Kernel-based identification using Lebesgue-sampled data
Automatica(2024)
摘要
Sampling in control applications is increasingly done non-equidistantly in time. This includes applications in motion control, networked control, resource-aware control, and event-based control. Some of these applications, like the ones where displacement is tracked using incremental encoders, are driven by signals that are only measured when their values cross fixed thresholds in the amplitude domain. This paper introduces a non-parametric estimator of the impulse response and transfer function of continuous-time systems based on such amplitude-equidistant sampling strategy, known as Lebesgue sampling. To this end, kernel methods are developed to formulate an algorithm that adequately takes into account the bounded output uncertainty between the event timestamps, which ultimately leads to more accurate models and more efficient output sampling compared to the equidistantly-sampled kernel-based approach. The efficacy of our proposed method is demonstrated through a mass–spring damper example with encoder measurements and extensive Monte Carlo simulation studies on system benchmarks.
更多查看译文
关键词
System identification,Event-based sampling,Kernel-based methods,Regularization,Impulse response estimation
AI 理解论文
溯源树
样例
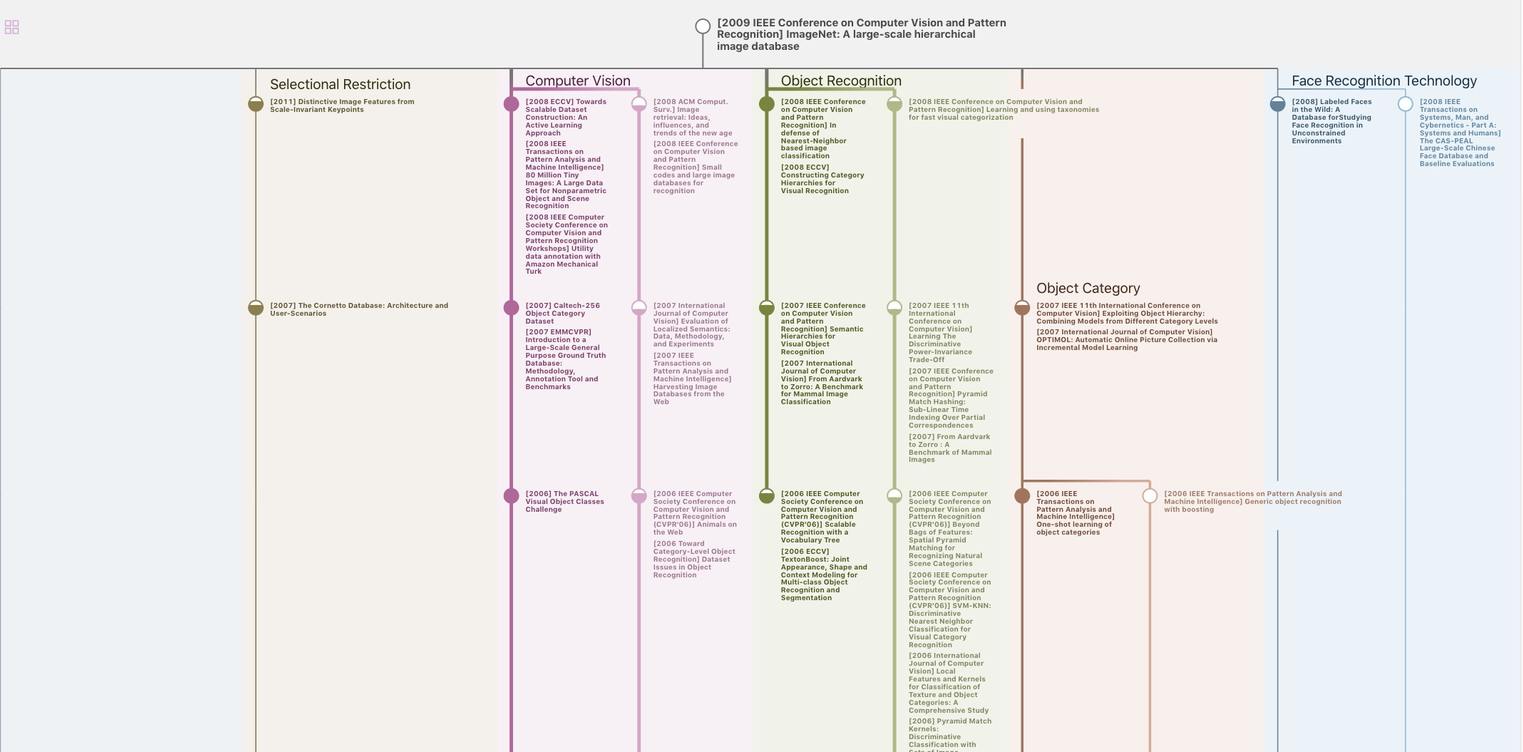
生成溯源树,研究论文发展脉络
Chat Paper
正在生成论文摘要