Structural plasticity-based hydrogel optical Willshaw model for one-shot on-the-fly edge learning
INFOMAT(2023)
摘要
Autonomous one-shot on-the-fly learning copes with the high privacy, small dataset, and in-stream data at the edge. Implementing such learning on digital hardware suffers from the well-known von-Neumann and scaling bottlenecks. The optical neural networks featuring large parallelism, low latency, and high efficiency offer a promising solution. However, ex-situ training of conventional optical networks, where optical path configuration and deep learning model optimization are separated, incurs hardware, energy and time overheads, and defeats the advantages in edge learning. Here, we introduced a bio-inspired material-algorithm co-design to construct a hydrogel-based optical Willshaw model (HOWM), manifesting Hebbian-rule-based structural plasticity for simultaneous optical path configuration and deep learning model optimization thanks to the underlying opto-chemical reactions. We first employed the HOWM as an all optical in-sensor AI processor for one-shot pattern classification, association and denoising. We then leveraged HOWM to function as a ternary content addressable memory (TCAM) of an optical memory augmented neural network (MANN) for one-shot learning the Omniglot dataset. The HOWM empowered one-shot on-the-fly edge learning leads to 1000x boost of energy efficiency and 10x boost of speed, which paves the way for the next-generation autonomous, efficient, and affordable smart edge systems.
更多查看译文
关键词
associative memory,hydrogel,one-shot learning,optical neural network,structural plasticity,Willshaw model
AI 理解论文
溯源树
样例
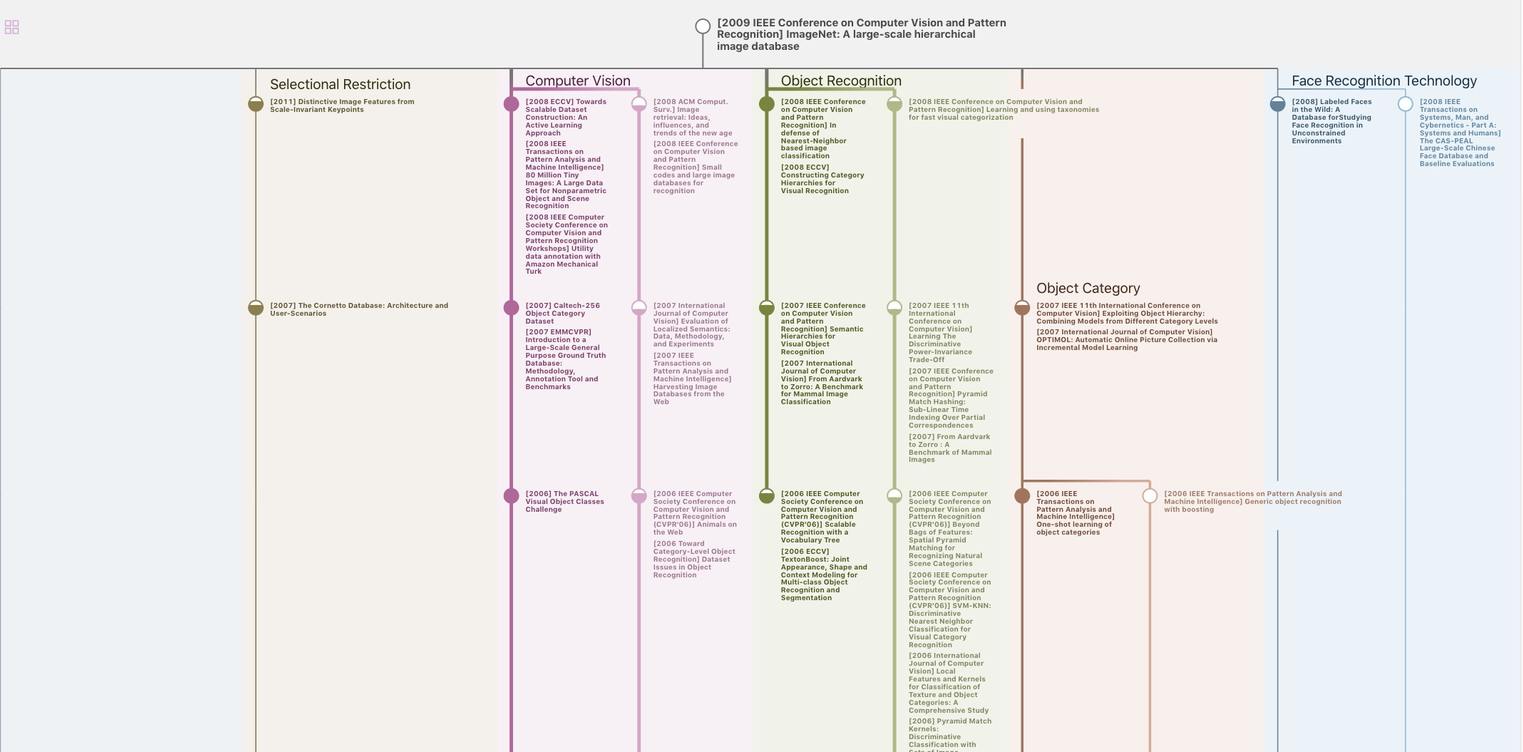
生成溯源树,研究论文发展脉络
Chat Paper
正在生成论文摘要