Snapshot Ensemble One-Dimensional Convolutional Neural Networks for Ballistic Target Recognition
2023 6th World Conference on Computing and Communication Technologies (WCCCT)(2023)
摘要
Aiming at improving the performance of ballistic target HRRP recognition, a ballistic target HRRP recognition method based on snapshot ensemble one-dimensional convolutional neural network (SSE-1DCNN) is proposed. The snapshot ensemble model is constructed by integrating a single 1DCNN estimator with a cosine annealing scheduler. The snapshot ensemble integrates 1DCNN estimators at different loss function minima in the same training process, which ensures the diversity of training estimators and avoids the increase in training cost for estimator ensemble. In addition, the AdamW algorithm is introduced to improve the convergence speed and degree of the ensemble training. The experimental results show that the use of snapshot ensemble can effectively improve the recognition of ballistic target HRRP by the 1DCNN, and the introduction of AdamW effectively improves the convergence effect. Compared with a single 1DCNN estimator, the optimal average recognition accuracy of SSE-1DCNN is improved by 0.31% withinside the hyperparameter experimental setting ranges.
更多查看译文
关键词
ballistic missiles,target recognition,high-resolution range profile,convolutional neural networks,snapshot ensemble,stochastic gradient descent
AI 理解论文
溯源树
样例
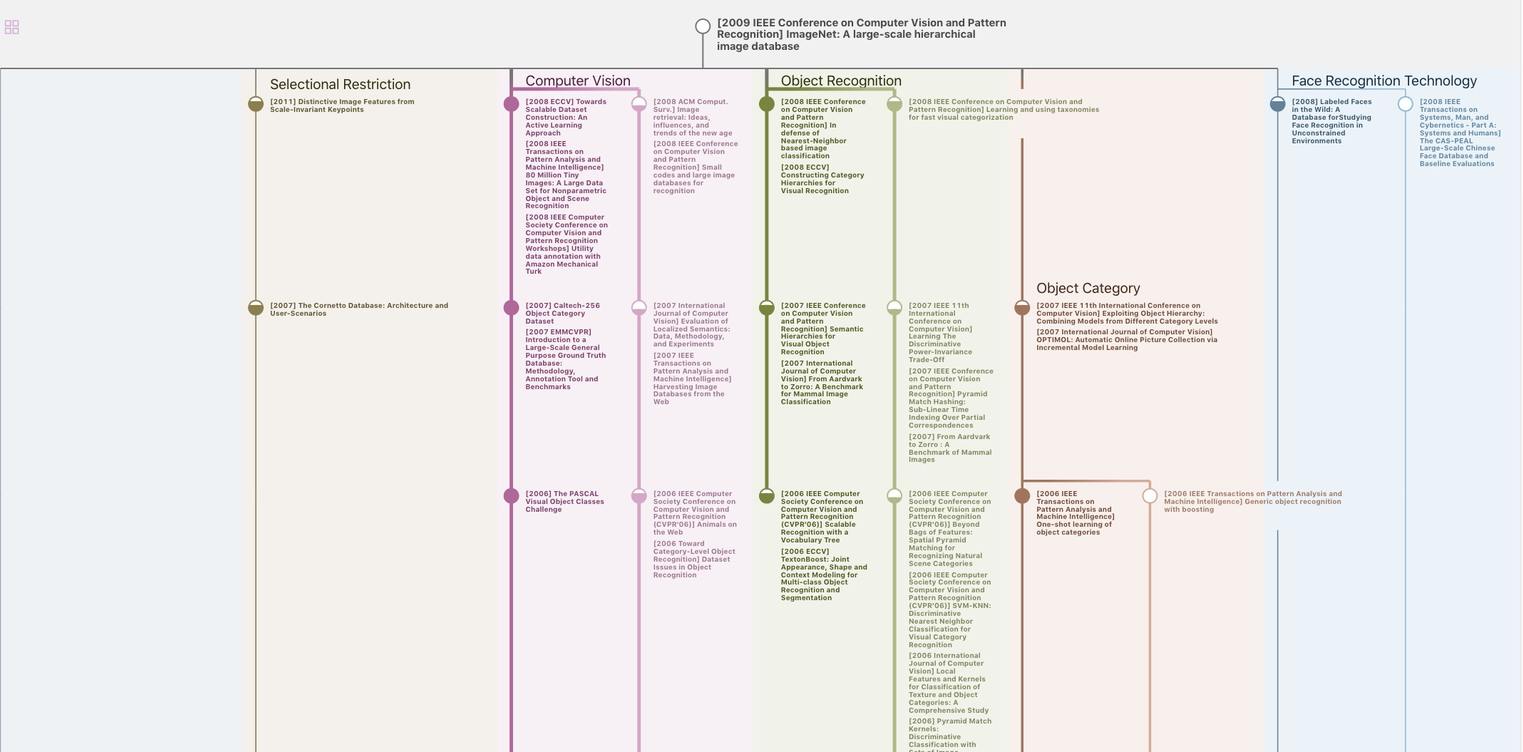
生成溯源树,研究论文发展脉络
Chat Paper
正在生成论文摘要