Learning to Backdoor Federated Learning
arxiv(2023)
摘要
In a federated learning (FL) system, malicious participants can easily embed backdoors into the aggregated model while maintaining the model's performance on the main task. To this end, various defenses, including training stage aggregation-based defenses and post-training mitigation defenses, have been proposed recently. While these defenses obtain reasonable performance against existing backdoor attacks, which are mainly heuristics based, we show that they are insufficient in the face of more advanced attacks. In particular, we propose a general reinforcement learning-based backdoor attack framework where the attacker first trains a (non-myopic) attack policy using a simulator built upon its local data and common knowledge on the FL system, which is then applied during actual FL training. Our attack framework is both adaptive and flexible and achieves strong attack performance and durability even under state-of-the-art defenses.
更多查看译文
关键词
backdoor federated learning
AI 理解论文
溯源树
样例
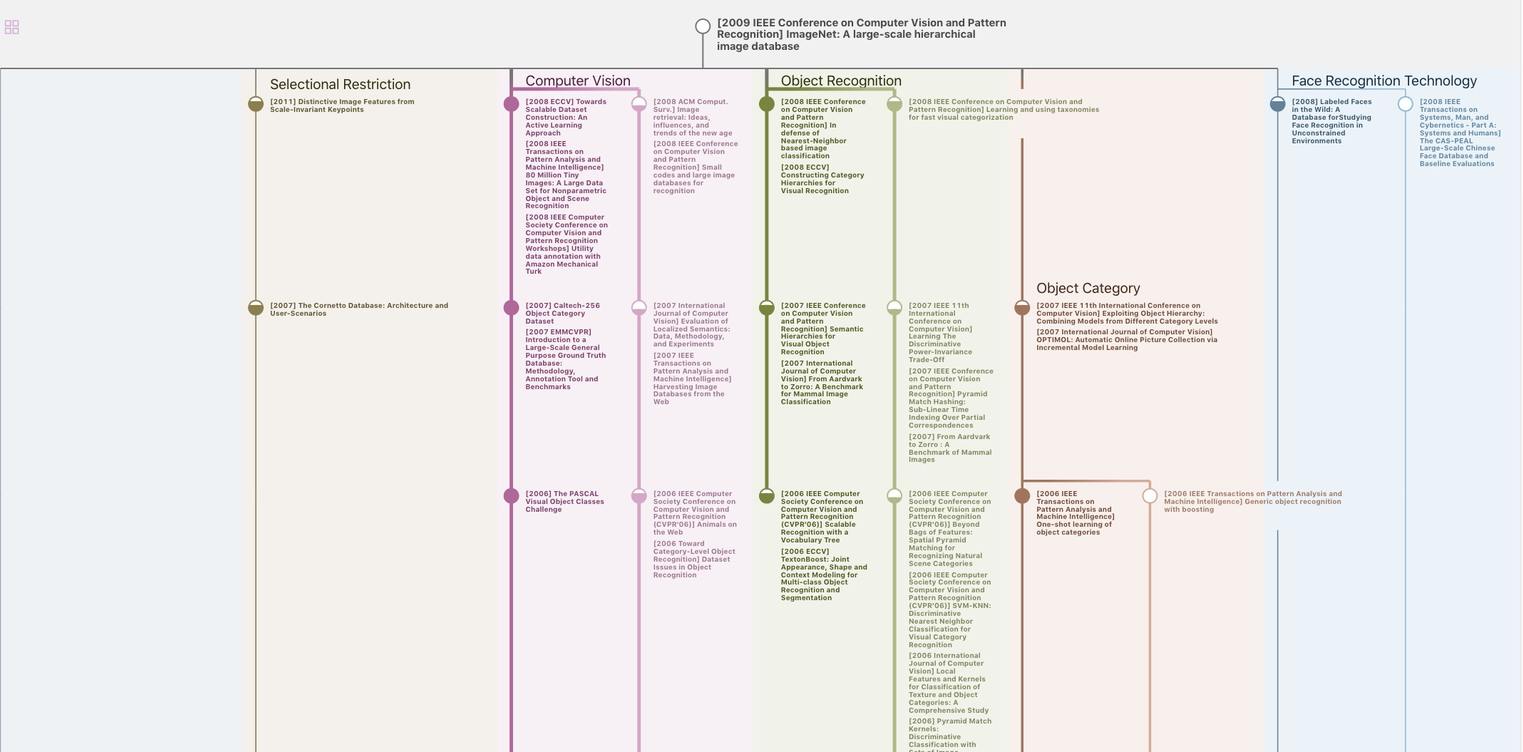
生成溯源树,研究论文发展脉络
Chat Paper
正在生成论文摘要