Data-Driven Control with Inherent Lyapunov Stability
2023 62ND IEEE CONFERENCE ON DECISION AND CONTROL, CDC(2023)
摘要
Recent advances in learning-based control leverage deep function approximators, such as neural networks, to model the evolution of controlled dynamical systems over time. However, the problem of learning a dynamics model and a stabilizing controller persists, since the synthesis of a stabilizing feedback law for known nonlinear systems is a difficult task, let alone for complex parametric representations that must be fit to data. To this end, we propose Control with Inherent Lyapunov Stability (CoILS), a method for jointly learning parametric representations of a nonlinear dynamics model and a stabilizing controller from data. To do this, our approach simultaneously learns a parametric Lyapunov function which intrinsically constrains the dynamics model to be stabilizable by the learned controller. In addition to the stabilizability of the learned dynamics guaranteed by our novel construction, we show that the learned controller stabilizes the true dynamics under certain assumptions on the fidelity of the learned dynamics. Finally, we demonstrate the efficacy of CoILS on a variety of simulated nonlinear dynamical systems.
更多查看译文
关键词
inherent lyapunov stability,control,data-driven
AI 理解论文
溯源树
样例
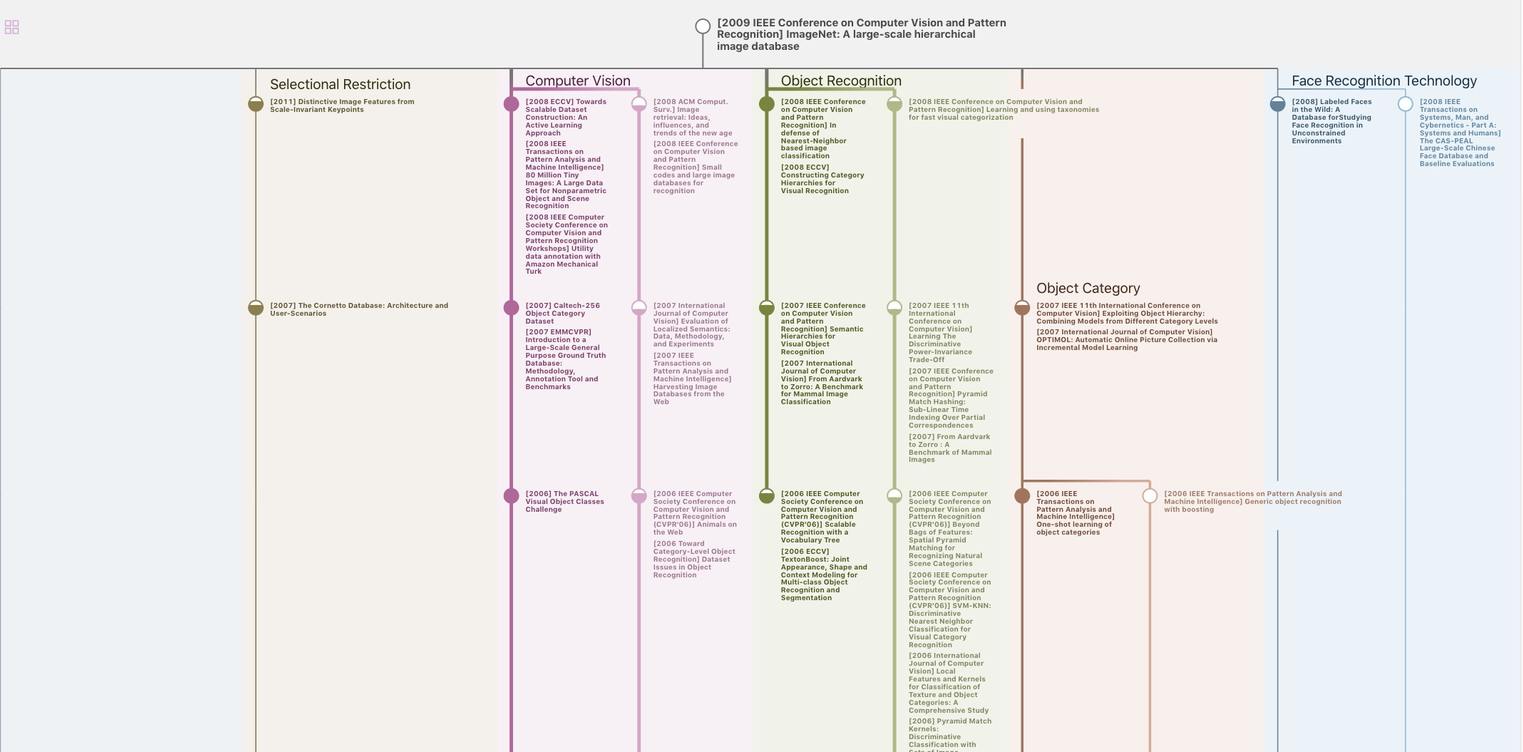
生成溯源树,研究论文发展脉络
Chat Paper
正在生成论文摘要