Determining Most Likely Links (MLL) for Network Fault Localization
IEEE Open Journal of the Communications Society(2023)
摘要
We propose and evaluate a technique that learns the probability of a network transmission link experiencing a fault by using outlier flows (in the performance sense) as training data. This technique autonomously determines the most likely links causing performance degradation in a communications network; a critical feature of zero-touch network management. Our new Network Link Outlier Factor (NLOF) with most likely links (NLOF:MLL) is experimentally compared to the existing literature (including our original NLOF) using classification performance measures: recall, precision,
$F_{1}$
-score, and time-to-detection. We utilize inferential statistics and a wide set of Mininet experiments to determine statistically significant performance differences. We find that our NLOF:MLL outperforms the existing literature wrt the important
$F_{1}$
-score while exhibiting a competitive time-to-detection.
更多查看译文
关键词
Zero-touch network management,clustering,outlier detection,data streaming,fault detection,fault localization
AI 理解论文
溯源树
样例
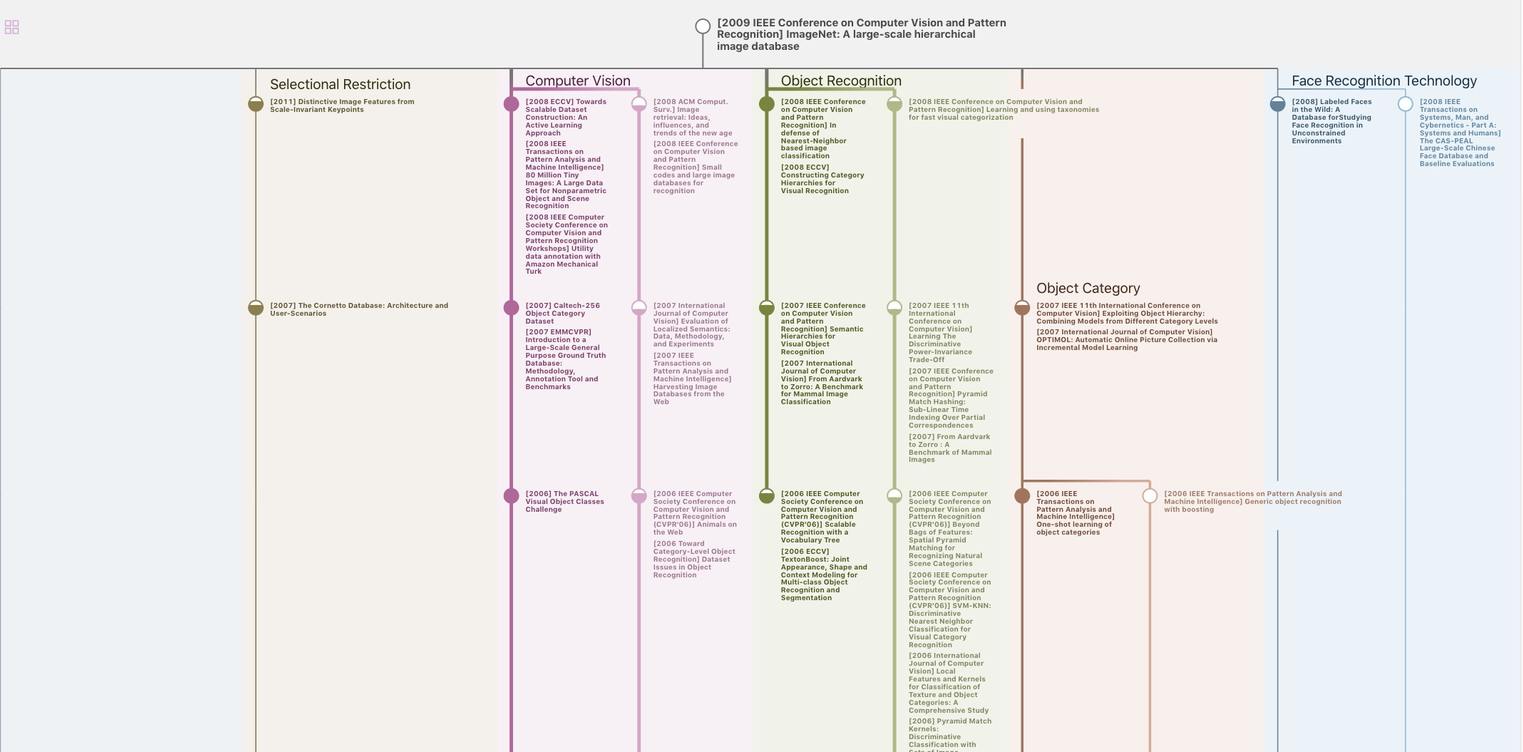
生成溯源树,研究论文发展脉络
Chat Paper
正在生成论文摘要