Detecting and grouping keypoints for multi-person pose estimation using instance-aware attention
Pattern Recognition(2023)
摘要
Bottom-up human pose estimation models detect keypoints and learn associative information between keypoints, usually requiring human predefined offset fields or embeddings for keypoints grouping (clus-tering). In this paper, we present a brand new method that can entirely solve these problems based on Transformer, making the grouping process free of the human-defined associative signals. Specifically, the self-attention in vision Transformer measures feature similarity between any pair of locations, which pro-vides a metric space to associate keypoints together into corresponding human instances. However, the naive attention patterns formed in Transformer are still not subjectively controlled, so there is no guar-antee that the keypoints only attend to the instances to which they belong. To address it we propose a novel approach of supervising self-attention to be instance-aware, simultaneously accomplishing multi -person keypoint detection and clustering. By doing so, we can group the detected keypoints to their corresponding instances, according to the pairwise attention scores.An additional benefit of our method is that the instance segmentation results of any number of people can be directly obtained from the supervised attention matrix, thereby simplifying the pixel assignment pipeline. The qualitative and quantitative results on the COCO shows that, with a very simple architecture design, our method can achieve comparable performance against the CNN-based bottom-up counterparts with fewer parameters, which also demonstrate a promising way to control self-attention mechanism behavior for specific purposes.(c) 2022 Elsevier Ltd. All rights reserved.
更多查看译文
关键词
Multi-person human pose estimation,Self-attention,Bottom-up,Transformer,Grouping,Keypoints association
AI 理解论文
溯源树
样例
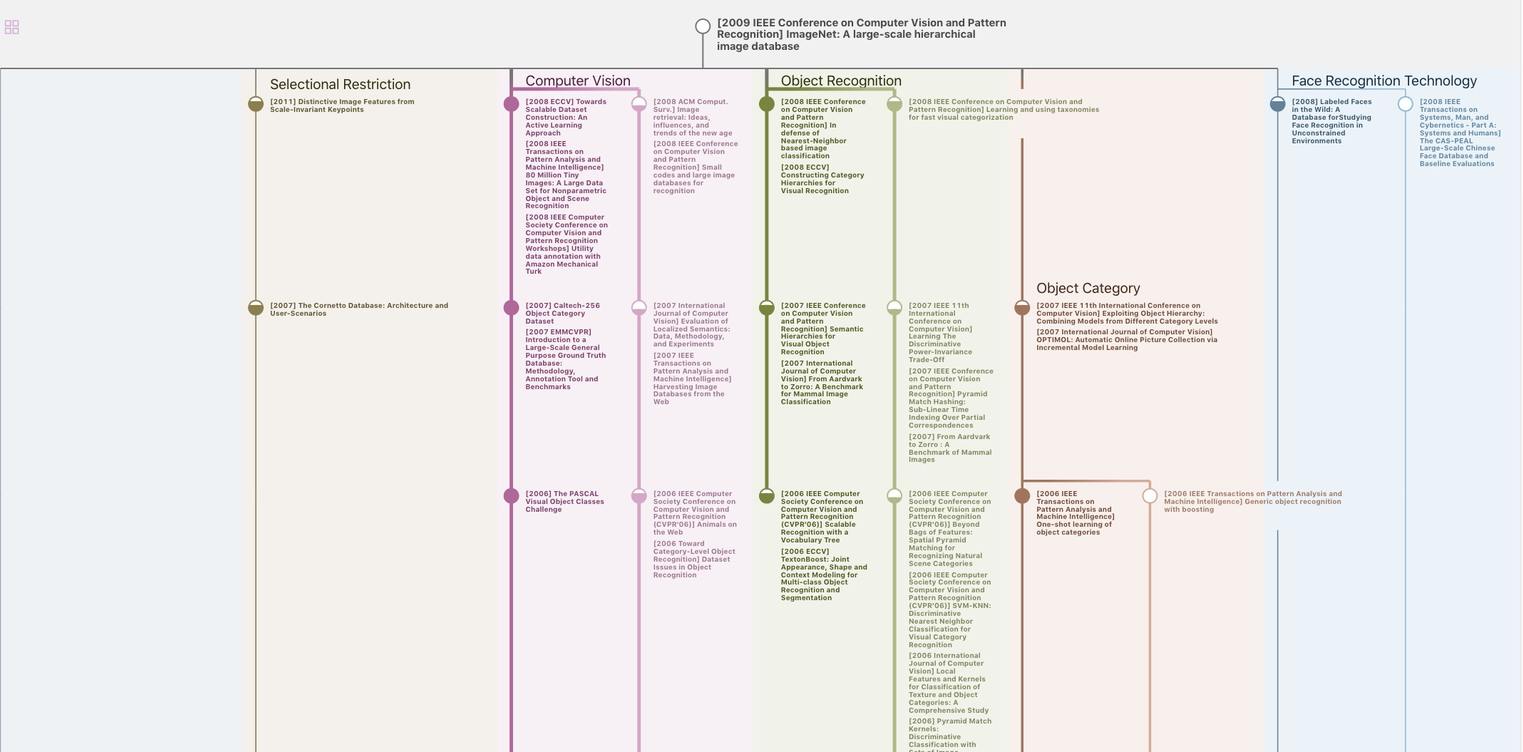
生成溯源树,研究论文发展脉络
Chat Paper
正在生成论文摘要