Dilated Multi-Activation Autoencoder to Improve the Performance of Sound Separation Mechanisms
INTERNATIONAL JOURNAL OF ADVANCED COMPUTER SCIENCE AND APPLICATIONS(2022)
摘要
Speech enhancement is the process of improving the quality of audio relative to target speaker while suppressing other sounds. It can be used in many applications as speech recognition, mobile phone, hearing aids and also enhancing audio files resulted from separation models. In this paper, a convolutional neural network (CNN) architecture is proposed to improve the quality of target's speaker resulted from speech separation models without having any prior information about the background sounds. The proposed model consists of three main phases: Pre-Processing phase, Autoencoder phase and Retrieving Audio phase. The pre-processing phase converts audio to short time Fourier transform (STFT) domain. Autoencoder phase consists of two main modules: dilated multi-Activation encoder and dilated multi- Activation decoder. Dilated multiActivation encoder module has a six blocks with different dilation factors and each block consists of three CNN layers where each layer has different activation function then the encoder's blocks are arranged in reverse order to construct dilated multi-activation decoder. Audio retrieving phase is used to reconstruct audio depending on feature resulted from second phase. Audio files resulted from separation models are used to build our datasets that consist of 31250 files. The proposed dilated multi-activation autoencoder improved separated audios Segmental Signal-to-Noise Ratio (SNRseg) with 33.9%, Shorttime objective intelligibility (STOI) with 1.3% and reduced bark spectral distortion (BSD) with 97%.
更多查看译文
关键词
Speech de-noising,speech enhancement,speech separation,short time Fourier transform (STFT),autoencoder,dilated Convolution neural network,multi-activation functions,convolution neural network (CNN),bidirectional long short memory (BLSTM)
AI 理解论文
溯源树
样例
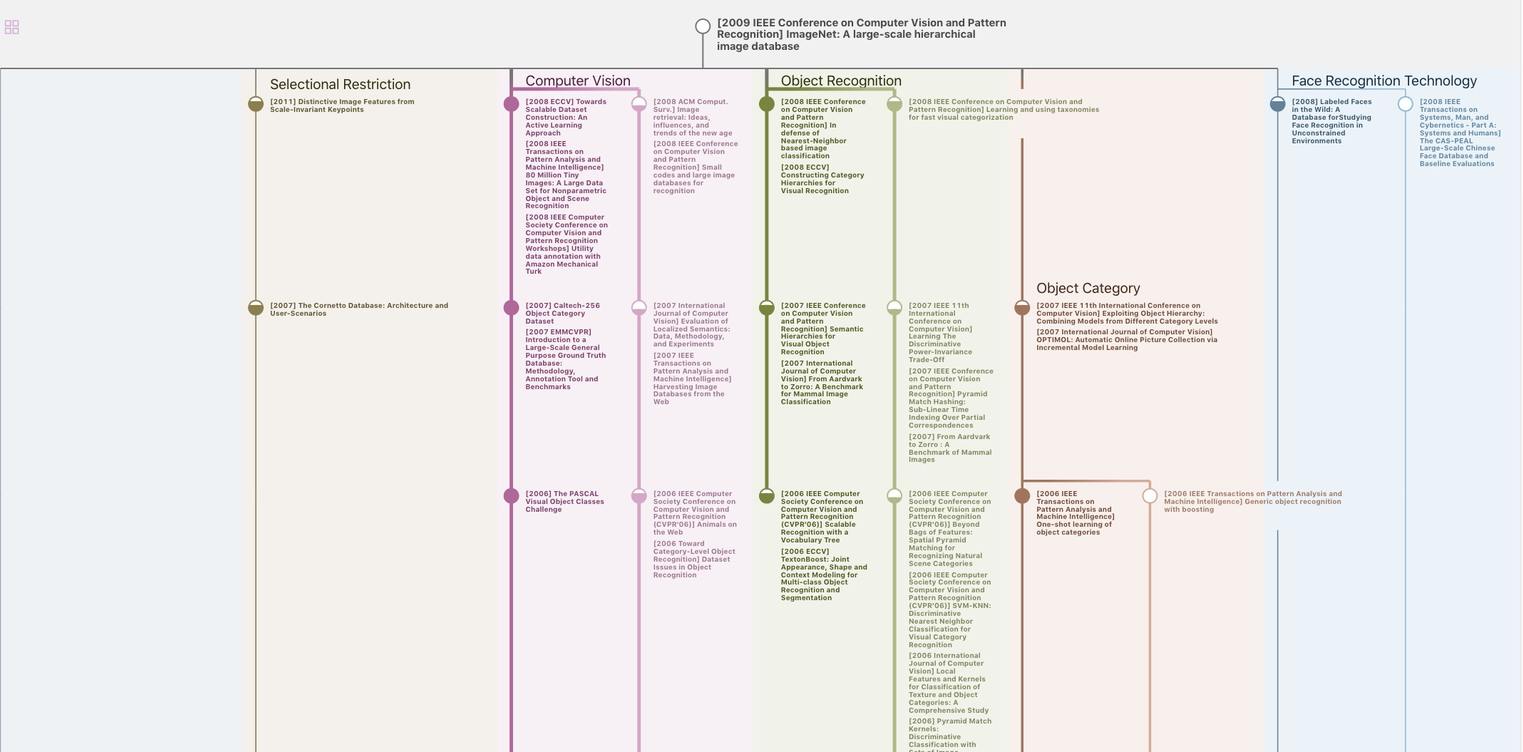
生成溯源树,研究论文发展脉络
Chat Paper
正在生成论文摘要