A novel back propagation neural network-dual extended Kalman filter method for state-of-charge and state-of-health co-estimation of lithium-ion batteries based on limited memory least square algorithm
Journal of Energy Storage(2023)
摘要
State-of-charge (SOC) and state-of-health (SOH) play a key role in the safety and reliability of batteries. To improve the real-time estimation accuracy of battery state, a novel back propagation neural network-dual extended Kalman filter method for SOC and SOH co-estimation of lithium-ion batteries based on limited memory recursive least square algorithm is proposed by establishing a second-order equivalent circuit model. Aiming at the problem of poor anti-interference ability and easy data saturation of recursive least square algorithm, a limited memory recursive least square algorithm that removes the old data and uses new data with only the restricted length is designed to improve the accuracy of on-line parameter identification. Considering the coupling effect between SOC and SOH, the dual extended Kalman filter is used to achieve the synergistic estimation to obtain better tracking accuracy. To compensate for the model error of the extended Kalman filter, a back propagation neural network is introduced for correction to further improve the SOC and SOH estimation precision. When the initial SOC value is set to 1 and the initial SOH value is 0.9809, under hybrid pulse power characterization working condition, the mean absolute error (MAE) and root-mean-square error (RMSE) of state of charge and state of health are 0.13 %, 0.15 % and 0.11 %, 0.12 %, respectively. The corresponding results are 0.24 %, 0.26 % and 0.096 %, 0.11 % under Beijing bus dynamic stress test (BBDST) working condition, respectively. When the initial SOC value is set to 0.8 and the initial SOH value is 0.9788, under BBDST working condition, the MAE and RMSE of SOC prediction are around 1 %, and the MAE and RMSE of SOH estimation are within 0.4 %. The method proposed in this study has high accuracy and robustness, which not only lays a theoretical foundation for battery condition monitoring but also provides a guarantee for the safe application of lithium batteries.
更多查看译文
关键词
Lithium-ion battery,Limited memory recursive least square,BP neural network,Dual extended Kalman filter,State-of-charge,State-of-health
AI 理解论文
溯源树
样例
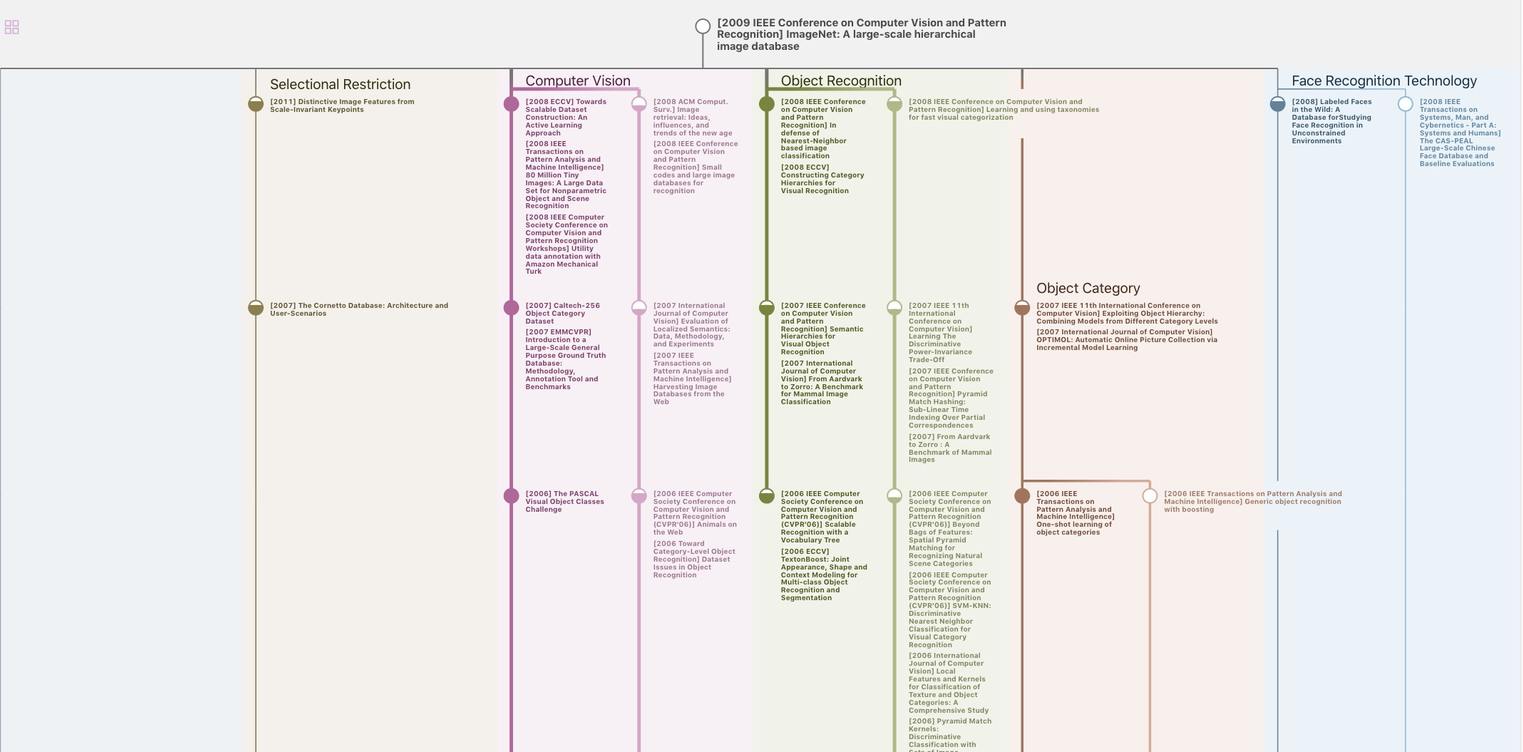
生成溯源树,研究论文发展脉络
Chat Paper
正在生成论文摘要