GHQ: Grouped Hybrid Q Learning for Heterogeneous Cooperative Multi-agent Reinforcement Learning
arxiv(2023)
摘要
Previous deep multi-agent reinforcement learning (MARL) algorithms have achieved impressive results, typically in homogeneous scenarios. However, heterogeneous scenarios are also very common and usually harder to solve. In this paper, we mainly discuss cooperative heterogeneous MARL problems in Starcraft Multi-Agent Challenges (SMAC) environment. We firstly define and describe the heterogeneous problems in SMAC. In order to comprehensively reveal and study the problem, we make new maps added to the original SMAC maps. We find that baseline algorithms fail to perform well in those heterogeneous maps. To address this issue, we propose the Grouped Individual-Global-Max Consistency (GIGM) and a novel MARL algorithm, Grouped Hybrid Q Learning (GHQ). GHQ separates agents into several groups and keeps individual parameters for each group, along with a novel hybrid structure for factorization. To enhance coordination between groups, we maximize the Inter-group Mutual Information (IGMI) between groups' trajectories. Experiments on original and new heterogeneous maps show the fabulous performance of GHQ compared to other state-of-the-art algorithms.
更多查看译文
关键词
multi-agent
multi-agent,learning
AI 理解论文
溯源树
样例
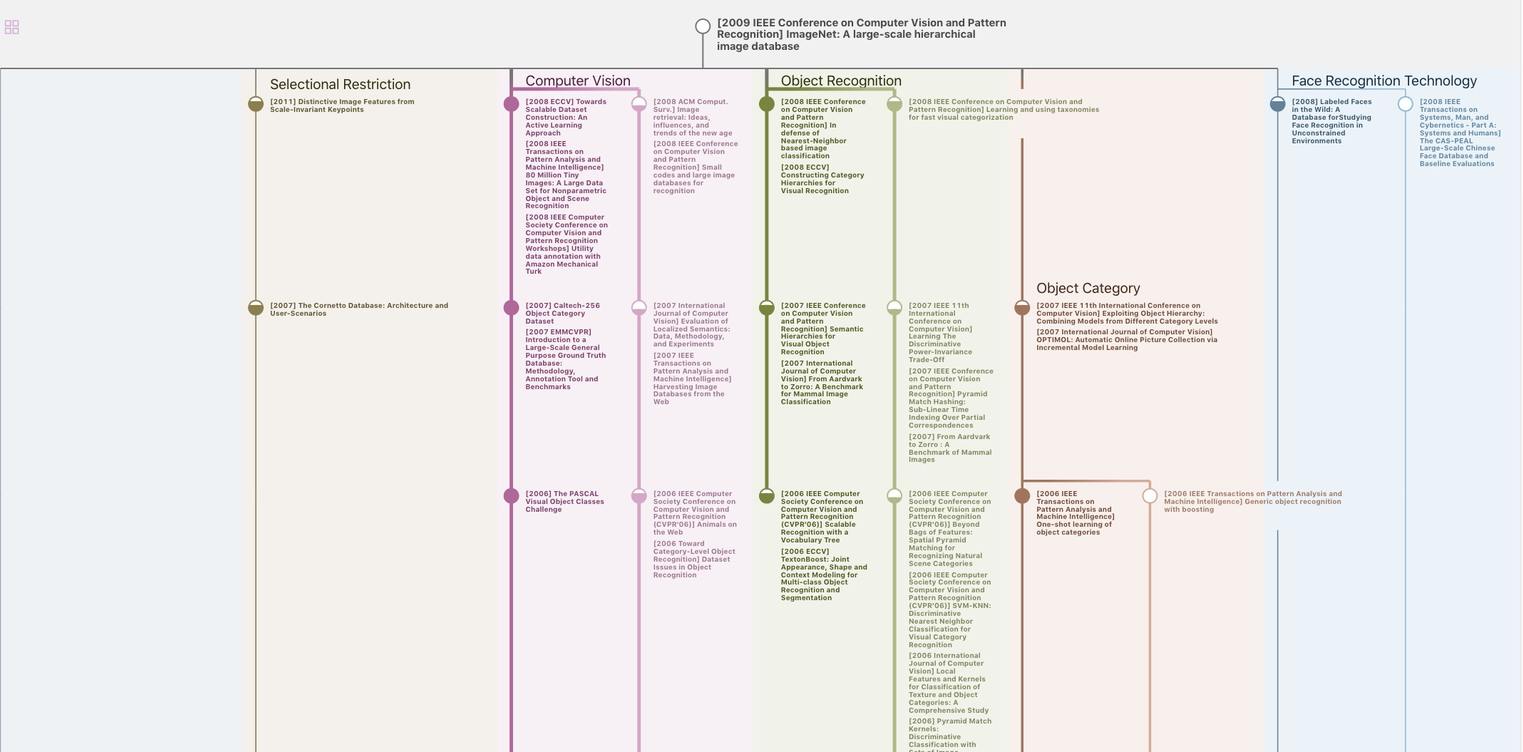
生成溯源树,研究论文发展脉络
Chat Paper
正在生成论文摘要