Using Memory-Based Learning to Solve Tasks with State-Action Constraints
arxiv(2023)
摘要
Tasks where the set of possible actions depend discontinuously on the state pose a significant challenge for current reinforcement learning algorithms. For example, a locked door must be first unlocked, and then the handle turned before the door can be opened. The sequential nature of these tasks makes obtaining final rewards difficult, and transferring information between task variants using continuous learned values such as weights rather than discrete symbols can be inefficient. Our key insight is that agents that act and think symbolically are often more effective in dealing with these tasks. We propose a memory-based learning approach that leverages the symbolic nature of constraints and temporal ordering of actions in these tasks to quickly acquire and transfer high-level information. We evaluate the performance of memory-based learning on both real and simulated tasks with approximately discontinuous constraints between states and actions, and show our method learns to solve these tasks an order of magnitude faster than both model-based and model-free deep reinforcement learning methods.
更多查看译文
关键词
continuous learned values,discontinuous constraints,discrete symbols,high-level information,memory-based learning approach,model-free deep reinforcement learning methods,reinforcement learning algorithms,state-action constraints
AI 理解论文
溯源树
样例
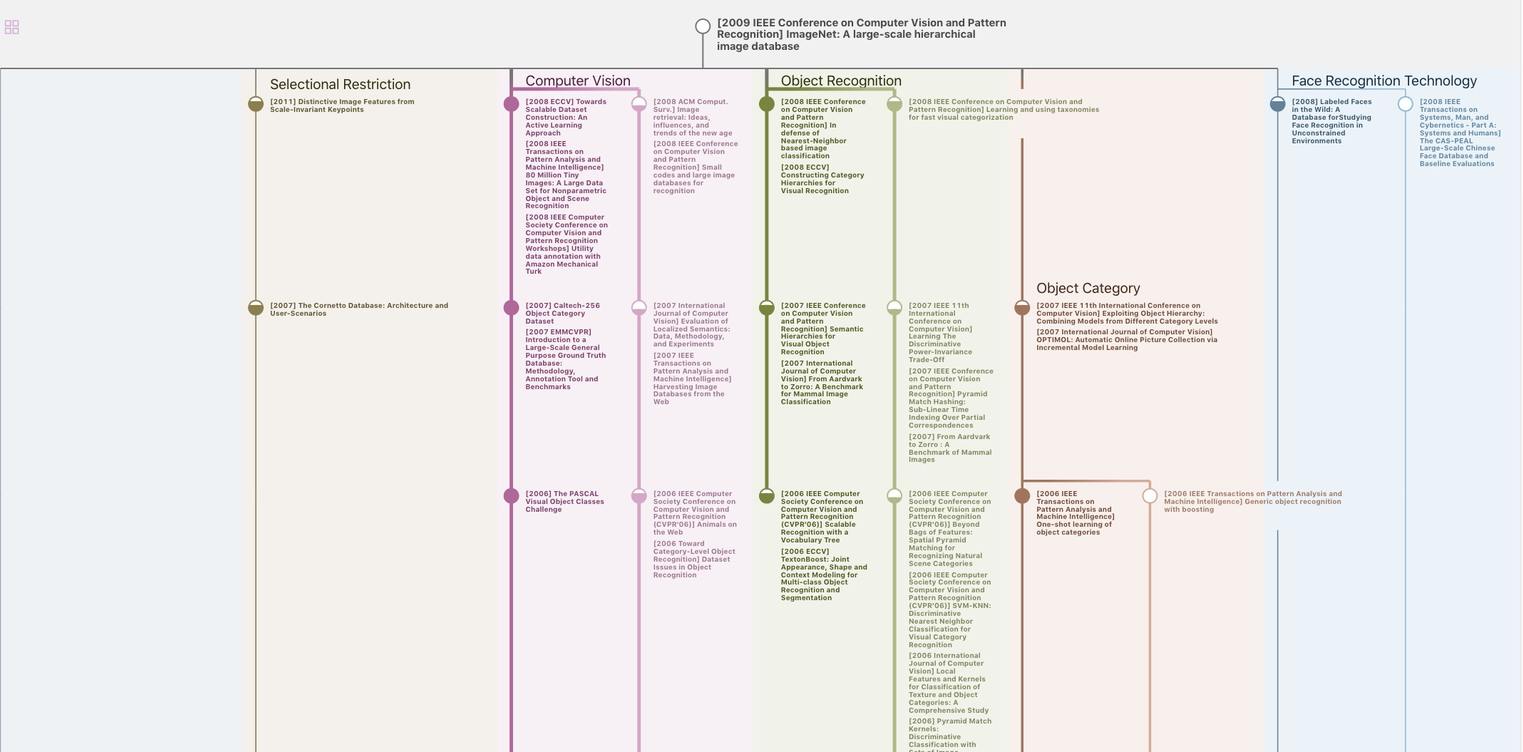
生成溯源树,研究论文发展脉络
Chat Paper
正在生成论文摘要