Benchmarks and Explanations for Deep Learning Estimates of X-ray Galaxy Cluster Masses
arxiv(2023)
摘要
We evaluate the effectiveness of deep learning (DL) models for reconstructing the masses of galaxy clusters using X-ray photometry data from next-generation surveys. We establish these constraints using a catalog of realistic mock eROSITA X-ray observations which use hydrodynamical simulations to model realistic cluster morphology, background emission, telescope response, and AGN sources. Using bolometric X-ray photon maps as input, DL models achieve a predictive mass scatter of $\sigma_{\ln M_\mathrm{500c}} = 18.3\%$, a factor of two improvements on scalar observables such as richness $N_\mathrm{gal}$, 1D velocity dispersion $\sigma_\mathrm{v,1D}$, and photon count $N_\mathrm{phot}$ as well as a $31\%$ improvement upon idealized, volume-integrated measurements of the bolometric X-ray luminosity $L_X$. We then show that extending this model to handle multichannel X-ray photon maps, separated in low, medium, and high energy bands, further reduces the mass scatter to $16.4\%$. We also tested a multimodal DL model incorporating both dynamical and X-ray cluster probes and achieved marginal gains at a mass scatter of $16.2\%$. Finally, we conduct a quantitative interpretability study of our DL models and find that they greatly down-weight the importance of pixels in the centers of clusters and at the location of AGN sources, validating previous claims of DL modeling improvements and suggesting practical and theoretical benefits for using DL in X-ray mass inference.
更多查看译文
关键词
deep learning estimates,cluster,x-ray
AI 理解论文
溯源树
样例
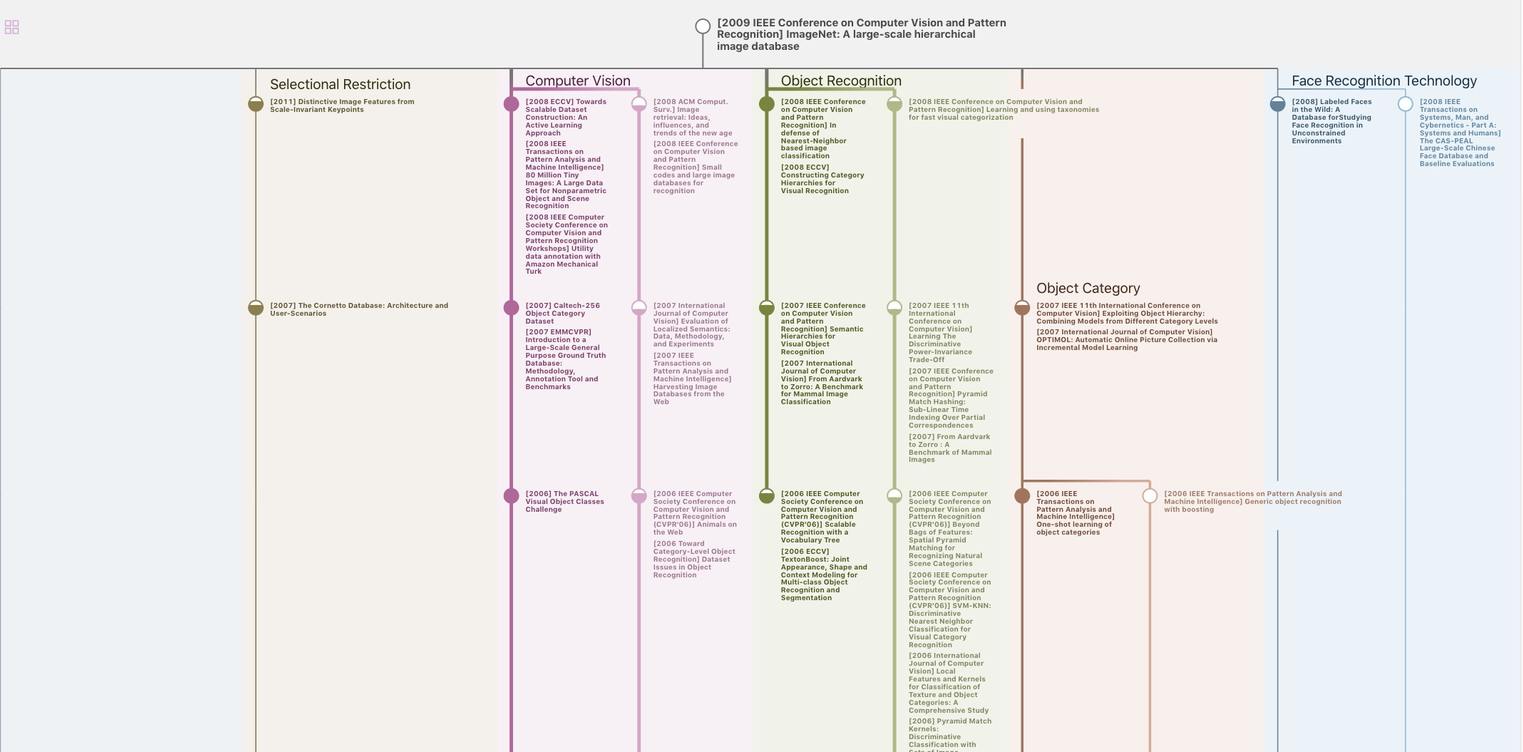
生成溯源树,研究论文发展脉络
Chat Paper
正在生成论文摘要