Evaluation of deep unsupervised anomaly detection methods with a data-centric approach for on-line inspection.
COMPUTERS IN INDUSTRY(2023)
摘要
Anomaly detection methods are used to find abnormal states, instances or data points that differ from a normal sample from the data domain space. Industrial processes are a domain where predictive models are needed for finding anomalous data instances for quality enhancement and to advance zero defect manufacturing objectives. A main challenge in this domain, however, is absence of labels for training supervised models. The potentials of domain randomisation and synthetic data in-the-loop are illustrated by a use case from additive manufacturing for automotive components. This paper contributes to a data-centric way of approaching artificial intelligence in industrial production by unsupervised anomaly detection. Based on a combination of WGAN and encoder CNN, adapted from f-AnoGAN, a solution with high potential for on-line anomaly detection is analysed. In an subsequent evaluation, we compare different metrics and neural network features for an automatic differentiation between normal or anomalous samples. We prove that using clustering methods with features generated by the discriminator yields better results than computing an anomaly score solely.
更多查看译文
关键词
Additive manufacturing,Deep unsupervised anomaly detection,Domain randomisation,Synthetic data,In-situ monitoring
AI 理解论文
溯源树
样例
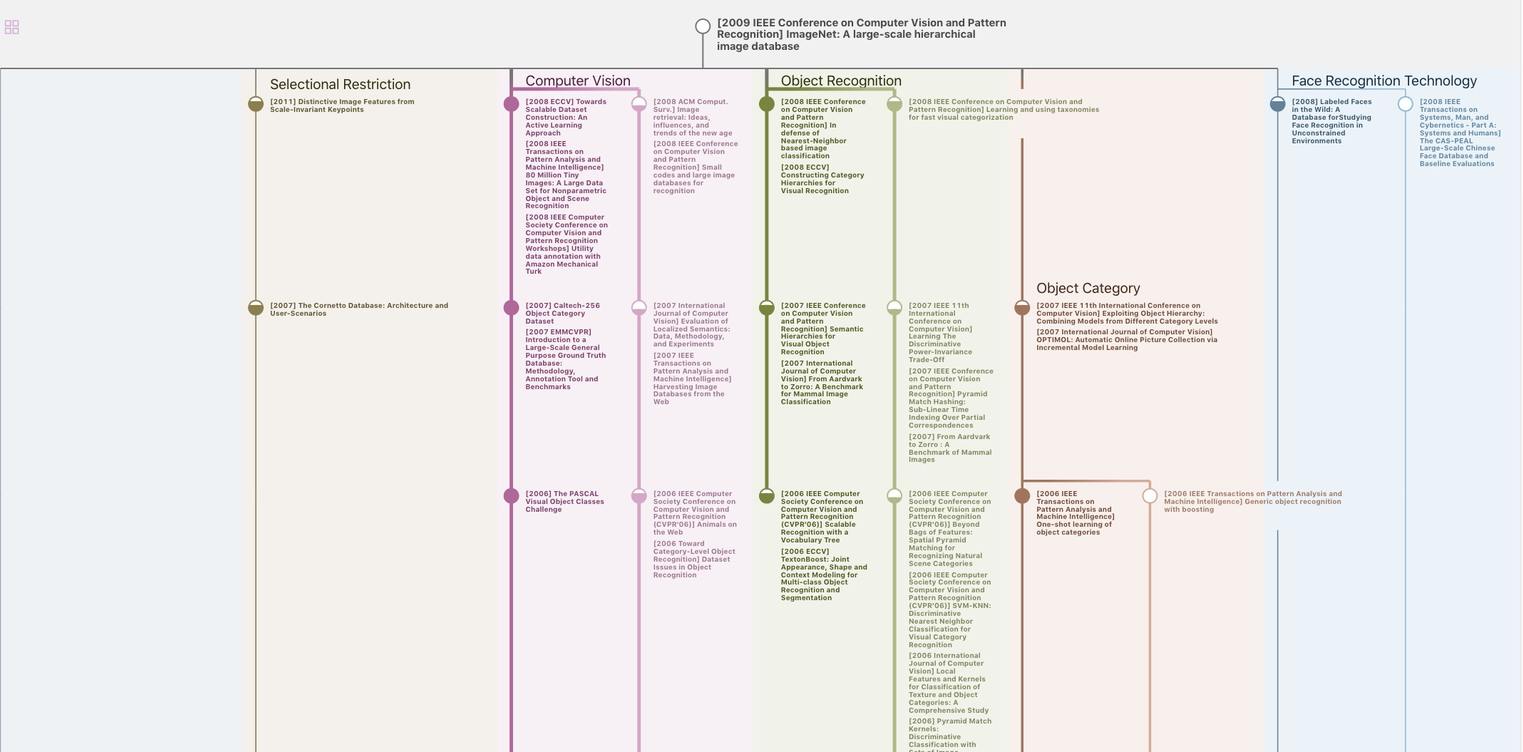
生成溯源树,研究论文发展脉络
Chat Paper
正在生成论文摘要