Gaussian Max-Value Entropy Search for Multi-Agent Bayesian Optimization
2023 IEEE/RSJ INTERNATIONAL CONFERENCE ON INTELLIGENT ROBOTS AND SYSTEMS (IROS)(2023)
摘要
We study the multi-agent Bayesian optimization (BO) problem, where multiple agents maximize a black-box function via iterative queries. We focus on Entropy Search (ES), a sample-efficient BO algorithm that selects queries to maximize the mutual information about the maximum of the black-box function. One of the main challenges of ES is that calculating the mutual information requires computationally-costly approximation techniques. For multi-agent BO problems, the computational cost of ES is exponential in the number of agents. To address this challenge, we propose the Gaussian Max-value Entropy Search, a multi-agent BO algorithm with favorable sample and computational efficiency. The key to our idea is to use a normal distribution to approximate the function maximum and calculate its mutual information accordingly. The resulting approximation allows queries to be cast as the solution of a closed-form optimization problem which, in turn, can be solved via a modified gradient ascent algorithm and scaled to a large number of agents. We demonstrate the effectiveness of Gaussian max-value Entropy Search through numerical experiments on standard test functions and realrobot experiments on the source seeking problem. Results show that the proposed algorithm outperforms the multi-agent BO baselines in the numerical experiments and can stably seek the source with a limited number of noisy observations on real robots.
更多查看译文
关键词
optimization,entropy,max-value,multi-agent
AI 理解论文
溯源树
样例
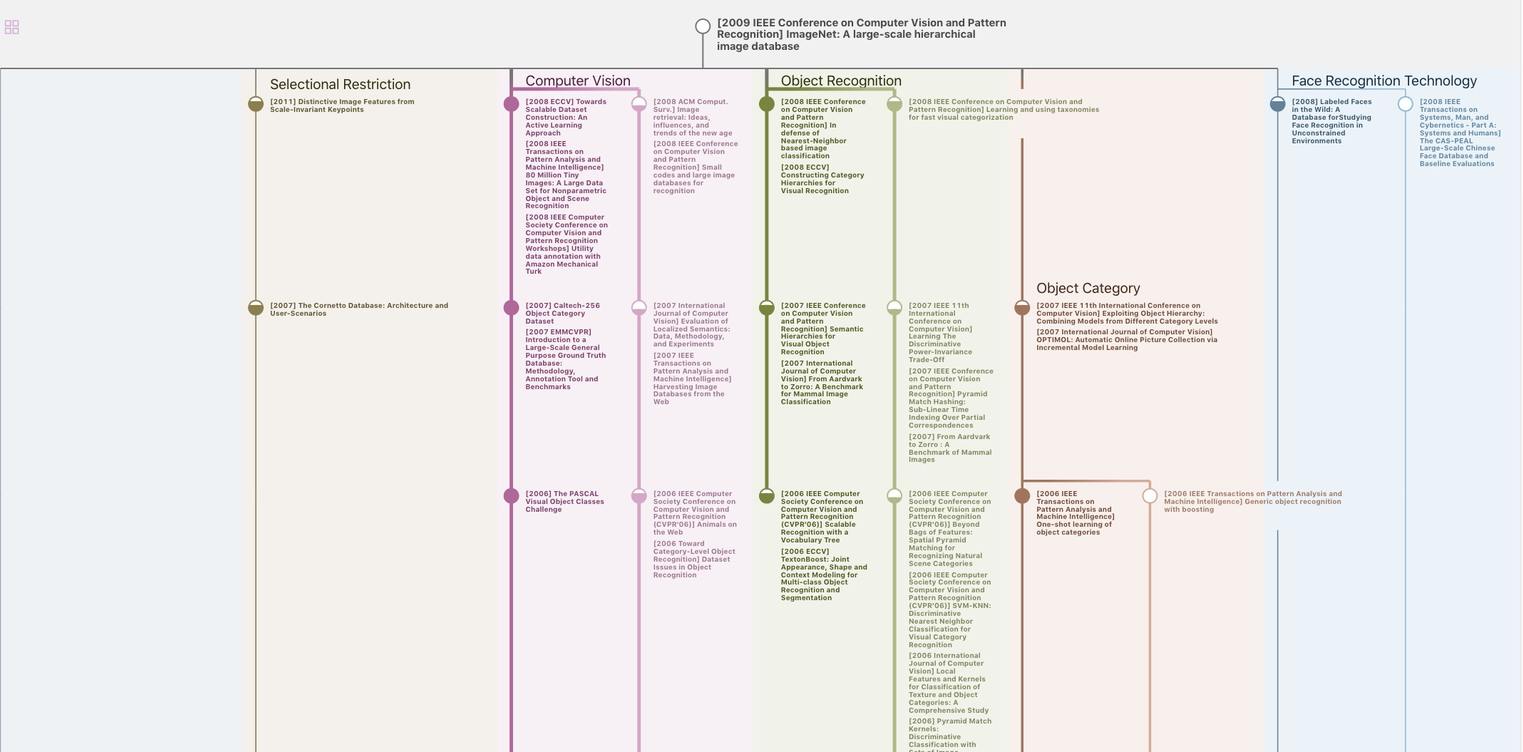
生成溯源树,研究论文发展脉络
Chat Paper
正在生成论文摘要