A novel hybrid model for lithium-ion batteries lifespan prediction with high accuracy and interpretability
JOURNAL OF ENERGY STORAGE(2023)
摘要
Machine learning can accurately predict the remaining useful life (RUL) of lithium-ion batteries because of its strong learning ability, efficient computing efficiency, and high accuracy. However, the prediction behavior and principle of many data-driven models as black box functions are unknown, and the potential of high accurate prediction requires further investigation. In view of these research gaps, this study proposes a novel hybrid model based on adaptive feature separable convolution (AFSC) and convolutional long short-term memory (ConvLSTM) network to improve the accuracy of RUL prediction and the interpretability of the model. The model extracts aging features from charging process data and can be applied to both early prediction and RUL prediction. Validation based on 124 commercial lithium iron phosphate battery aging data shows that the mean absolute error (MAE) of the early prediction results using the first 20 cycles is only 7 cycles, while the MAE of the RUL prediction is 0.12 cycles, both demonstrating excellent performance. In addition, the feature processing and prediction process of the model is analyzed through the visualization of upsampling and attention weights.
更多查看译文
关键词
Lithium-ion battery,Machine learning,Early prediction,Remaining useful life,Model interpretability
AI 理解论文
溯源树
样例
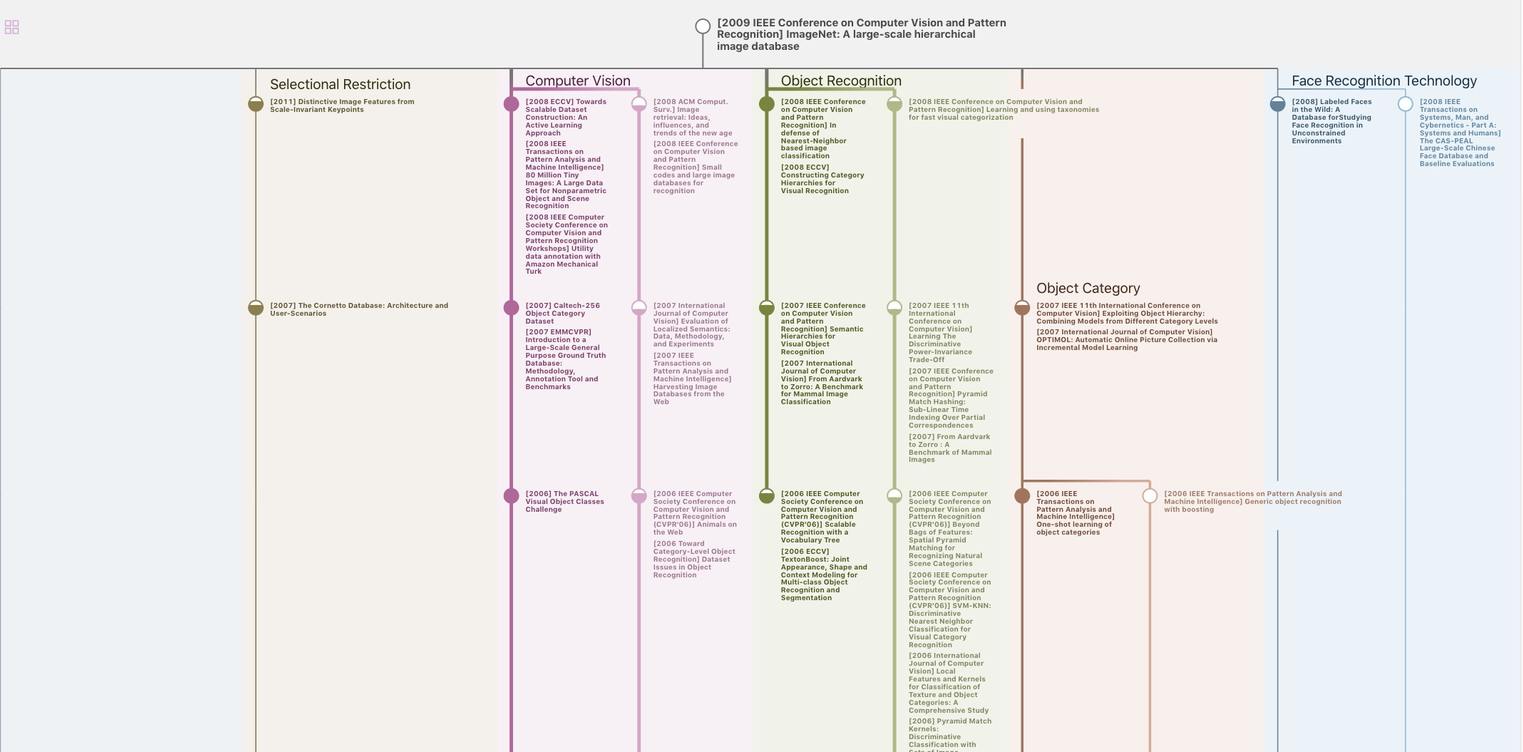
生成溯源树,研究论文发展脉络
Chat Paper
正在生成论文摘要