Metagrad: adaptive gradient quantization with hypernetworks
2023 IEEE INTERNATIONAL CONFERENCE ON IMAGE PROCESSING, ICIP(2023)
摘要
A popular track of network compression approach is Quantization aware Training (QAT), which accelerates the forward pass during the neural network training and inference. However, not much prior efforts have been made to quantize and accelerate the backward pass during training, even though that contributes around half of the training time. This can be partly attributed to the fact that errors of low-precision gradients during backward cannot be amortized by the training objective as in the QAT setting. In this work, we propose to solve this problem by incorporating the gradients into the computation graph of the next training iteration via a hypernetwork. Various experiments on CIFAR-10 dataset with different CNN network architectures demonstrate that our hypernetwork-based approach can effectively reduce the negative effect of gradient quantization noise and successfully quantizes the gradients to INT4 with only 0.64 accuracy drop for VGG-16 on CIFAR-10.
更多查看译文
关键词
Network Compression,Network Quantization,Quantization-Aware Training,Gradient Quantization,Convolution Neural Networks
AI 理解论文
溯源树
样例
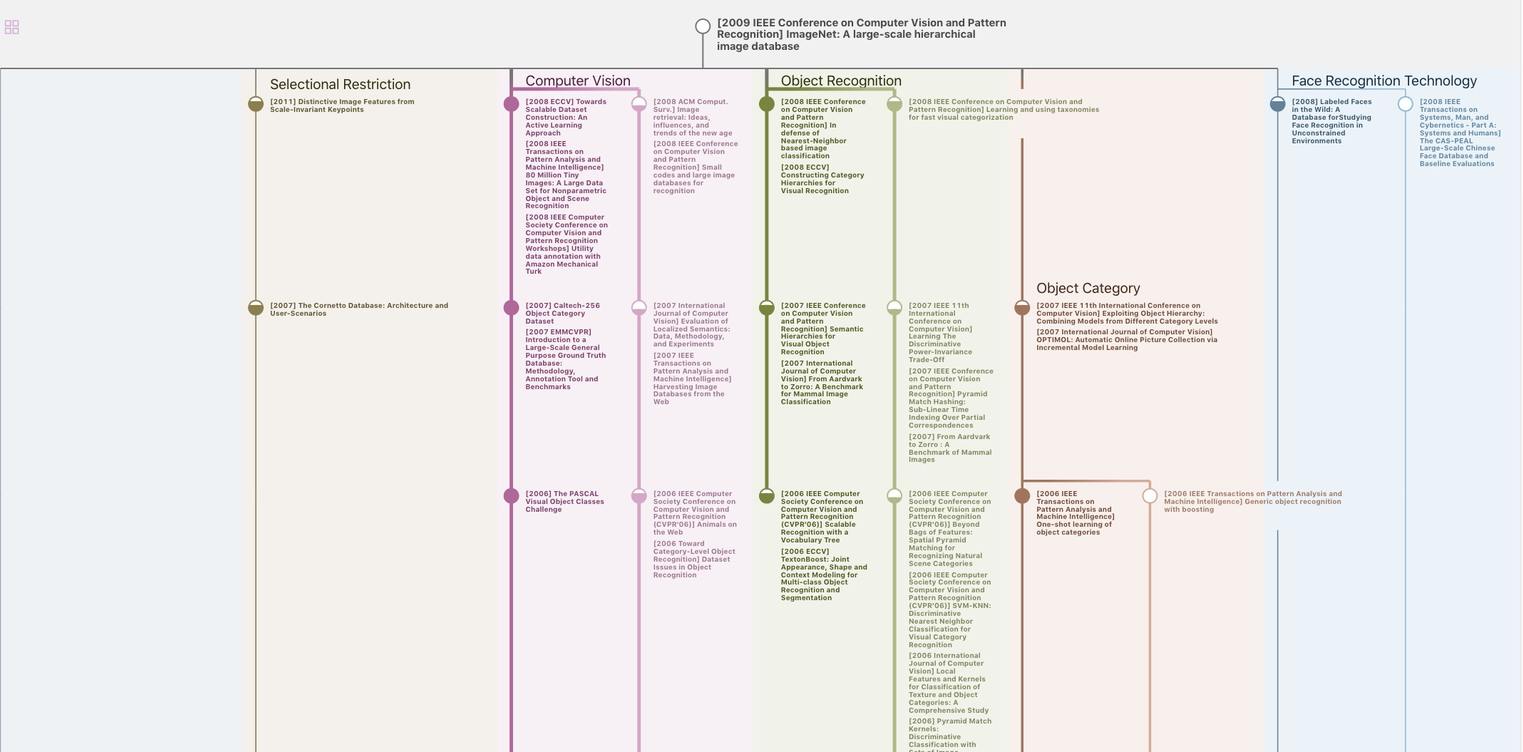
生成溯源树,研究论文发展脉络
Chat Paper
正在生成论文摘要