with the input to target inconsistencies in a proposed H&E-to-IHC image-to-image translation framework. The ASP loss is built upon a patch-based contrastive learning criterion, named Supervised PatchNCE (SP), and augments it further with weight scheduling to mitigate the negative impact of noisy supervision. Lastly, we introduce the Multi-IHC Stain Translation (MIST) dataset, which contains aligned H&E-IHC patches for 4 different IHC stains critical to breast cancer diagnosis. In our experiment, we demonstrate that our proposed method outperforms existing image-to-image translation methods for stain translation to multiple IHC stains. All of our code and datasets are available at https://github.com/lifangda01/AdaptiveSupervisedPatchNCE.
更多查看译文
关键词
Generative Adversarial Network,Contrastive Learning,H&E-to-IHC Stain Translation
AI 理解论文
溯源树
样例
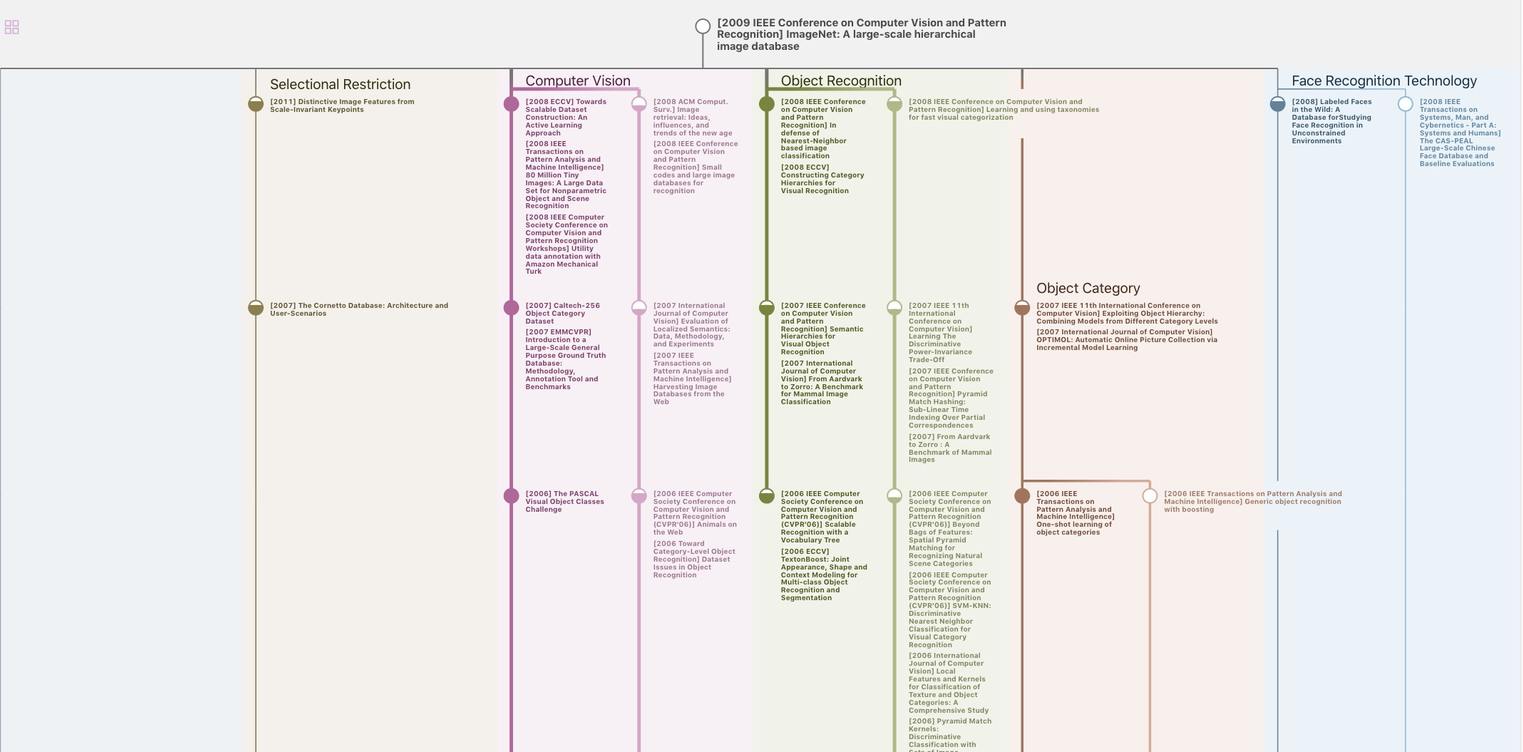
生成溯源树,研究论文发展脉络
Chat Paper
正在生成论文摘要