Schema dependency-enhanced curriculum pre-training for table semantic parsing
Knowledge-Based Systems(2023)
摘要
Large pre-trained models exhibit improved table-semantic-parsing performances by leveraging large-scale corpora to enhance the representation learning ability of semantic parsers. However, existing table pre-training methods do not sufficiently consider the explicit interactions among natural language (NL) questions, SQL queries, and the corresponding database schemas, which are essential for table semantic parsing. To overcome the aforementioned limitation, this study designs a novel schema dependency prediction (SDP) objective to incorporate SQL-aware schema linking information into table pre-training. Specifically, SDP aims to predict the schema dependency connecting the NL question, table schema, and triggered SQL operations. We further propose a schema-aware curriculum learning approach (SAC) to mitigate the impact of noise present in the pre-training data and train the table pre-training model in an easy-to-hard manner. We evaluate the effectiveness of our pre-training framework by fine-tuning it on three downstream benchmarks (Spider, Spider-DK, and SQUALL). Experimental results demonstrate that the proposed pre-training method outperforms existing methods considered for comparison.
更多查看译文
关键词
Table semantic parsing,Table pre-training,Schema dependency,Curriculum learning
AI 理解论文
溯源树
样例
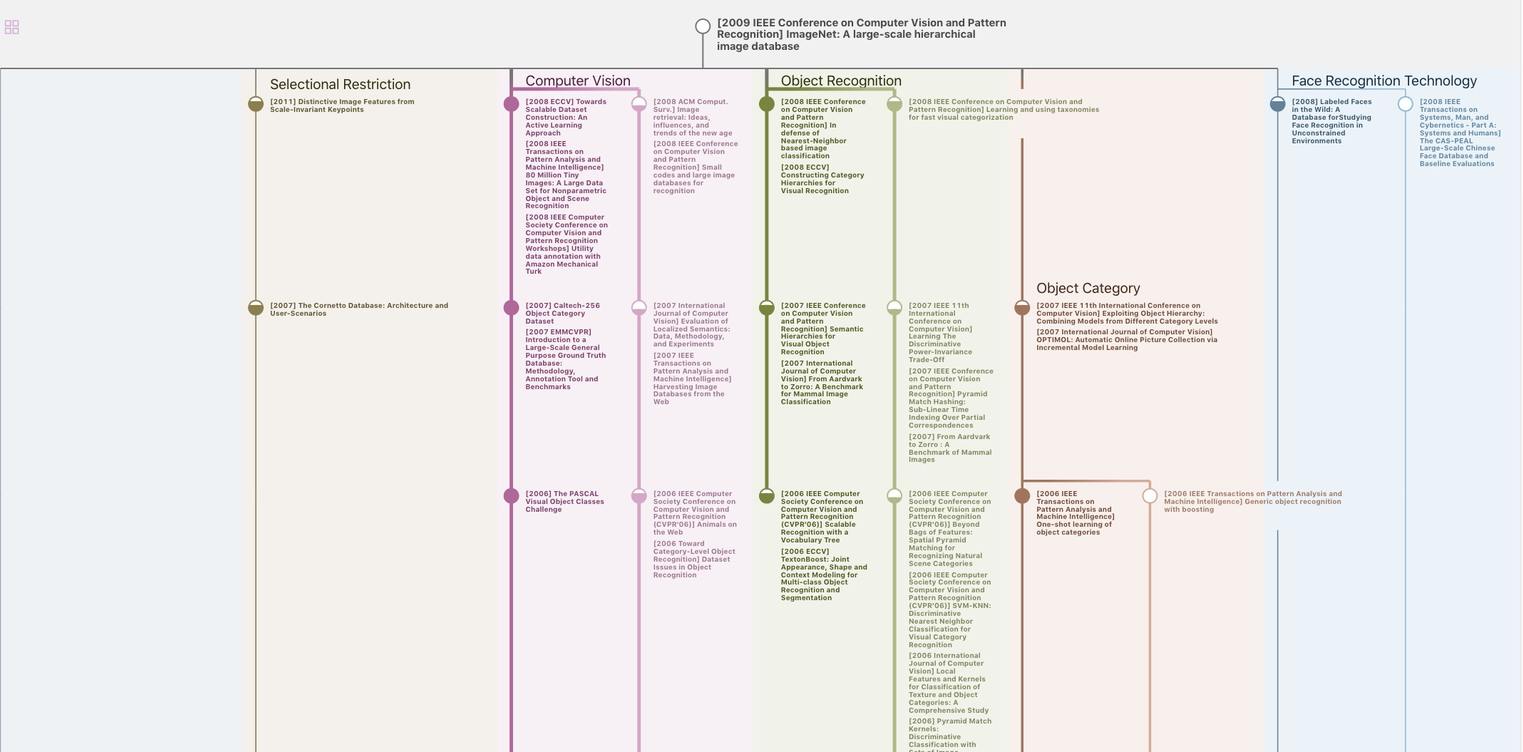
生成溯源树,研究论文发展脉络
Chat Paper
正在生成论文摘要