An automated method for mining high-quality assertion sets
MICROPROCESSORS AND MICROSYSTEMS(2023)
摘要
Assertion-Based Verification (ABV) is one of the promising ways of functional verification. The efficiency of ABV largely depends on the quality of the assertions in terms of how accurately they capture the consistency between implementation and specification. To this end, several assertion miners have been developed to automatically generate assertions. However, existing automatic assertion miners typically generate a huge amount of assertions which can lead to overhead in the verification process. Assertion evaluation, on the other hand, has recently appeared to evaluate and select high-quality assertions among the huge generated assertion set. These methods typically measure the quality of an assertion based on different metrics. These metrics nonetheless, consider dissimilar and distinct aspects which lead to difficulties in deciding what metric should influence more in assertion evaluation. Thereby, to exceed the state-of-the-art, a flow is proposed in which an assertion miner and an assertion evaluator are introduced. The assertion miner is capable of generating a set of readable and compact assertions. The assertion evaluator instead estimates the quality of the assertion set with a data-mining-based algorithm called dominance. Dominance is able to analyze the outcome of different metrics to unify them. Experimental results present the effectiveness of the proposed flow by comparing them to the state-of-the-art.
更多查看译文
关键词
Assertion-based verification,Automatic assertion mining,Assertion qualification,Data mining
AI 理解论文
溯源树
样例
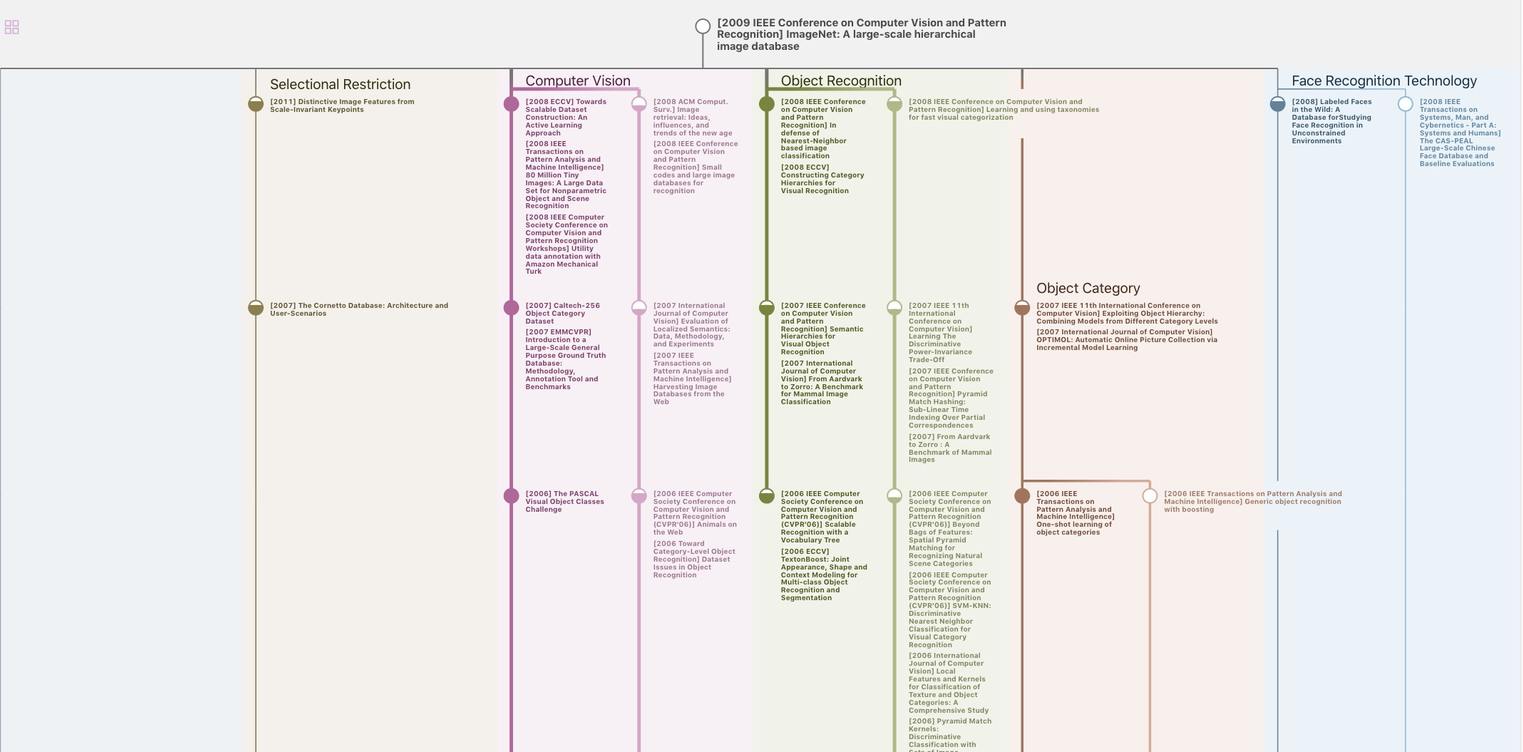
生成溯源树,研究论文发展脉络
Chat Paper
正在生成论文摘要