Outlier Removal for Fingerprinting Localization Methods
2022 56th Asilomar Conference on Signals, Systems, and Computers(2022)
摘要
Indoor localization measurements can be broadly grouped by ranging, multilateration, or RF mapping techniques. In practical implementations, these measurements are affected by the physical environment and by the non-ideal performance of sensors. Particularly for time-difference of arrival (TDoA) or angle of arrival (AoA) measurements, indoor environments naturally impose a Laplacian error distribution on the measurements. Additionally, in a distributed sensors arrangement, often only a subset of sensors may receive sufficient power for successful correlation and estimation with remaining sensors providing spurious or outlier measurements. The resulting outliers have a significant impact on localization estimation techniques that inherently utilize an L2 distance or similarity function in comparing measurements. Using data collected from a distributed, multisensor system, we utilize a sparsity-based approach to remove the outliers. We further show that removing these outliers improves performance of two separate localization estimation approaches. The first being k-NN which is the standard approach for use with RF mapping. The second approach is the data-transformation approach of kernelizing the measurements.
更多查看译文
AI 理解论文
溯源树
样例
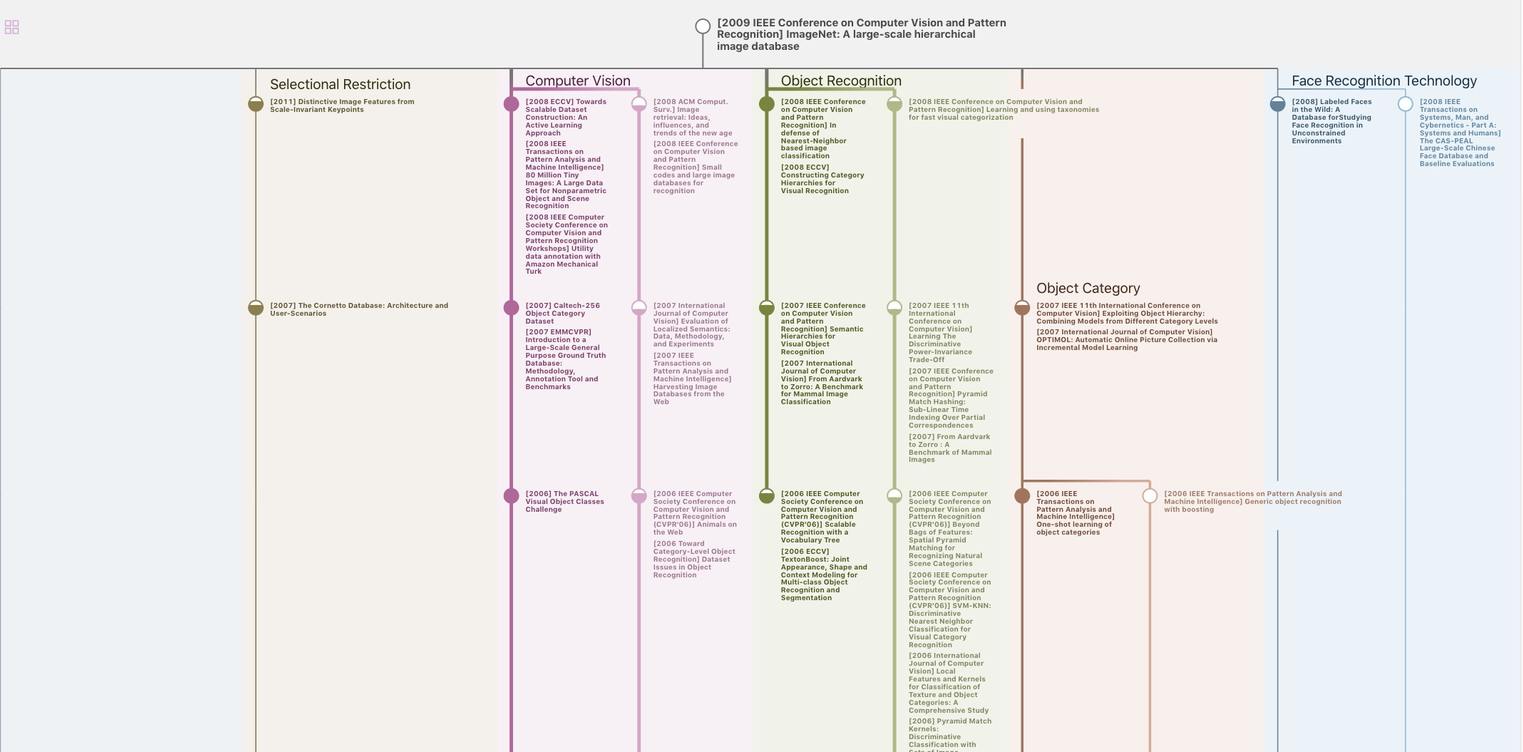
生成溯源树,研究论文发展脉络
Chat Paper
正在生成论文摘要