Hyper-sausage coverage function neuron model and learning algorithm for image classification
Pattern Recognition(2023)
摘要
Recently, deep neural networks (DNNs) promote mainly by network architectures and loss functions; however, the development of neuron models has been quite limited. In this study, inspired by the mechanism of human cognition, a hyper-sausage coverage function (HSCF) neuron model possessing a high flexible plasticity. Then, a novel cross-entropy and volume-coverage (CE_VC) loss is defined, which compresses the volume of the hyper-sausage to the hilt, and helps alleviate confusion among different classes, thus ensuring the intra-class compactness of the samples. Finally, a divisive iteration method is introduced, which considers each neuron model as a weak classifier, and iteratively increases the number of weak classifiers. Thus, the optimal number of the HSCF neuron is adaptively determined and an endto-end learning framework is constructed. In particular, to improve the classification performance, the HSCF neuron can be applied to classical DNNs. Comprehensive experiments on eight datasets in several domains demonstrate the effectiveness of the proposed method. The proposed method exhibits the feasibility of boosting DNNs with neuron plasticity and provides a novel perspective for further developments in DNNs. The source code is available at https://github.com/Tough2011/HSCFNet.git .(c) 2022 The Author(s). Published by Elsevier Ltd. This is an open access article under the CC BY license ( http://creativecommons.org/licenses/by/4.0/ )
更多查看译文
关键词
Pattern recognition,Deep neural networks,Neuron model,Brain -inspired,Computer vision
AI 理解论文
溯源树
样例
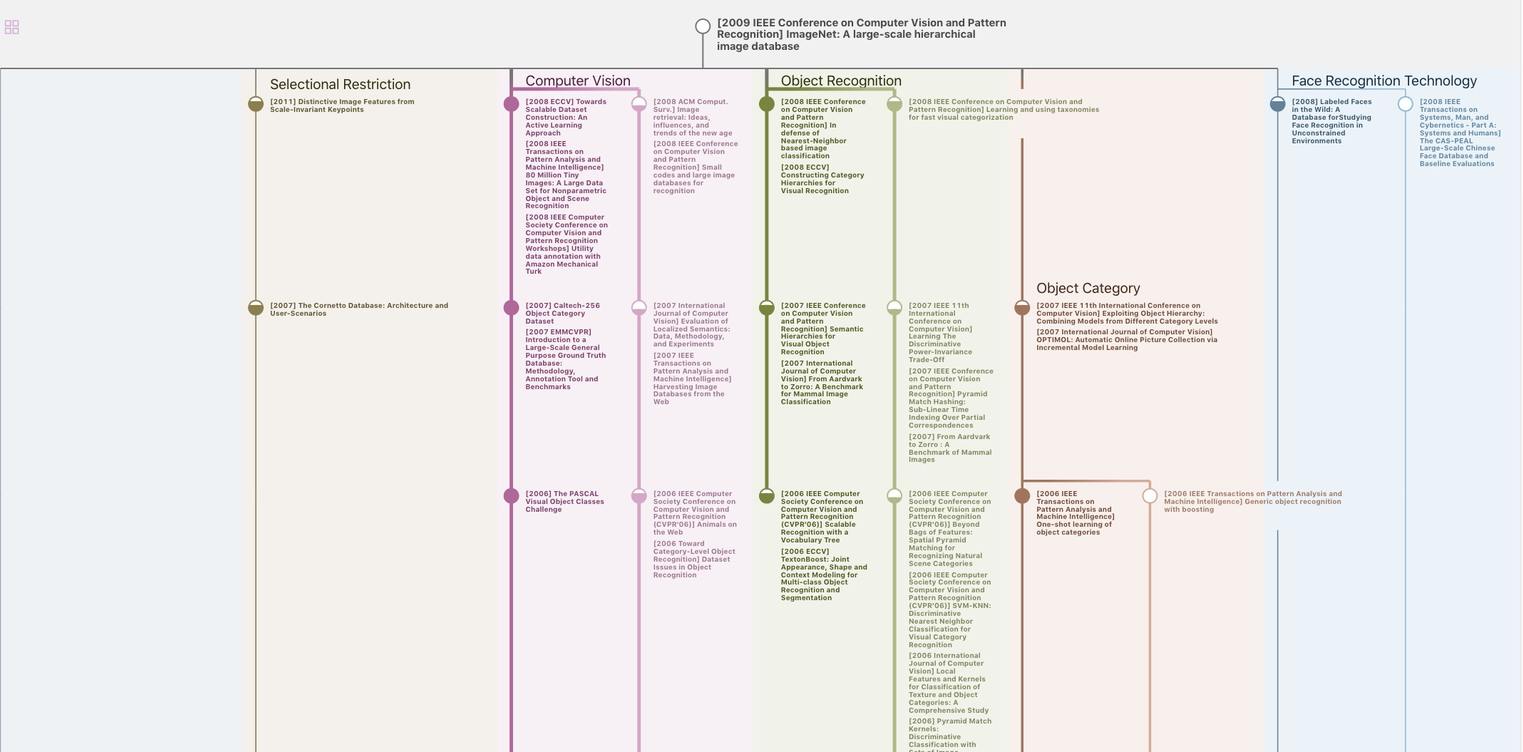
生成溯源树,研究论文发展脉络
Chat Paper
正在生成论文摘要