Building High-accuracy Multilingual ASR with Gated Language Experts and Curriculum Training
arxiv(2023)
摘要
We propose gated language experts to improve multilingual transformer transducer models without any language identification (LID) input from users during inference. We define gating mechanism and LID loss to let transformer encoders learn language-dependent information, construct the multilingual transformer block with gated transformer experts and shared transformer layers for compact models, and apply linear experts on joint network output to better regularize speech acoustic and token label joint information. Furthermore, a curriculum training scheme is proposed to let LID guide the gated language experts for better serving their corresponding languages. Evaluated on the English and Spanish bilingual task, our methods achieve average 12.5% and 7.3% relative word error reductions over the baseline bilingual model and monolingual models, respectively, obtaining similar results to the upper bound model trained and inferred with oracle LID. We further explore our method on trilingual, quadrilingual, and pentalingual models, and observe similar advantages as in the bilingual models, which demonstrates the easy extension to more languages.
更多查看译文
关键词
Multilingual automatic speech recognition,transformer transducer,language ID,expert
AI 理解论文
溯源树
样例
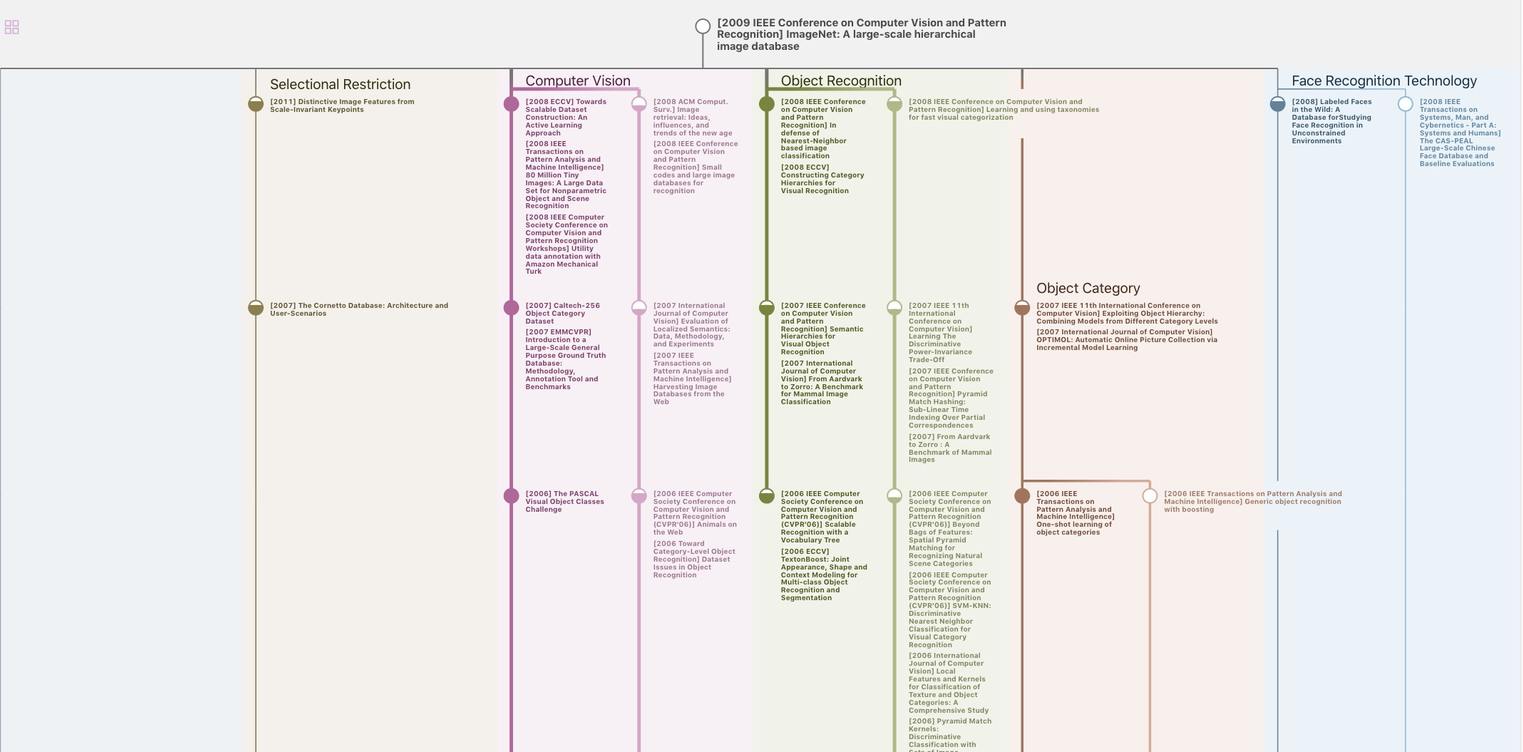
生成溯源树,研究论文发展脉络
Chat Paper
正在生成论文摘要