Predicting functional effects of ion channel variants using new phenotypic machine learning methods.
PLoS computational biology(2023)
摘要
Missense variants in genes encoding ion channels are associated with a spectrum of severe diseases. Variant effects on biophysical function correlate with clinical features and can be categorized as gain- or loss-of-function. This information enables a timely diagnosis, facilitates precision therapy, and guides prognosis. Functional characterization presents a bottleneck in translational medicine. Machine learning models may be able to rapidly generate supporting evidence by predicting variant functional effects. Here, we describe a multi-task multi-kernel learning framework capable of harmonizing functional results and structural information with clinical phenotypes. This novel approach extends the human phenotype ontology towards kernel-based supervised machine learning. Our gain- or loss-of-function classifier achieves high performance (mean accuracy 0.853 SD 0.016, mean AU-ROC 0.912 SD 0.025), outperforming both conventional baseline and state-of-the-art methods. Performance is robust across different phenotypic similarity measures and largely insensitive to phenotypic noise or sparsity. Localized multi-kernel learning offered biological insight and interpretability by highlighting channels with implicit genotype-phenotype correlations or latent task similarity for downstream analysis.
更多查看译文
关键词
functional channel variants,new phenotypic machine,machine learning
AI 理解论文
溯源树
样例
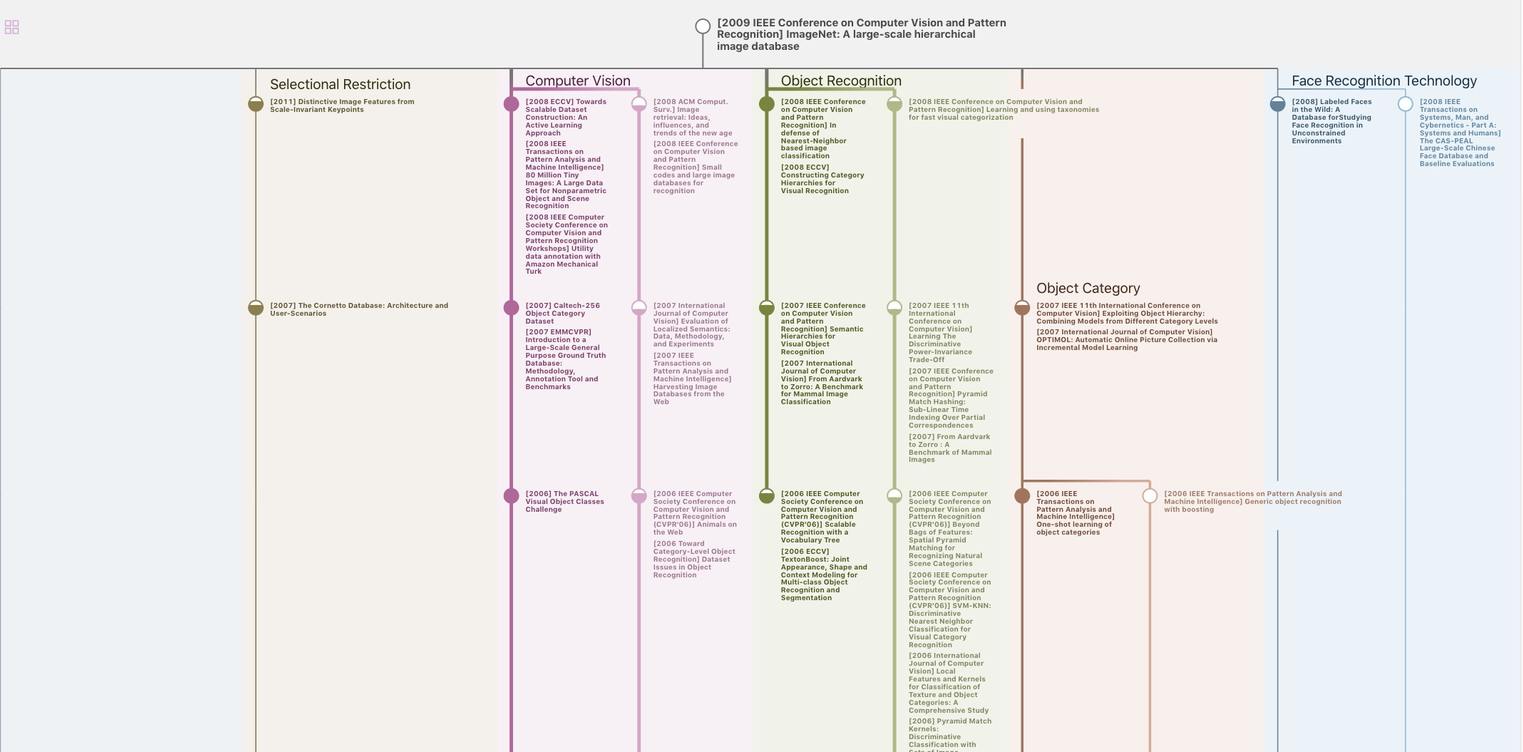
生成溯源树,研究论文发展脉络
Chat Paper
正在生成论文摘要