Improving the Classification Accuracy in Label-Free Flow Cytometry Using Event-Based Vision and Simple Logistic Regression
IEEE Journal of Selected Topics in Quantum Electronics(2023)
摘要
Event-based cameras are novel bio-inspired vision sensors that do not follow the mechanism of traditional frame-based cameras. In the field of data acquisition, the replacement of CMOS cameras with event-based cameras has proved to enhance the accuracy of machine learning methods in situations where critical lighting conditions and rapid dynamics are paramount. In this paper, we investigate for the first time the use of extreme learning machines on data coming from event-based cameras in the context of flow cytometry. Except for the image sensor, the experimental setup is similar to a setup we used in (Lugnan et al., 2020) where we showed that a simple linear classifier can achieve around 10% error rate on background-subtracted cell frames. Here, we show that the the error rate of this simple imaging flow cytometer could be decreased to less than 2% just by making use of the capabilities of an event camera. Moreover, additional benefits like more sensitivity and efficient memory usage are gained. Finally, we suggest further possible improvements to the experimental setup used to record events from flowing micro-particles allowing for more accurate and stable cellx sorting.
更多查看译文
关键词
Event-based camera,extreme learning machine,flow cytometry,image classification
AI 理解论文
溯源树
样例
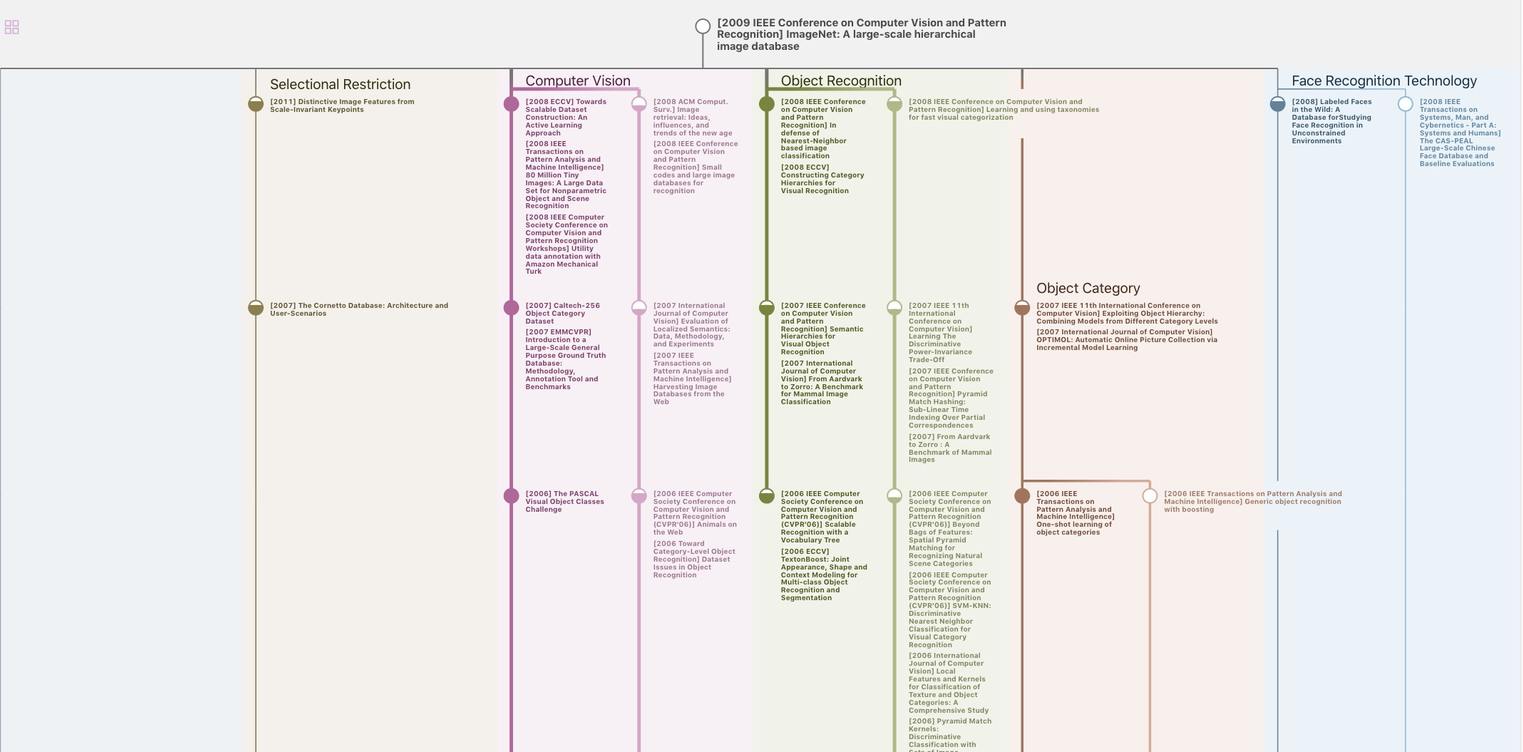
生成溯源树,研究论文发展脉络
Chat Paper
正在生成论文摘要