Machine learning-based surrogate modeling approaches for fixed-wing store separation
AEROSPACE SCIENCE AND TECHNOLOGY(2023)
摘要
In pursuit of deriving a limited expense store trajectory prediction model, this work investigates the application of two data-driven surrogate modeling approaches for the prediction of surface pressure and shear stress distributions of a store separating under supersonic conditions. Through the use of computational fluid dynamics (CFD), store separation simulations at three supersonic Mach numbers were carried out, M = 1.2, 1.4, and 1.6, for fixed-wing store separation. Pressure and shear stress distributions were recorded and used to construct a proper orthogonal decomposition (POD) and convolutional neural network (CNN) based surrogate model such that predictions of store load distributions could be obtained under new operating conditions. Load predictions are then integrated and coupled with the equations of motion to predict store trajectories at intermediate Mach numbers of M = 1.3 and M = 1.5. Results demonstrated that both the CNN and POD-based surrogate models were proven capable of providing both high fidelity distributed load and trajectory predictions at a greatly reduced computational expense. Computational run times for a single trajectory prediction simulation while using CFD simulation was 6 hours on 220 CPUs. When using the POD-based surrogate model, run time was reduced to one minute on a single CPU while the CNN-based surrogate model required 15 minutes on a single GPU.(c) 2023 Elsevier Masson SAS. All rights reserved.
更多查看译文
关键词
surrogate modeling approaches,separation,learning-based,fixed-wing
AI 理解论文
溯源树
样例
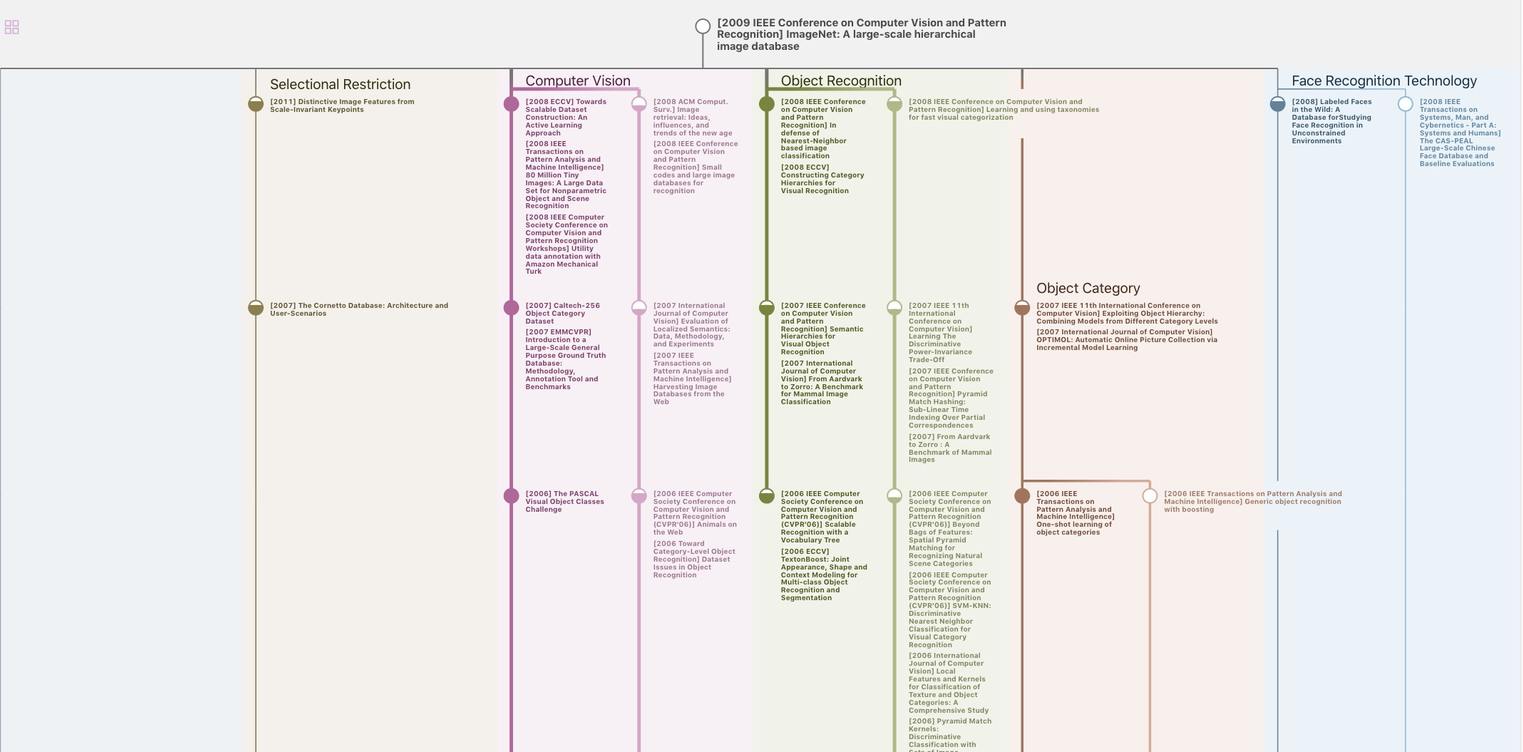
生成溯源树,研究论文发展脉络
Chat Paper
正在生成论文摘要