Cloudless-Training: A Framework to Improve Efficiency of Geo-Distributed ML Training
arxiv(2023)
摘要
Geo-distributed ML training can benefit many emerging ML scenarios (e.g., large model training, federated learning) with multi-regional cloud resources and wide area network. However, its efficiency is limited due to 2 challenges. First, efficient elastic scheduling of multi-regional cloud resources is usually missing, affecting resource utilization and performance of training. Second, training communication on WAN is still the main overhead, easily subjected to low bandwidth and high fluctuations of WAN. In this paper, we propose a framework, Cloudless-Training, to realize efficient PS-based geo-distributed ML training in 3 aspects. First, it uses a two-layer architecture with control and physical training planes to support elastic scheduling and communication for multi-regional clouds in a serverless maner.Second, it provides an elastic scheduling strategy that can deploy training workflows adaptively according to the heterogeneity of available cloud resources and distribution of pre-existing training datasets. Third, it provides 2 new synchronization strategies for training partitions among clouds, including asynchronous SGD with gradient accumulation (ASGD-GA) and inter-PS model averaging (MA). It is implemented with OpenFaaS and evaluated on Tencent Cloud. Experiments show that Cloudless-Training can support general ML training in a geo-distributed way, greatly improve resource utilization (e.g., 9.2%-24.0% training cost reduction) and synchronization efficiency (e.g., 1.7x training speedup over baseline at most) with model correctness guarantees.
更多查看译文
关键词
ml,cloudless-training,geo-distributed
AI 理解论文
溯源树
样例
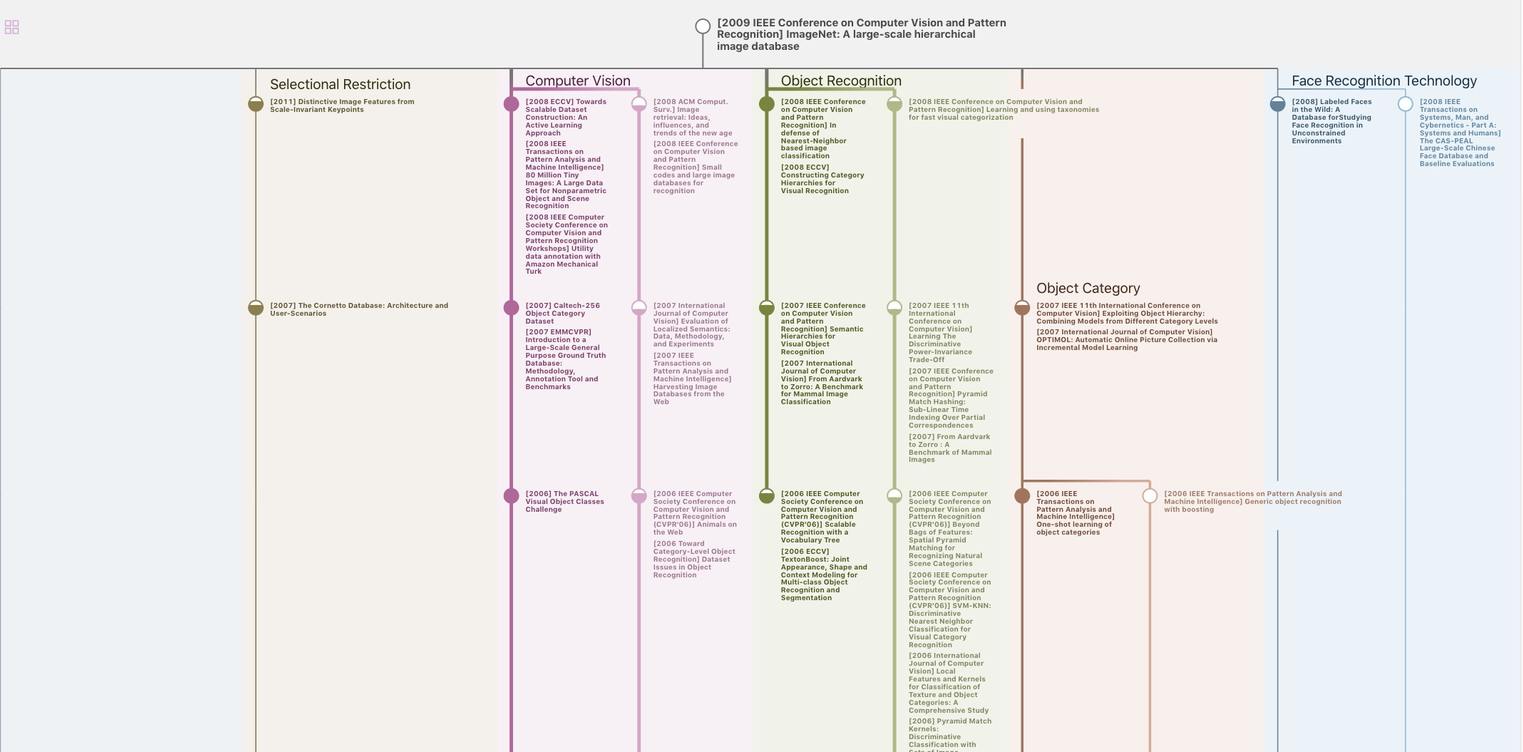
生成溯源树,研究论文发展脉络
Chat Paper
正在生成论文摘要