CWC-transformer: a visual transformer approach for compressed whole slide image classification
NEURAL COMPUTING & APPLICATIONS(2023)
摘要
The rapid development of Artificial Intelligence (AI) technology accelerates the application of computational pathology in clinical decision-making. Due to the restriction of computing resources and annotation information, it is challenging for AI-based computational pathology methods to effectively process and analyze the gigapixel whole slide image (WSI). Conventional methods utilize multiple instance learning (MIL) to convert WSI into patches for classification. However, without the patch-level annotation, it is difficult to extract discriminative features, even with pre-trained networks. Furthermore, forcibly applying the patch-level conversion will break the pathological characteristics of WSI from the spatial structure. In this study, we present a two-stage framework named Compressed WSI Classification (CWC-Transformer) to effectively solve the problems of feature extraction and spatial information loss in WSI classification. In the compression stage, we adopt contrastive learning to present a feature compression method, which not only extracts the discriminative features but also decreases the data deviation caused by staining and scanning inconsistency. In the learning stage, we extend the advantages of the convolutional neural network and transformer mechanism to enhance the co-relations between local and global information to provide the final results jointly. Experiments on three large-scale public datasets of different tasks show that our proposed framework outperforms other advanced methods in terms of robustness and interpretation.
更多查看译文
关键词
Whole slide image,Deep learning,Vision transformer,Medical image analysis,Computer-aided diagnosis
AI 理解论文
溯源树
样例
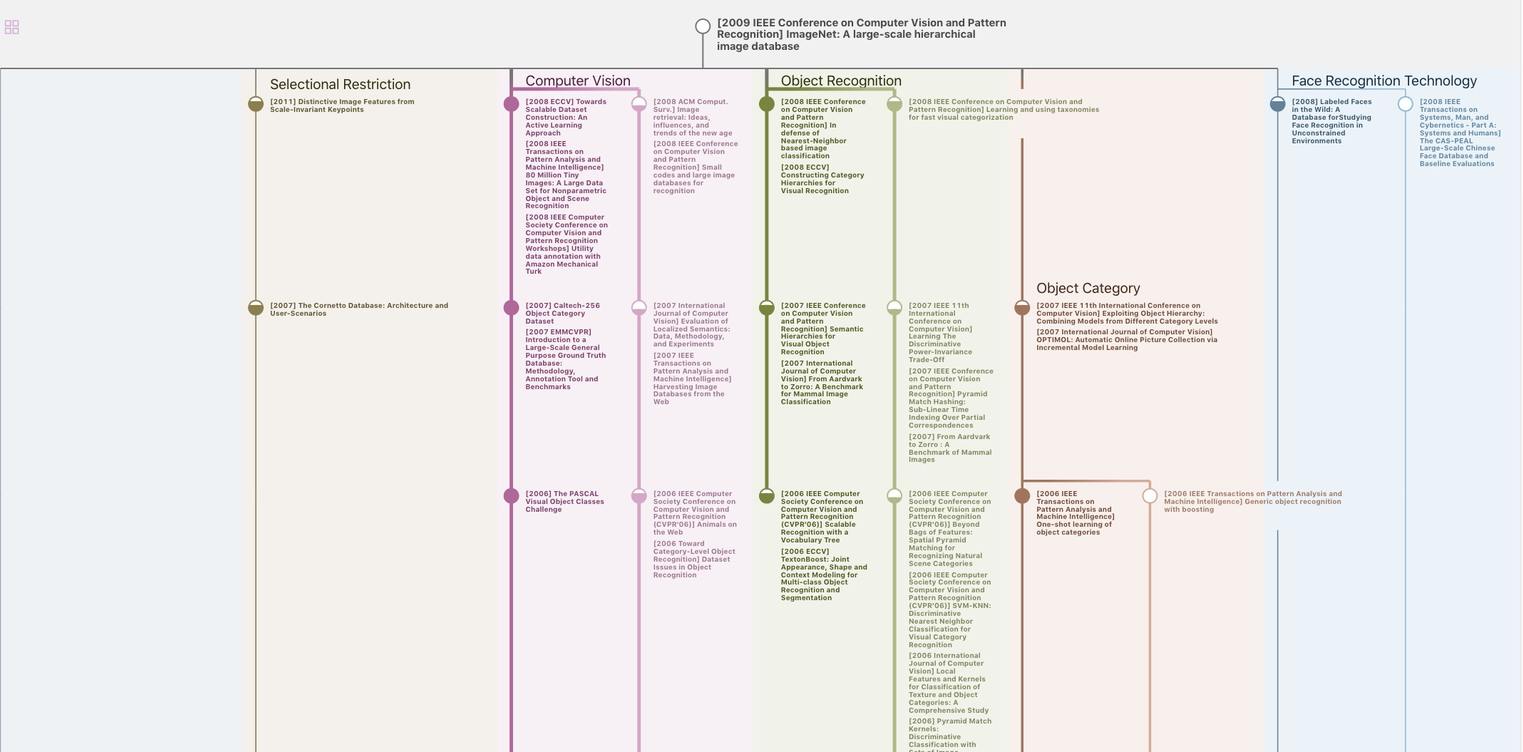
生成溯源树,研究论文发展脉络
Chat Paper
正在生成论文摘要