Isolating Features of Object and Its State for Compositional Zero-Shot Learning
IEEE TRANSACTIONS ON EMERGING TOPICS IN COMPUTATIONAL INTELLIGENCE(2023)
摘要
The purpose of Compositional Zero Shot Learning (CZSL) is to recognize previously unseen compositions of known objects (e.g. apple, banana) and their states (e.g. ripe, unripe) as seen in an image. The CZSL is a challenging problem as it is difficult to isolate the visual features of object and its states from its compositions in images. The features of a state may have wide variation across different compositions. For example, the state sliced has different visual features in compositions sliced apple and sliced tomato. In this paper, we attempt to solve the problem of CZSL using a two-stage recognition approach. Each stage sequentially performs recognition task utilising two distinct modalities of compositions. The modalities are image features as well as textual features representing features of objects and states respectively. We propose a novel gradient regularized loss term for better disentanglement of object and state features from the visual features of the composition. An appropriate disentanglement of the features of visual primitives (states and objects) leads to correct identification of images of unseen state-object compositions. The proposed approach and the competing methods are evaluated on three benchmark datasets, MIT States, UT-Zappos50 k and CGQA. Our extensive experiments establish the efficacy of our proposed algorithm that outperforms other state-of-the-art approaches.
更多查看译文
关键词
CZSL,composition,disentanglement,state-object composition,sequential learning
AI 理解论文
溯源树
样例
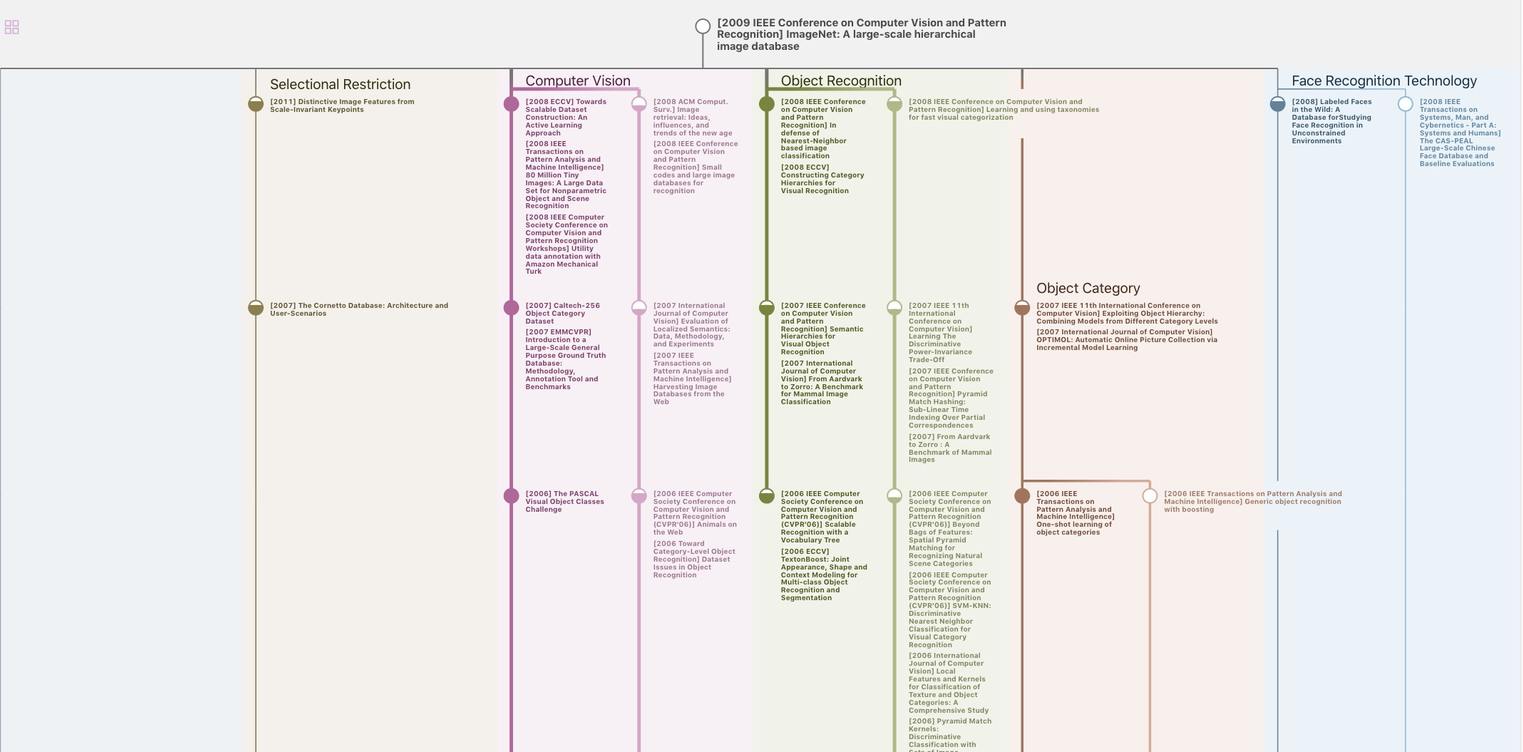
生成溯源树,研究论文发展脉络
Chat Paper
正在生成论文摘要