Federating Medical Deep Learning Models from Private Jupyter Notebooks to Distributed Institutions
APPLIED SCIENCES-BASEL(2023)
摘要
Deep learning-based algorithms have led to tremendous progress over the last years, but they face a bottleneck as their optimal development highly relies on access to large datasets. To mitigate this limitation, cross-silo federated learning has emerged as a way to train collaborative models among multiple institutions without having to share the raw data used for model training. However, although artificial intelligence experts have the expertise to develop state-of-the-art models and actively share their code through notebook environments, implementing a federated learning system in real-world applications entails significant engineering and deployment efforts. To reduce the complexity of federation setups and bridge the gap between federated learning and notebook users, this paper introduces a solution that leverages the Jupyter environment as part of the federated learning pipeline and simplifies its automation, the Notebook Federator. The feasibility of this approach is then demonstrated with a collaborative model solving a digital pathology image analysis task in which the federated model reaches an accuracy of 0.8633 on the test set, as compared to the centralized configurations for each institution obtaining 0.7881, 0.6514, and 0.8096, respectively. As a fast and reproducible tool, the proposed solution enables the deployment of a cross-country federated environment in only a few minutes.
更多查看译文
关键词
federated learning,Jupyter notebook,medical image analysis,collaborative models,cloud environment,distributed medical applications
AI 理解论文
溯源树
样例
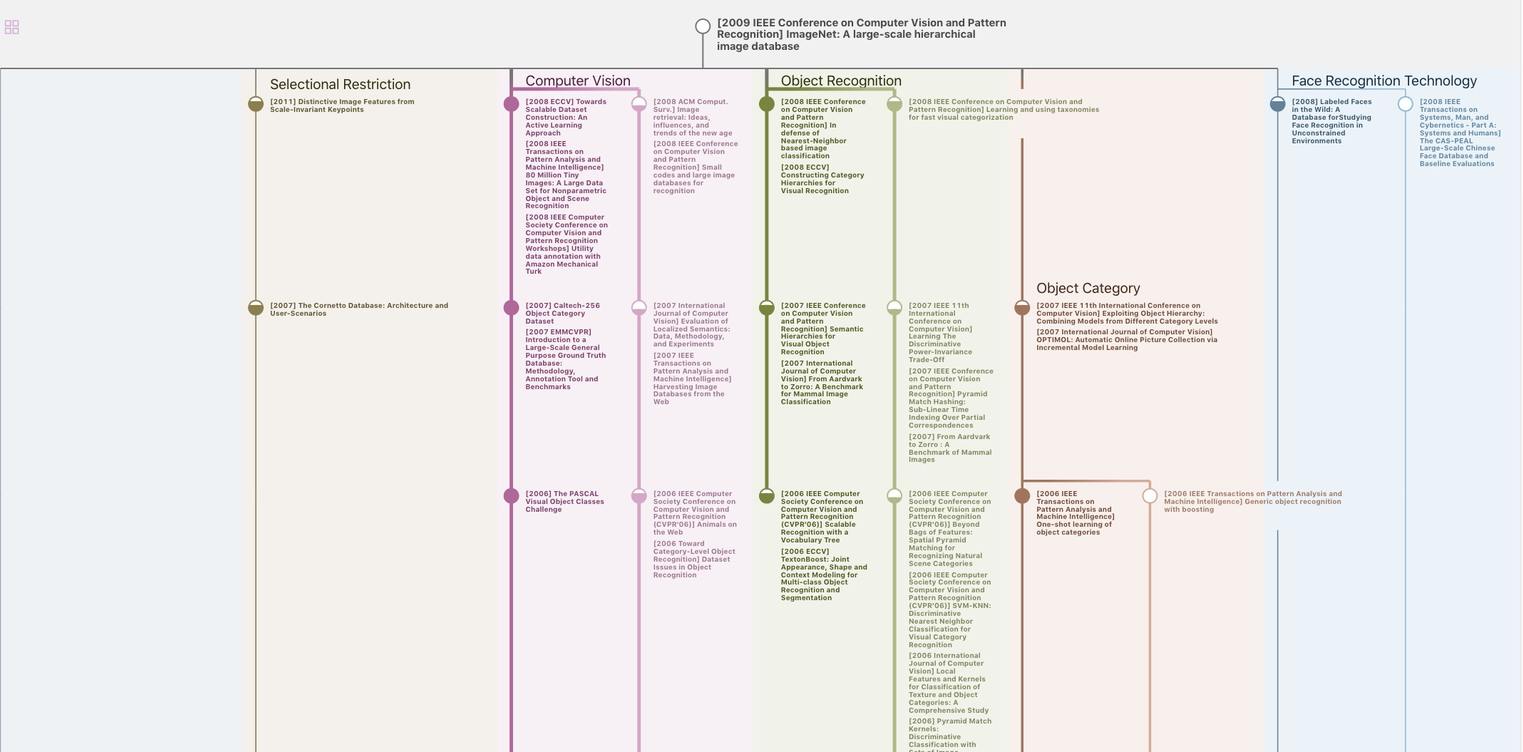
生成溯源树,研究论文发展脉络
Chat Paper
正在生成论文摘要