A Deep Neural Architecture for Harmonizing 3-D Input Data Analysis and Decision Making in Medical Imaging
arxiv(2023)
摘要
Harmonizing the analysis of data, especially of 3-D image volumes, consisting of different number of slices and annotated per volume, is a significant problem in training and using deep neural networks in various applications, including medical imaging. Moreover, unifying the decision making of the networks over different input datasets is crucial for the generation of rich data-driven knowledge and for trusted usage in the applications. This paper presents a new deep neural architecture, named RACNet, which includes routing and feature alignment steps and effectively handles different input lengths and single annotations of the 3-D image inputs, whilst providing highly accurate decisions. In addition, through latent variable extraction from the trained RACNet, a set of anchors are generated providing further insight on the network's decision making. These can be used to enrich and unify data-driven knowledge extracted from different datasets. An extensive experimental study illustrates the above developments, focusing on COVID-19 diagnosis through analysis of 3-D chest CT scans from databases generated in different countries and medical centers.
更多查看译文
关键词
deep neural architecture,imaging,data,harmonizing,input
AI 理解论文
溯源树
样例
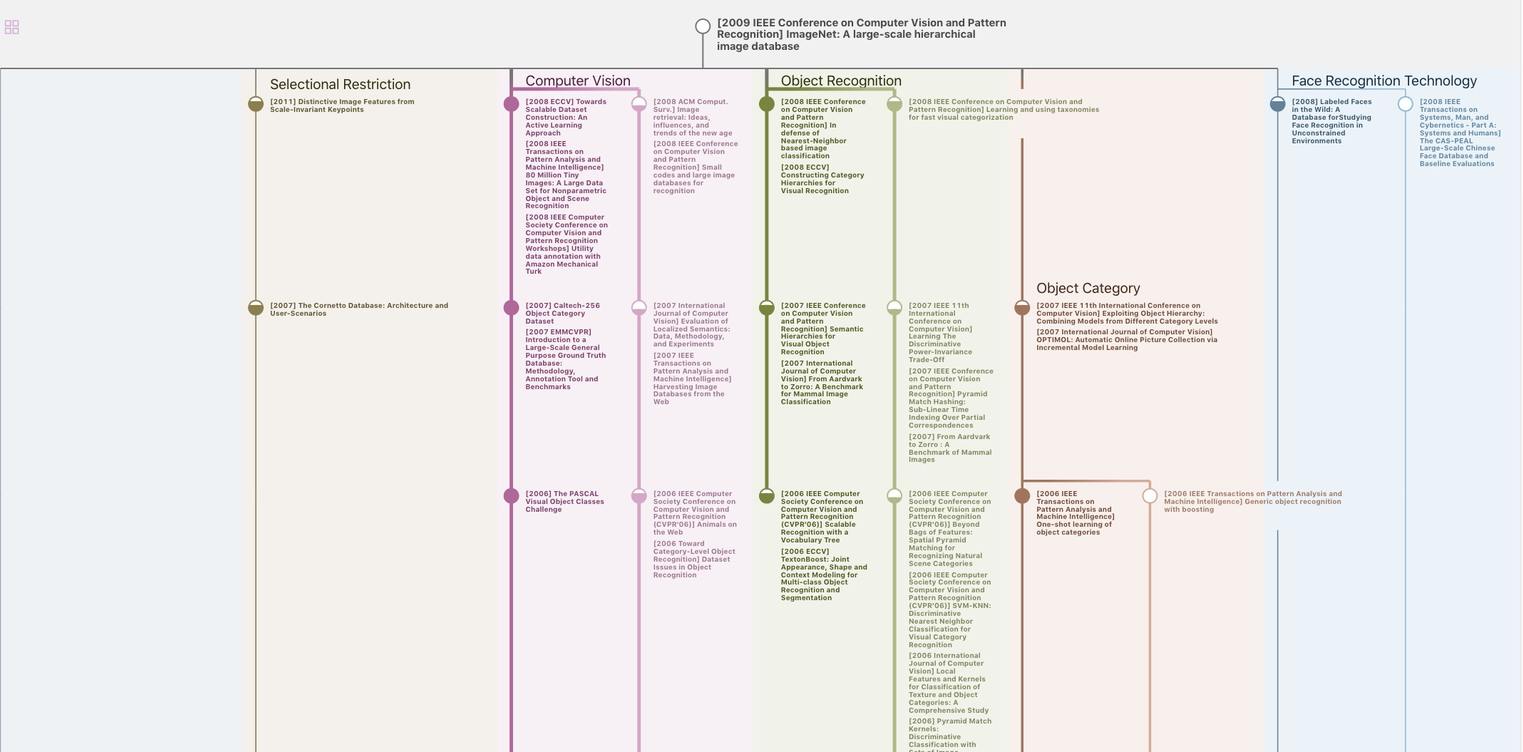
生成溯源树,研究论文发展脉络
Chat Paper
正在生成论文摘要