A Theory-Driven Deep Learning Method for Voice Chat-Based Customer Response Prediction
INFORMATION SYSTEMS RESEARCH(2023)
摘要
As artificial intelligence and digitalization technologies are flourishing real-time, online interaction-based commercial modes, exploiting customers' purchase intention implied in online interaction processes may foster huge business opportunities. In this study, we target the task of voice chat-based customer response prediction in an emerging online interaction-based commercial mode, the invite-online-and-experience-in-store mode. Prior research shows that satisfaction, which can be revealed by the discrepancy between prior expectation and actual experience, is a key factor to disentangle customers' purchase intention, whereas black-box deep learning methods empirically promise us advantageous capabilities in dealing with complex voice data, for example, text and audio information incorporated in voice chat. To this end, we propose a theory-driven deep learn-ing method that enables us to (1) learn customers' personalized product preferences and dynamic satisfaction in the absence of their profile information, (2) model customers' actual experiences based on multiview voice chat information in an interlaced way, and (3) enhance the customer response prediction performance of a black-box deep learning model with theory-driven dynamic satisfaction. Empirical evaluation results demonstrate the advantageous prediction performance of our proposed method over state-of-the-art deep learning alternatives. Investigation of cumulative satisfaction reveals the collaborative pre-dictive roles of theory-driven dynamic satisfaction and deep representation features for cus-tomer response prediction. Explanatory analysis further renders insights into customers' personalized preferences and dynamic satisfaction for key product attributes.
更多查看译文
关键词
customer response prediction,voice chat,theory-driven deep learning,dynamic satisfaction,personalized preference,cross-view attention
AI 理解论文
溯源树
样例
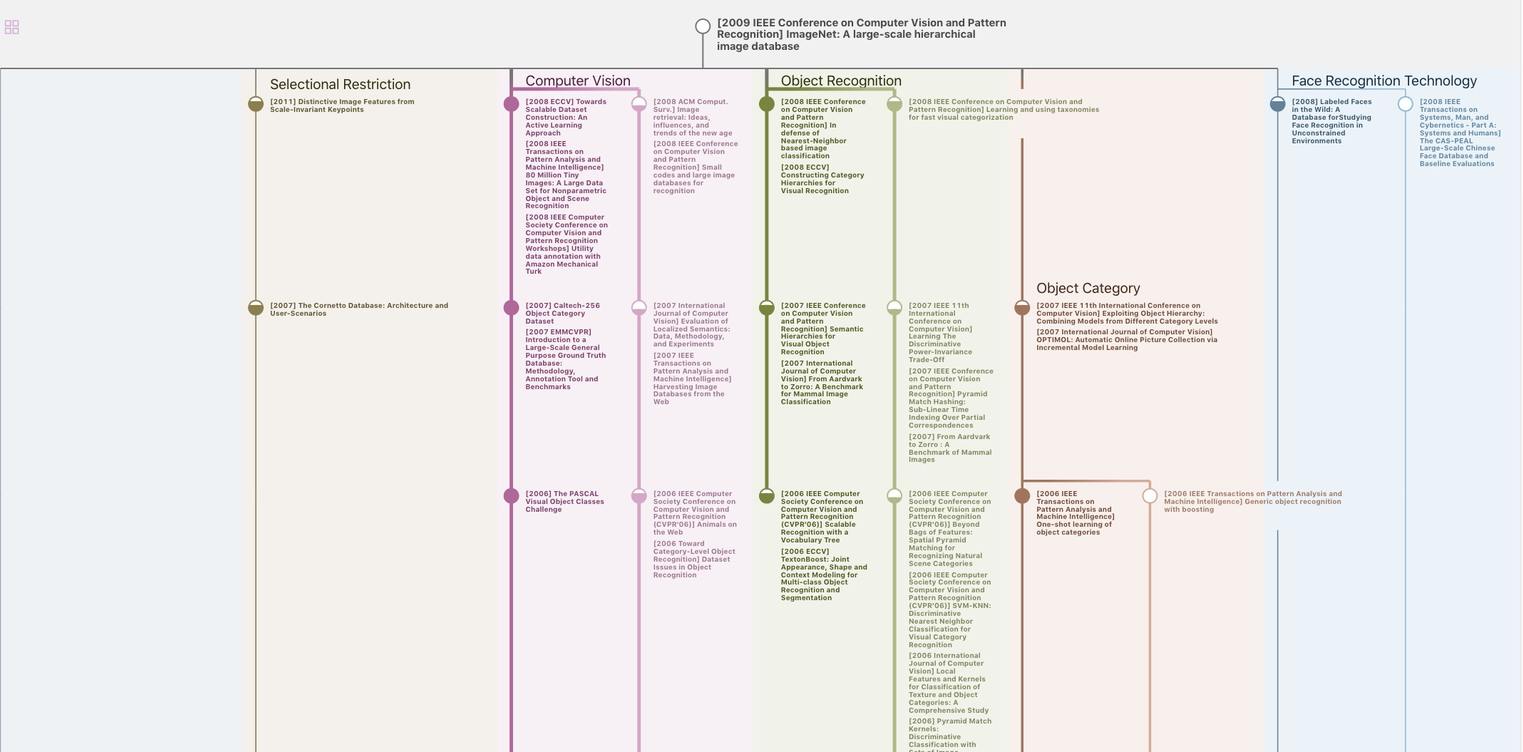
生成溯源树,研究论文发展脉络
Chat Paper
正在生成论文摘要