An empirical study of text-based machine learning models for vulnerability detection
Empir. Softw. Eng.(2023)
摘要
With an increase in complexity and severity, it is becoming harder to identify and mitigate vulnerabilities. Although traditional tools remain useful, machine learning models are being adopted to expand efforts. To help explore methods of vulnerability detection, we present an empirical study on the effectiveness of text-based machine learning models by utilizing 344 open-source projects, 2,182 vulnerabilities and 38 vulnerability types. With the availability of vulnerabilities being presented in forms such as code snippets, we construct a methodology based on extracted source code functions and create equal pairings. We conduct experiments using seven machine learning models, five natural language processing techniques and three data processing methods. First, we present results based on full context function pairings. Next, we introduce condensed functions and conduct a statistical analysis to determine if there is a significant difference between the models, techniques, or methods. Based on these results, we answer research questions regarding model prediction for testing within and across projects and vulnerability types. Our results show that condensed functions with fewer features may achieve greater prediction results when testing within rather than across. Overall, we conclude that text-based machine learning models are not effective in detecting vulnerabilities within or across projects and vulnerability types.
更多查看译文
关键词
Vulnerability detection,Machine learning,Text-based analysis
AI 理解论文
溯源树
样例
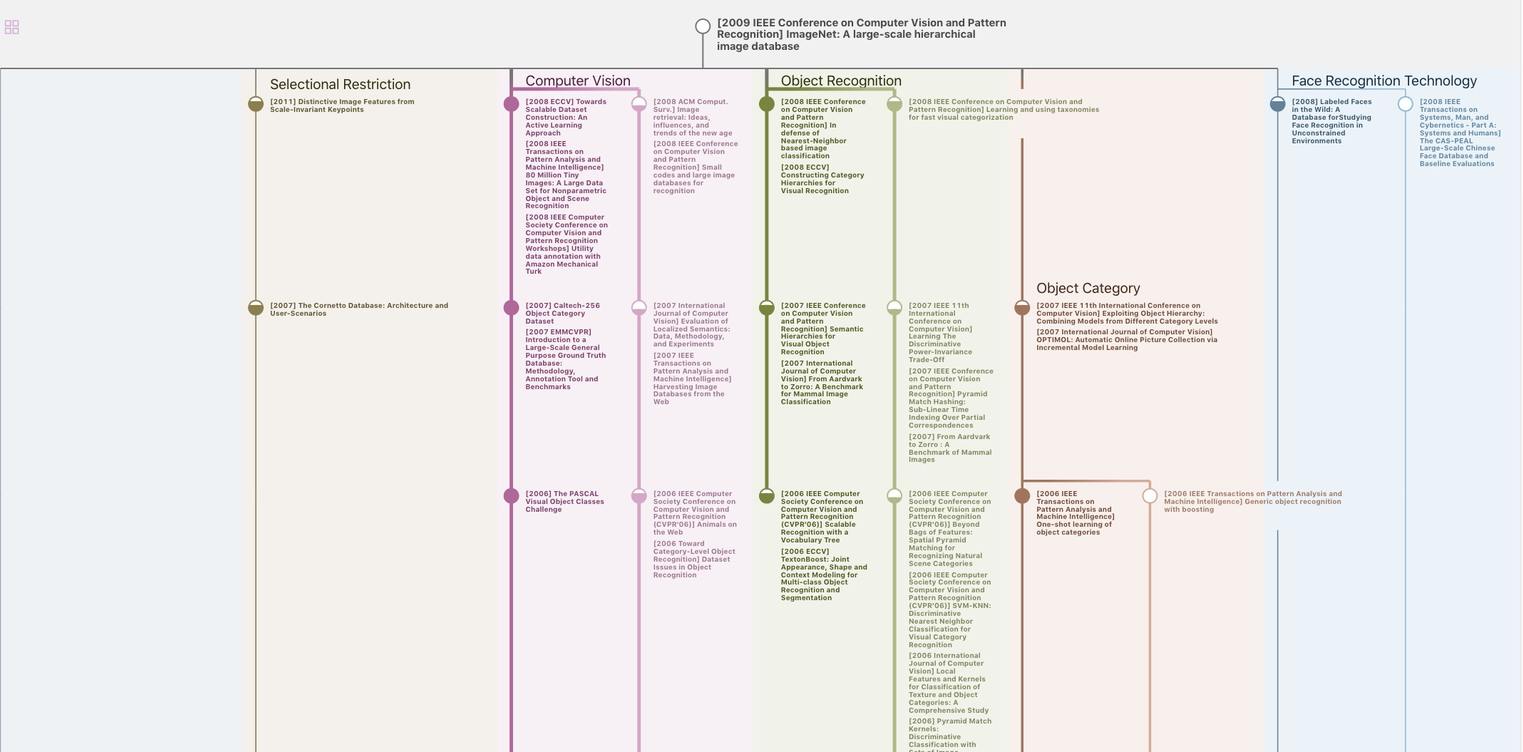
生成溯源树,研究论文发展脉络
Chat Paper
正在生成论文摘要