Blind2Sound: Self-Supervised Image Denoising without Residual Noise
arxiv(2023)
摘要
Self-supervised blind denoising for Poisson-Gaussian noise remains a challenging task. Pseudo-supervised pairs constructed from single noisy images re-corrupt the signal and degrade the performance. The visible blindspots solve the information loss in masked inputs. However, without explicitly noise sensing, mean square error as an objective function cannot adjust denoising intensities for dynamic noise levels, leading to noticeable residual noise. In this paper, we propose Blind2Sound, a simple yet effective approach to overcome residual noise in denoised images. The proposed adaptive re-visible loss senses noise levels and performs personalized denoising without noise residues while retaining the signal lossless. The theoretical analysis of intermediate medium gradients guarantees stable training, while the Cramer Gaussian loss acts as a regularization to facilitate the accurate perception of noise levels and improve the performance of the denoiser. Experiments on synthetic and real-world datasets show the superior performance of our method, especially for single-channel images.
更多查看译文
关键词
image denoising,residual noise,self-supervised
AI 理解论文
溯源树
样例
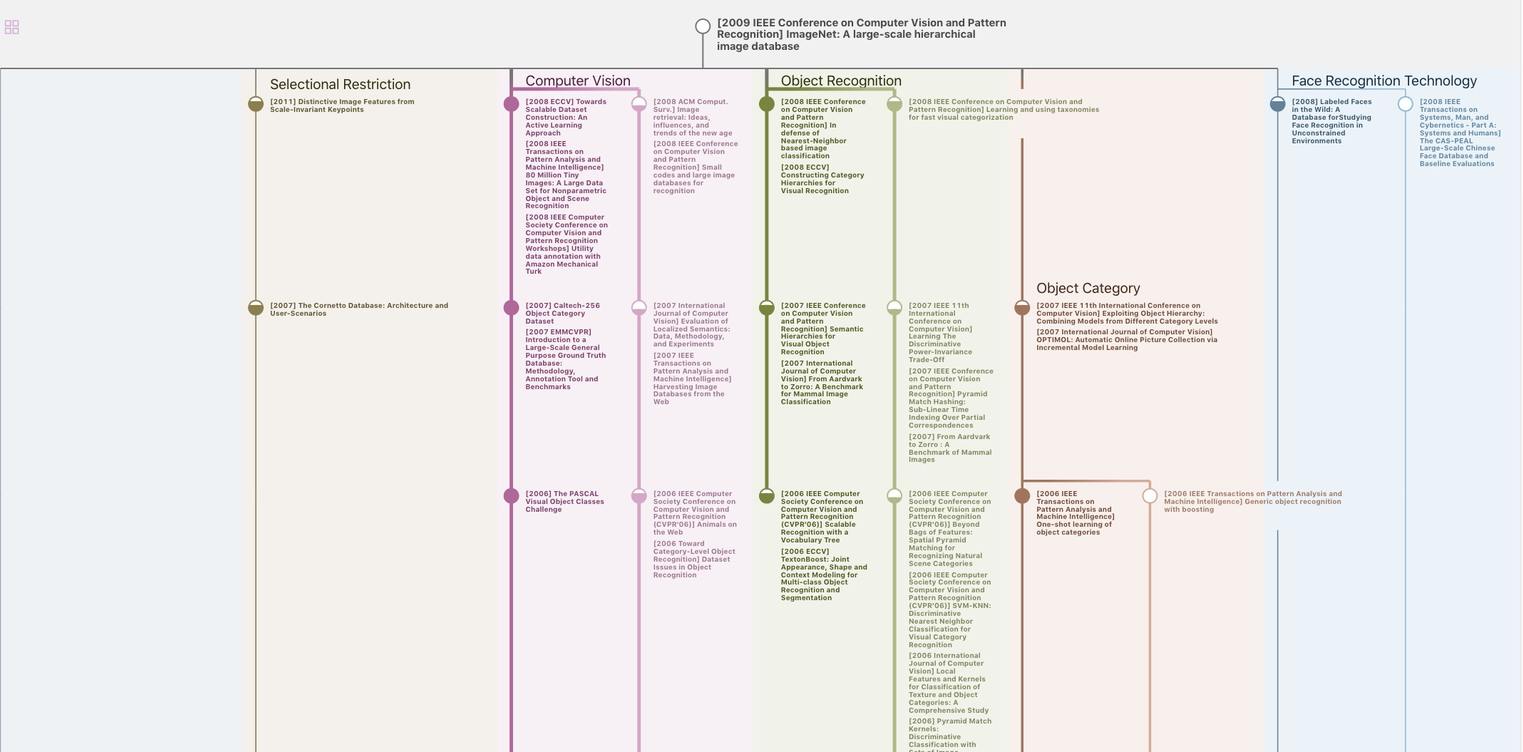
生成溯源树,研究论文发展脉络
Chat Paper
正在生成论文摘要