Adaptive Differential Privacy Mechanism Based on Entropy Theory for Preserving Deep Neural Networks
MATHEMATICS(2023)
摘要
Recently, deep neural networks (DNNs) have achieved exciting things in many fields. However, the DNN models have been proven to divulge privacy, so it is imperative to protect the private information of the models. Differential privacy is a promising method to provide privacy protection for DNNs. However, existing DNN models based on differential privacy protection usually inject the same level of noise into parameters, which may lead to a balance between model performance and privacy protection. In this paper, we propose an adaptive differential privacy scheme based on entropy theory for training DNNs, with the aim of giving consideration to the model performance and protecting the private information in the training data. The proposed scheme perturbs the gradients according to the information gain of neurons during training, that is, in the process of back propagation, less noise is added to neurons with larger information gain, and vice-versa. Rigorous experiments conducted on two real datasets demonstrate that the proposed scheme is highly effective and outperforms existing solutions.
更多查看译文
关键词
deep neural networks,differential privacy,Laplace noise
AI 理解论文
溯源树
样例
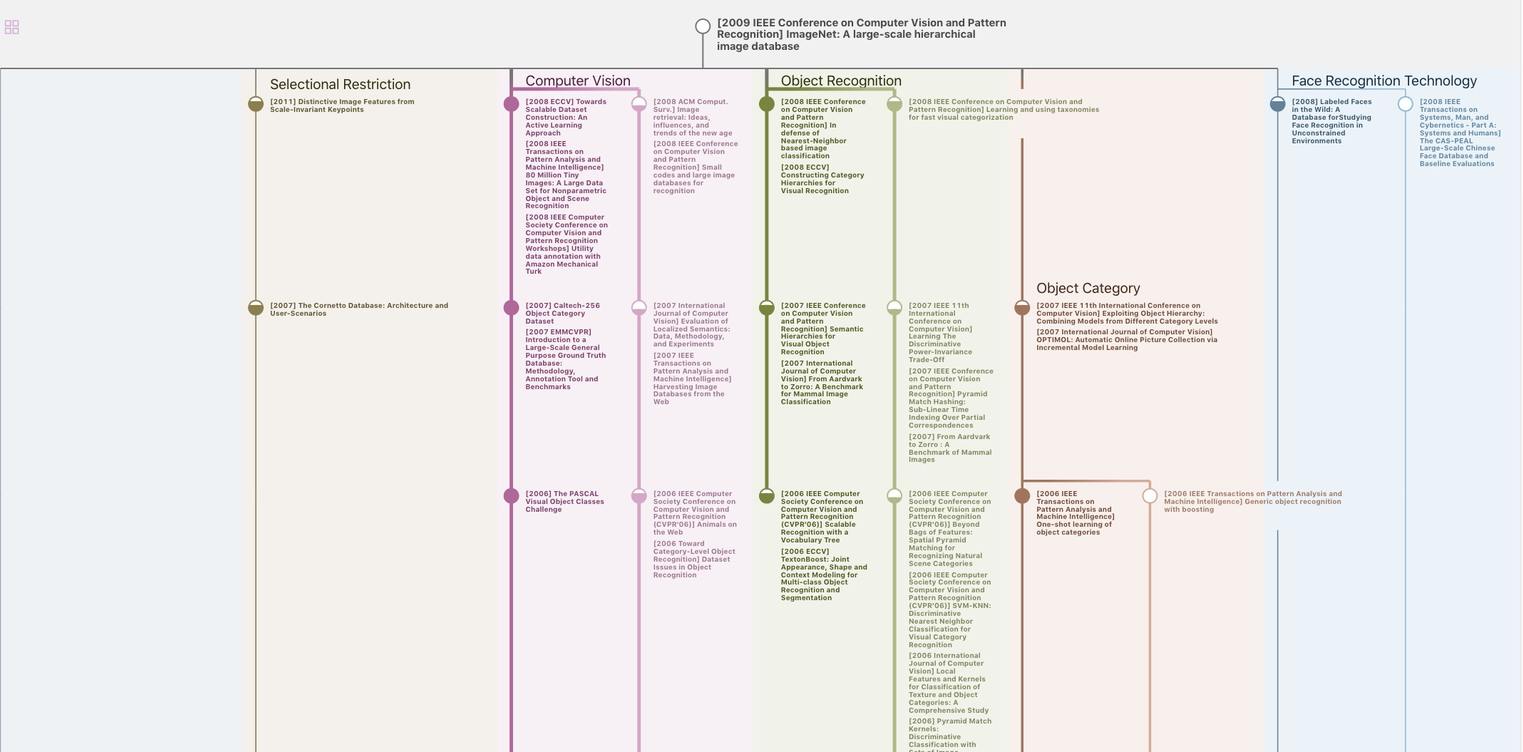
生成溯源树,研究论文发展脉络
Chat Paper
正在生成论文摘要