Counterfactual Edits for Generative Evaluation
arxiv(2023)
摘要
Evaluation of generative models has been an underrepresented field despite the surge of generative architectures. Most recent models are evaluated upon rather obsolete metrics which suffer from robustness issues, while being unable to assess more aspects of visual quality, such as compositionality and logic of synthesis. At the same time, the explainability of generative models remains a limited, though important, research direction with several current attempts requiring access to the inner functionalities of generative models. Contrary to prior literature, we view generative models as a black box, and we propose a framework for the evaluation and explanation of synthesized results based on concepts instead of pixels. Our framework exploits knowledge-based counterfactual edits that underline which objects or attributes should be inserted, removed, or replaced from generated images to approach their ground truth conditioning. Moreover, global explanations produced by accumulating local edits can also reveal what concepts a model cannot generate in total. The application of our framework on various models designed for the challenging tasks of Story Visualization and Scene Synthesis verifies the power of our approach in the model-agnostic setting.
更多查看译文
关键词
counterfactual edits,evaluation
AI 理解论文
溯源树
样例
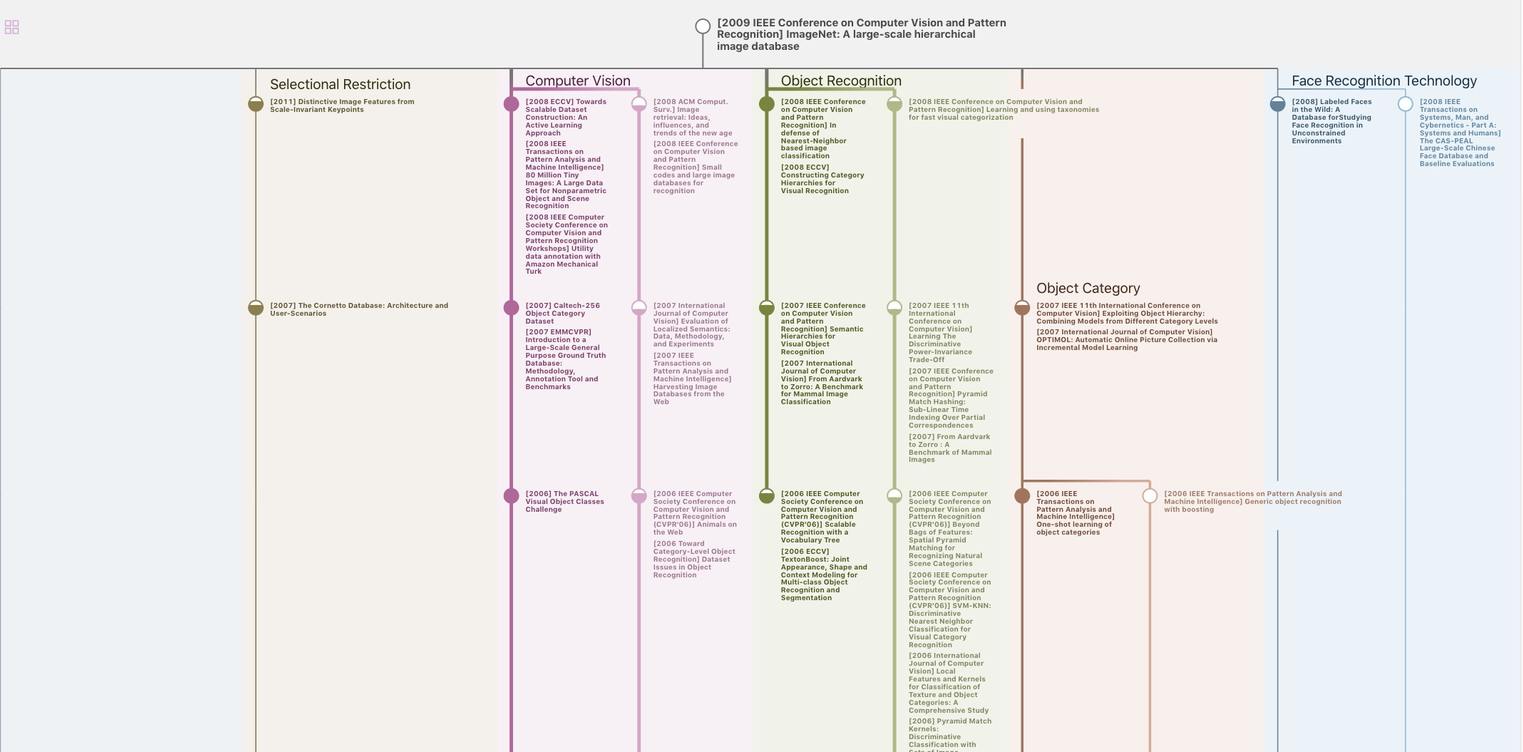
生成溯源树,研究论文发展脉络
Chat Paper
正在生成论文摘要