Visualizing Transferred Knowledge: An Interpretive Model of Unsupervised Domain Adaptation
arxiv(2023)
摘要
Many research efforts have been committed to unsupervised domain adaptation (DA) problems that transfer knowledge learned from a labeled source domain to an unlabeled target domain. Various DA methods have achieved remarkable results recently in terms of predicting ability, which implies the effectiveness of the aforementioned knowledge transferring. However, state-of-the-art methods rarely probe deeper into the transferred mechanism, leaving the true essence of such knowledge obscure. Recognizing its importance in the adaptation process, we propose an interpretive model of unsupervised domain adaptation, as the first attempt to visually unveil the mystery of transferred knowledge. Adapting the existing concept of the prototype from visual image interpretation to the DA task, our model similarly extracts shared information from the domain-invariant representations as prototype vectors. Furthermore, we extend the current prototype method with our novel prediction calibration and knowledge fidelity preservation modules, to orientate the learned prototypes to the actual transferred knowledge. By visualizing these prototypes, our method not only provides an intuitive explanation for the base model's predictions but also unveils transfer knowledge by matching the image patches with the same semantics across both source and target domains. Comprehensive experiments and in-depth explorations demonstrate the efficacy of our method in understanding the transferred mechanism and its potential in downstream tasks including model diagnosis.
更多查看译文
关键词
transferred knowledge,adaptation,interpretive model,unsupervised
AI 理解论文
溯源树
样例
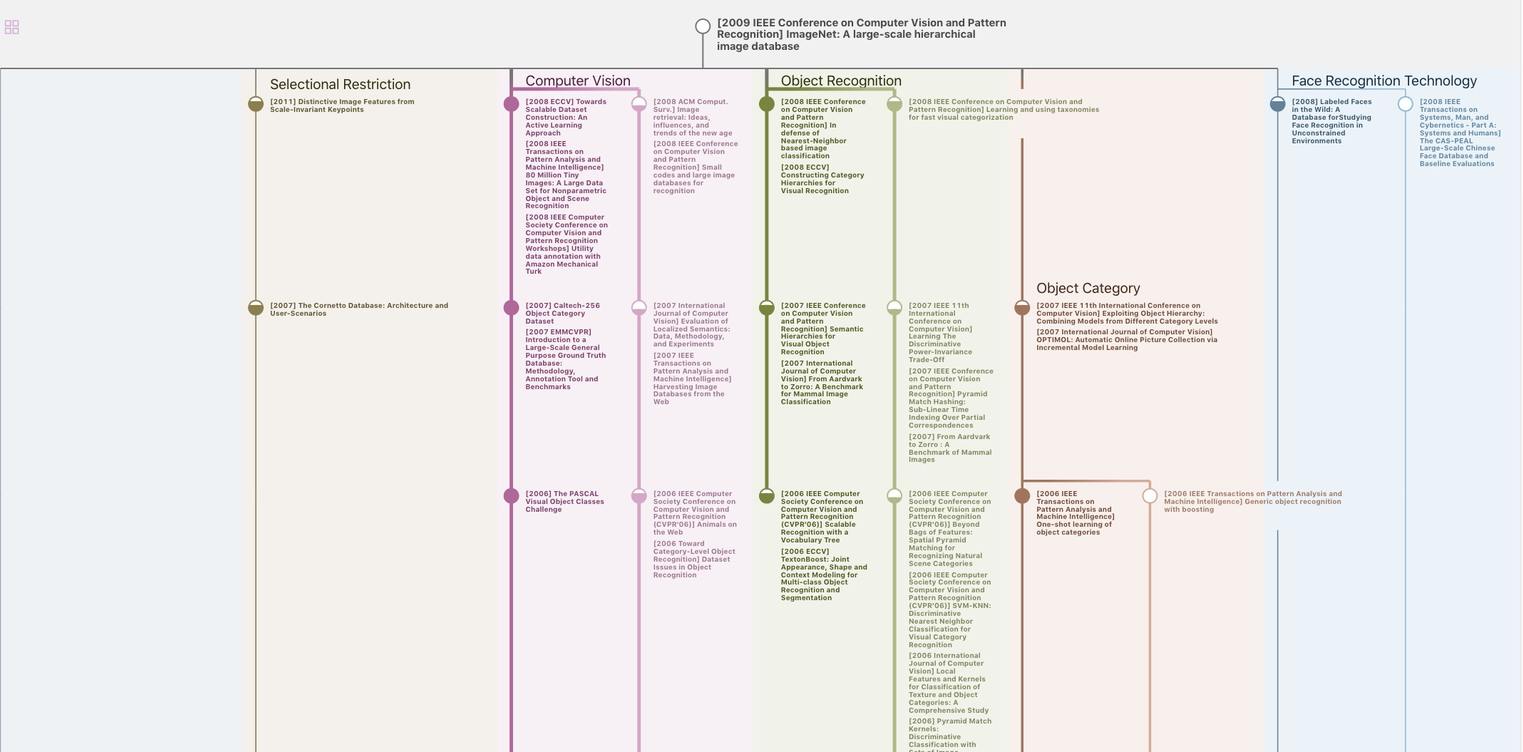
生成溯源树,研究论文发展脉络
Chat Paper
正在生成论文摘要