An Iterative Discrete Least Square Estimator with Dynamic Parameterization via Deep-Unfolding
2022 56th Asilomar Conference on Signals, Systems, and Computers(2022)
摘要
We propose a new dynamic parameterization approach via deep unfolding as an extension of the recently-introduced iterative discrete least square (IDLS) scheme, shown to elegantly generalize the conventional linear minimum mean squared error (LMMSE) method to enable the solution of inversion problems in complex multidimensional linear systems subject to discrete inputs. Configuring a layer-wise structure analogous to a deep neural network, the new approach enables an efficient optimization of the iterative IDLS algorithm, by finding optimal hyper-parameters for the related optimization problem through backpropagation and stochastic gradient descent techniques. The effectiveness of the proposed approach is confirmed via computer simulations.
更多查看译文
关键词
Discrete inversion,deep unfolding,least square,multidimensional linear systems
AI 理解论文
溯源树
样例
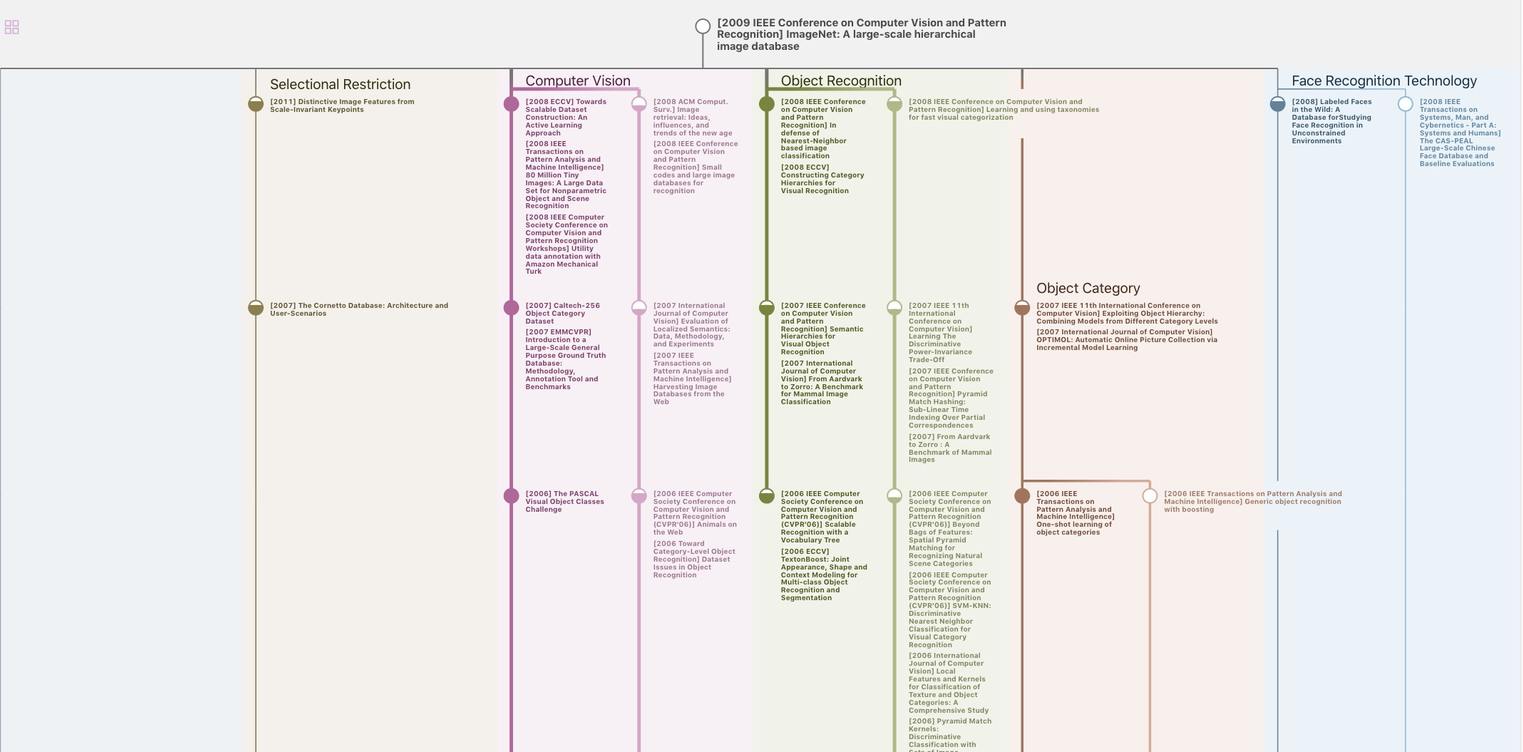
生成溯源树,研究论文发展脉络
Chat Paper
正在生成论文摘要