A Detailed Survey on Federated Learning Attacks and Defenses
ELECTRONICS(2023)
摘要
A traditional centralized method of training AI models has been put to the test by the emergence of data stores and public privacy concerns. To overcome these issues, the federated learning (FL) approach was introduced. FL employs a privacy-by-design architecture to train deep neural networks utilizing decentralized data, in which numerous devices collectively build any machine learning system that does not reveal users' personal information under the supervision of a centralized server. While federated learning (FL), as a machine learning (ML) strategy, may be effective for safeguarding the confidentiality of local data, it is also vulnerable to attacks. Increased interest in the FL domain inspired us to write this paper, which informs readers of the numerous threats to and flaws in the federated learning strategy, and introduces a multiple-defense mechanism that can be employed to fend off threats.
更多查看译文
关键词
federated learning (FL),machine learning (ML),FL attacks,defensive mechanisms
AI 理解论文
溯源树
样例
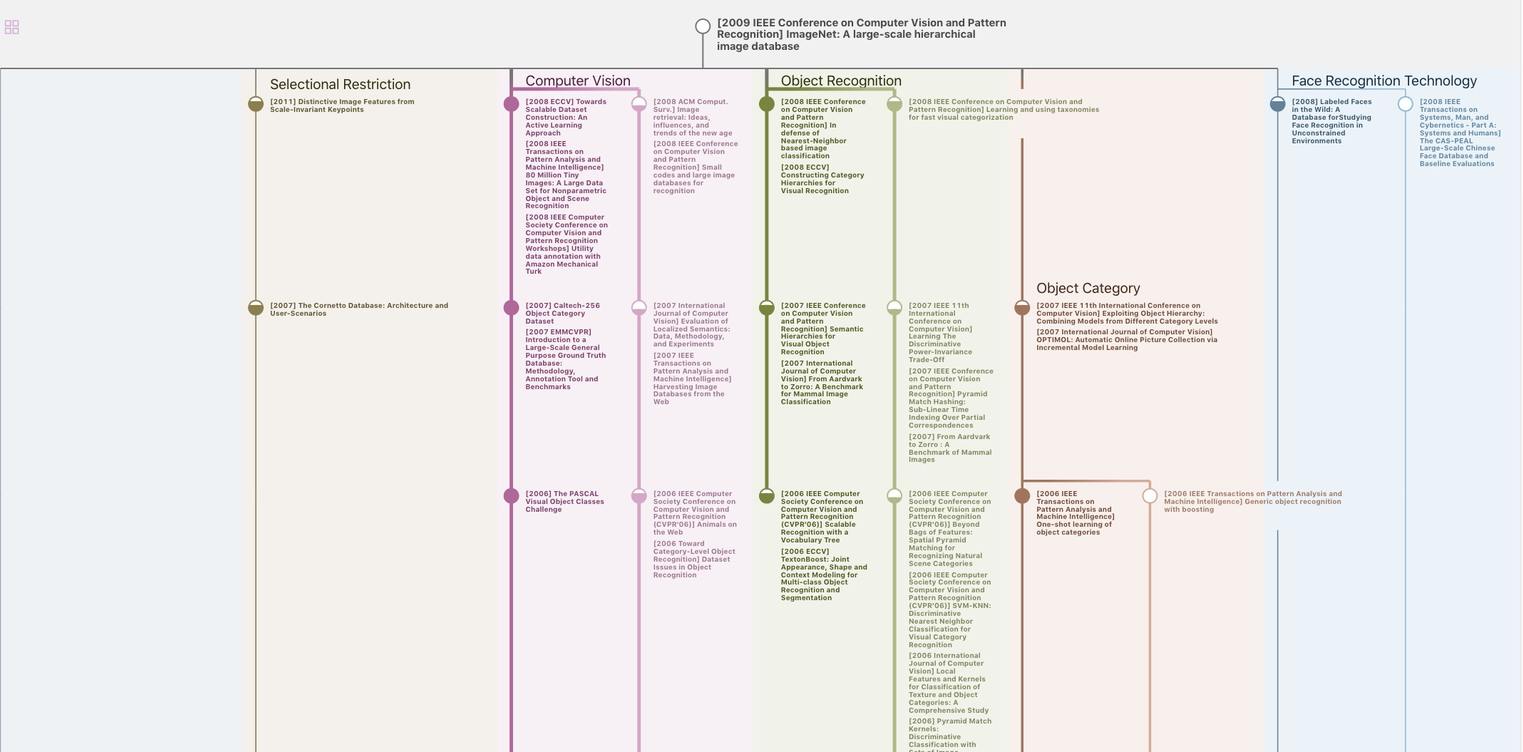
生成溯源树,研究论文发展脉络
Chat Paper
正在生成论文摘要